Automated speech detection in eco-acoustic data enables privacy protection and human disturbance quantification
biorxiv(2022)
摘要
Eco-acoustic monitoring is increasingly being used to map biodiversity across huge scales, yet little thought is given to the privacy concerns and potential scientific value of inadvertently recorded human speech. Automated speech detection is possible using Voice Activity Detection (VAD) models, but existing approaches have been developed for indoor or urban use cases, rather than diverse natural soundscapes. In this study we used a data augmentation approach to create ecoVAD, a convolutional neural network designed for robust voice detection in eco-acoustic data. We performed playback experiments using speech samples from a woman, man, and child in two ecosystems in Børsa, Norway, and showed that ecoVAD was able to accurately detect voices at distances of up to 20m – at which point the speech was unintelligible. We compared ecoVAD with two existing VAD models and found that ecoVAD consistently outperformed the state-of-the-art (mean F1 scores: ecoVAD, 0.917; pyannote, 0.890; WebRTC VAD, 0.876). Using long-term passive recordings from a popular hiking location in Bymarka, Norway, we found that the frequency of speech detections was linked closely to peak traffic hours (using bus timings) demonstrating how VAD models can be used to quantify human activity with a fine temporal resolution. Anonymising audio data effectively using VAD models will allow eco-acoustic monitoring to continue to deliver invaluable ecological insight at scale, whilst minimising the risk of data misuse. Furthermore, using speech detections as a fine scale measure of human disturbance opens new possibilities for studying subtle human-wildlife interactions on the vast scales made possible by eco-acoustic monitoring technology.
### Competing Interest Statement
The authors have declared no competing interest.
更多查看译文
AI 理解论文
溯源树
样例
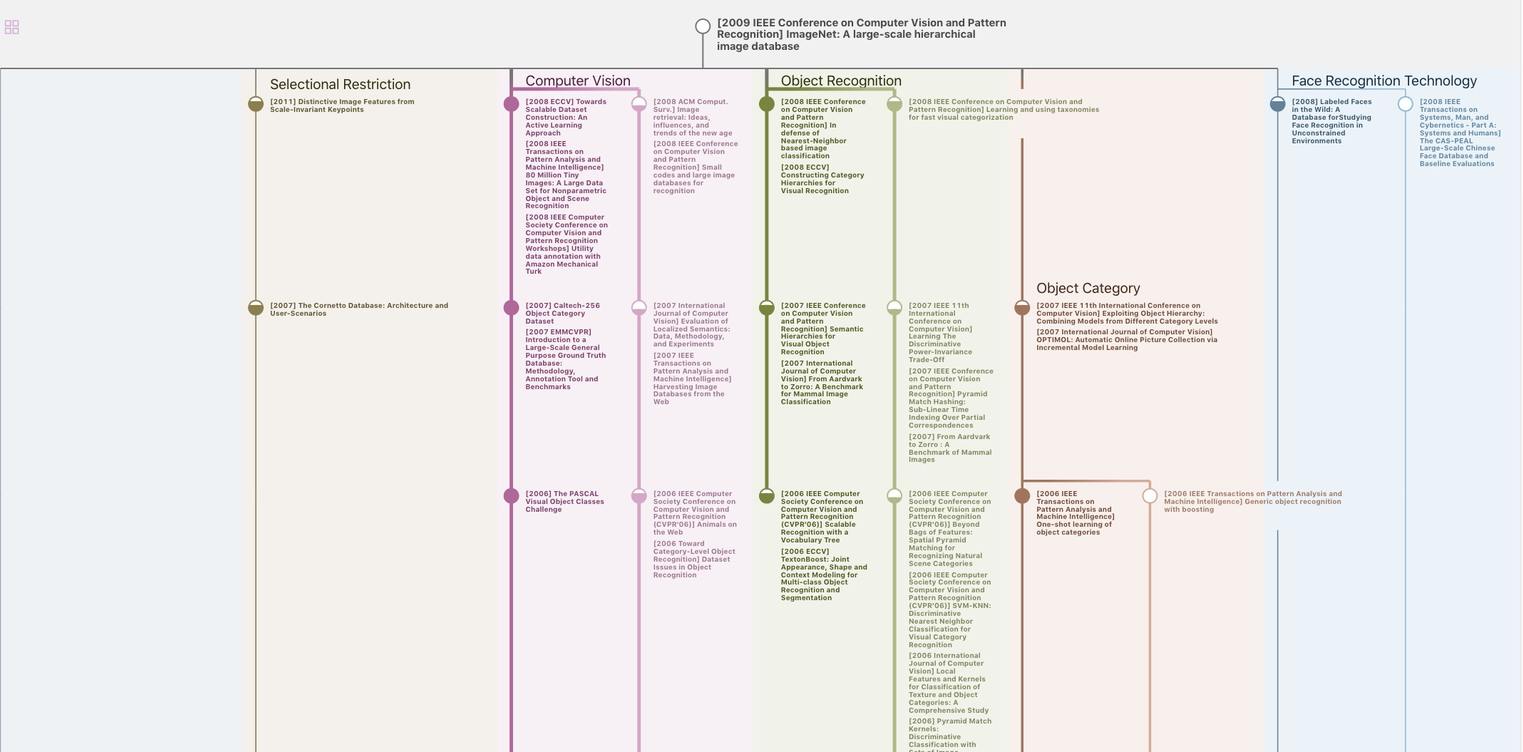
生成溯源树,研究论文发展脉络
Chat Paper
正在生成论文摘要