Differential Private Knowledge Transfer for Privacy-Preserving Cross-Domain Recommendation
International World Wide Web Conference(2022)
摘要
ABSTRACT Cross Domain Recommendation (CDR) has been popularly studied to alleviate the cold-start and data sparsity problem commonly existed in recommender systems. CDR models can improve the recommendation performance of a target domain by leveraging the data of other source domains. However, most existing CDR models assume information can directly ‘transfer across the bridge’, ignoring the privacy issues. To solve this problem, we propose a novel two stage based privacy-preserving CDR framework (PriCDR). In the first stage, we propose two methods, i.e., Johnson-Lindenstrauss Transform (JLT) and Sparse-aware JLT (SJLT), to publish the rating matrix of the source domain using Differential Privacy (DP). We theoretically analyze the privacy and utility of our proposed DP based rating publishing methods. In the second stage, we propose a novel heterogeneous CDR model (HeteroCDR), which uses deep auto-encoder and deep neural network to model the published source rating matrix and target rating matrix respectively. To this end, PriCDR can not only protect the data privacy of the source domain, but also alleviate the data sparsity of the source domain. We conduct experiments on two benchmark datasets and the results demonstrate the effectiveness of PriCDR and HeteroCDR.
更多查看译文
关键词
Cross-domain recommendation, Differential privacy, Privacy-preserving
AI 理解论文
溯源树
样例
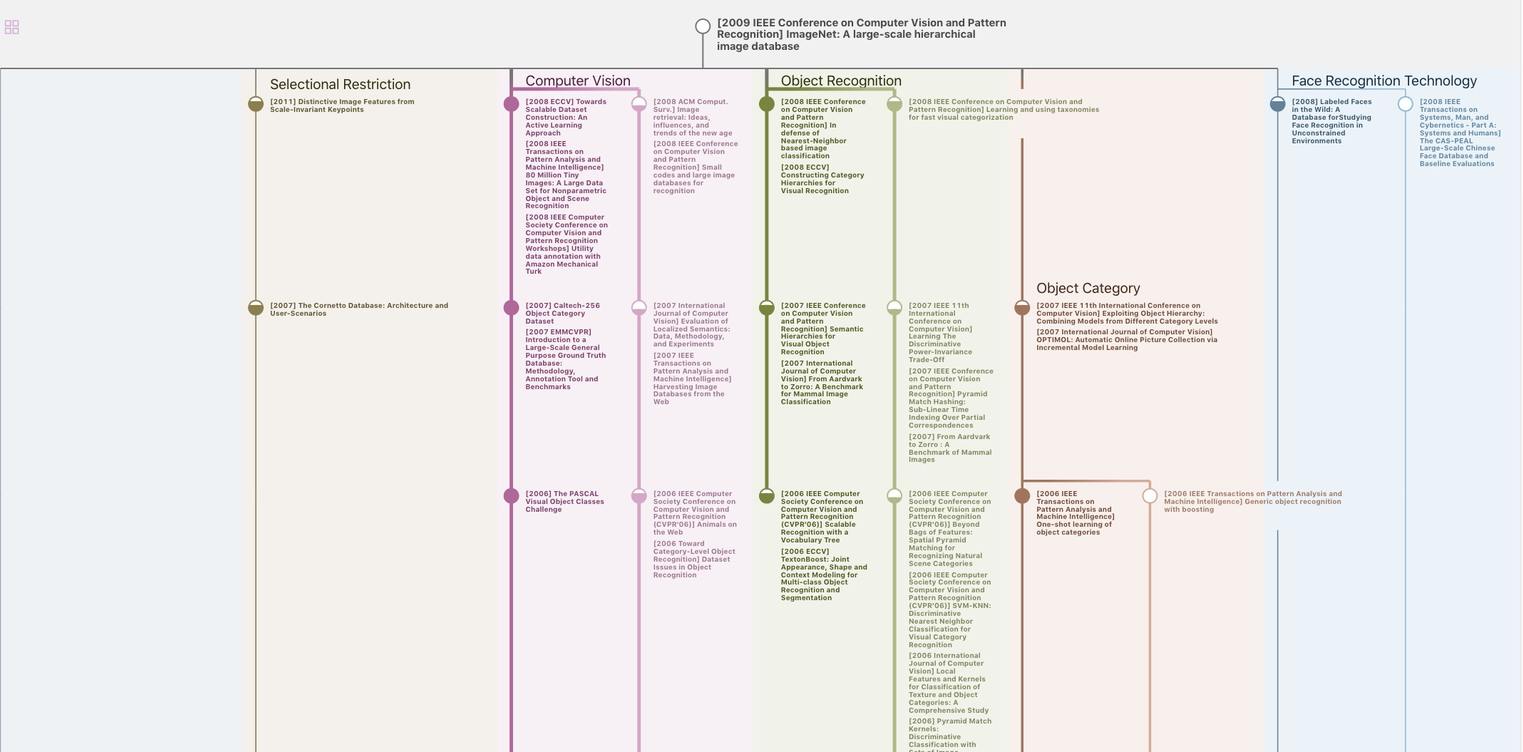
生成溯源树,研究论文发展脉络
Chat Paper
正在生成论文摘要