Ultra-short-term power forecast method for the wind farm based on feature selection and temporal convolution network
ISA Transactions(2022)
摘要
The random fluctuation of wind energy is so strong that the output power cannot be predicted in time and accurately, which will influence the safety and stability of the power system. By analyzing the output power and meteorological data, the ultra-short-term power forecast method of the wind farm has been studied in this paper. Firstly, all the feature data are preprocessed and part of them with stronger correlation with the output power are obtained according to the eXtreme Gradient Boosting (XGBoost) algorithm. Then, with the reconstructed datasets and the Tree-structured Parzen Estimator (TPE) algorithm, the optimal temporal convolution network (TCN) is achieved to forecast the output power. Finally, with respect to a certain wind farm in China, ablation study and comparative experiments are conducted respectively. The ablation experiment results show that by adding the feature selection procedure into all the models, the indicators RMSE and MAE are obviously reduced as well as the running time of the model. Among them, our proposed method based on XGBoost and TCN performs best, which provides a new prospect for investigating the ultra-short-term wind power forecast problem.
更多查看译文
关键词
Ultra-short-term wind power forecast,XGBoost,TPE,Deep learning,TCN
AI 理解论文
溯源树
样例
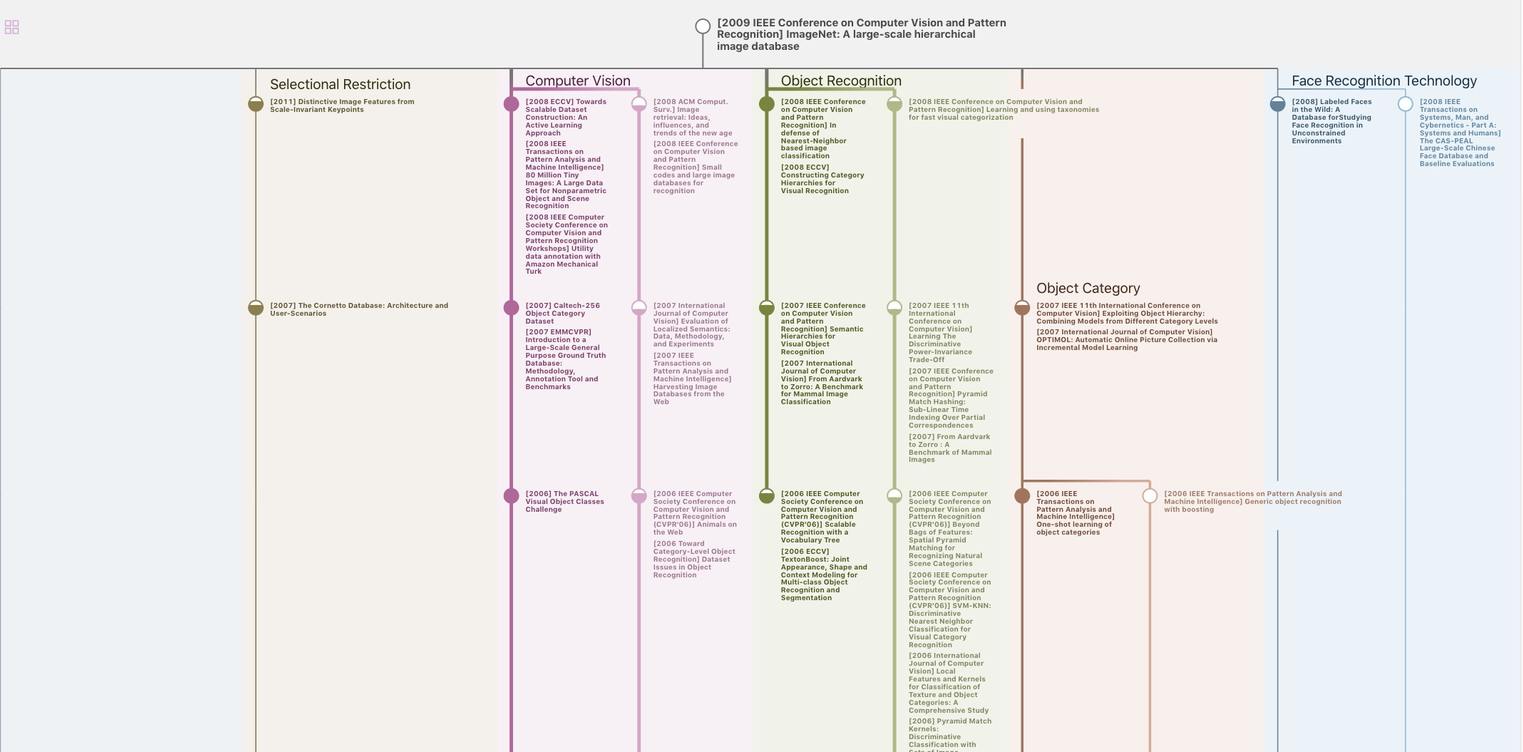
生成溯源树,研究论文发展脉络
Chat Paper
正在生成论文摘要