Empirical Risk Minimization with Relative Entropy Regularization: Optimality and Sensitivity Analysis.
International Symposium on Information Theory (ISIT)(2022)
摘要
The optimality and sensitivity of the empirical risk minimization problem with relative entropy regularization (ERM-RER) are investigated for the case in which the reference is a sigma-finite measure instead of a probability measure. This generalization allows for a larger degree of flexibility in the incorporation of prior knowledge over the set of models. In this setting, the interplay of the regularization parameter, the reference measure, the risk function, and the empirical risk induced by the solution of the ERM-RER problem is characterized. This characterization yields necessary and sufficient conditions for the existence of a regularization parameter that achieves an arbitrarily small empirical risk with arbitrarily high probability. The sensitivity of the expected empirical risk to deviations from the solution of the ERM-RER problem is studied. The sensitivity is then used to provide upper and lower bounds on the expected empirical risk. Moreover, it is shown that the expectation of the sensitivity is upper bounded, up to a constant factor, by the square root of the lautum information between the models and the datasets.
更多查看译文
关键词
empirical risk minimization,relative entropy regularization
AI 理解论文
溯源树
样例
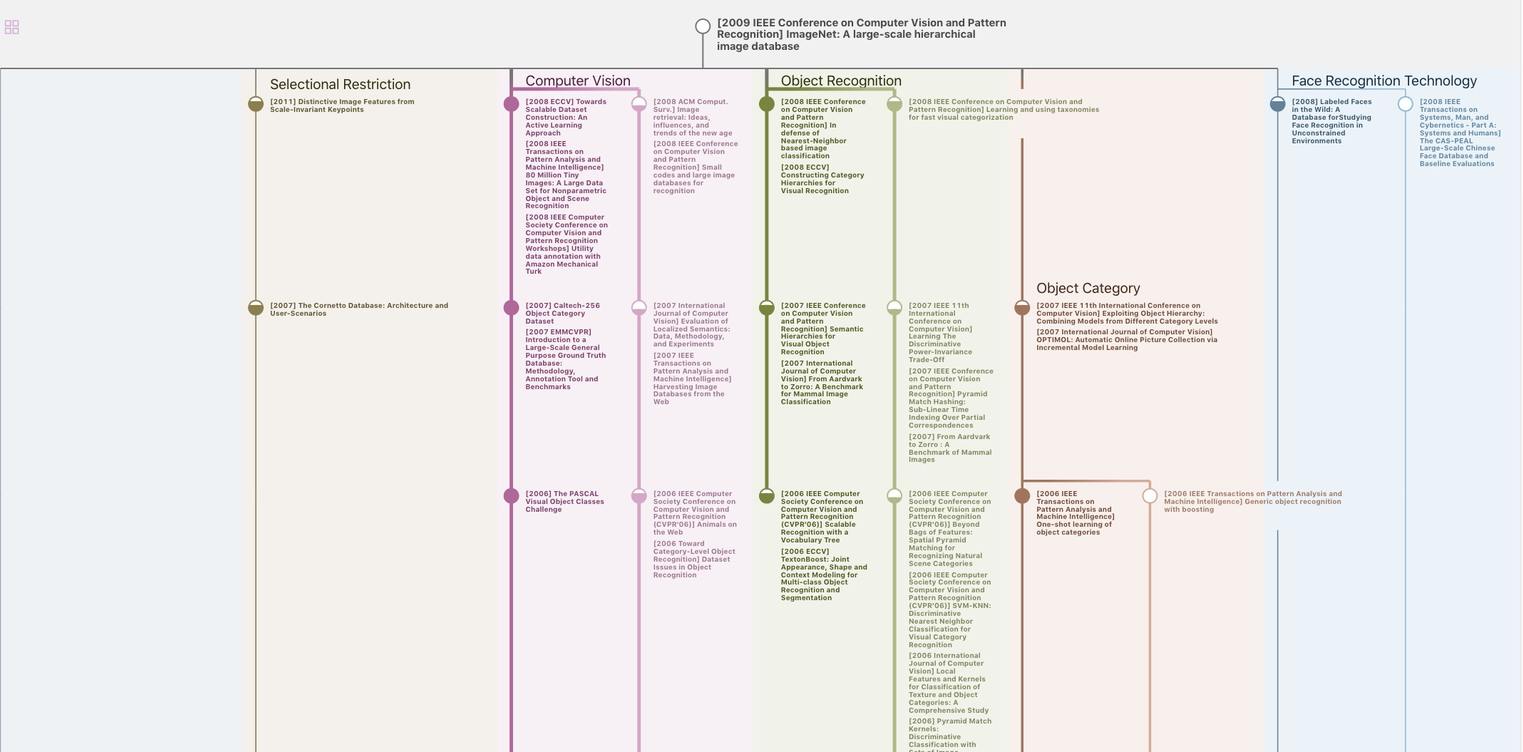
生成溯源树,研究论文发展脉络
Chat Paper
正在生成论文摘要