Predictive Beamforming for Integrated Sensing and Communication in Vehicular Networks: A Deep Learning Approach
IEEE International Conference on Communications (ICC)(2022)
摘要
The implementation of integrated sensing and communication (ISAC) highly depends on the effective beamforming design exploiting accurate instantaneous channel state information (ICSI). However, channel tracking in ISAC requires large amount of training overhead and prohibitively large computational complexity. To address this problem, in this paper, we focus on ISAC-assisted vehicular networks and exploit a deep learning approach to implicitly learn the features of historical channels and directly predict the beamforming matrix for the next time slot to maximize the average achievable sum-rate of system, thus bypassing the need of explicit channel tracking for reducing the system signaling overhead. To this end, a general sum-rate maximization problem with Cramer-Rao lower bounds-based sensing constraints is first formulated for the considered ISAC system. Then, a historical channels-based convolutional long short-term memory network is designed for predictive beamforming that can exploit the spatial and temporal dependencies of communication channels to further improve the learning performance. Finally, simulation results show that the proposed method can satisfy the requirement of sensing performance, while its achievable sum-rate can approach the upper bound obtained by a genie-aided scheme with perfect ICSI available.
更多查看译文
关键词
historical channels-based convolutional long short-term memory network,system signaling overhead,integrated sensing-and-communication,sensing performance,learning performance,communication channels,temporal dependencies,spatial dependencies,ISAC system,Cramer-Rao lower bounds-based sensing constraints,general sum-rate maximization problem,channel tracking,average achievable sum-rate,beamforming matrix,ISAC-assisted vehicular networks,computational complexity,training overhead,instantaneous channel state information,beamforming design,deep learning approach,predictive beamforming
AI 理解论文
溯源树
样例
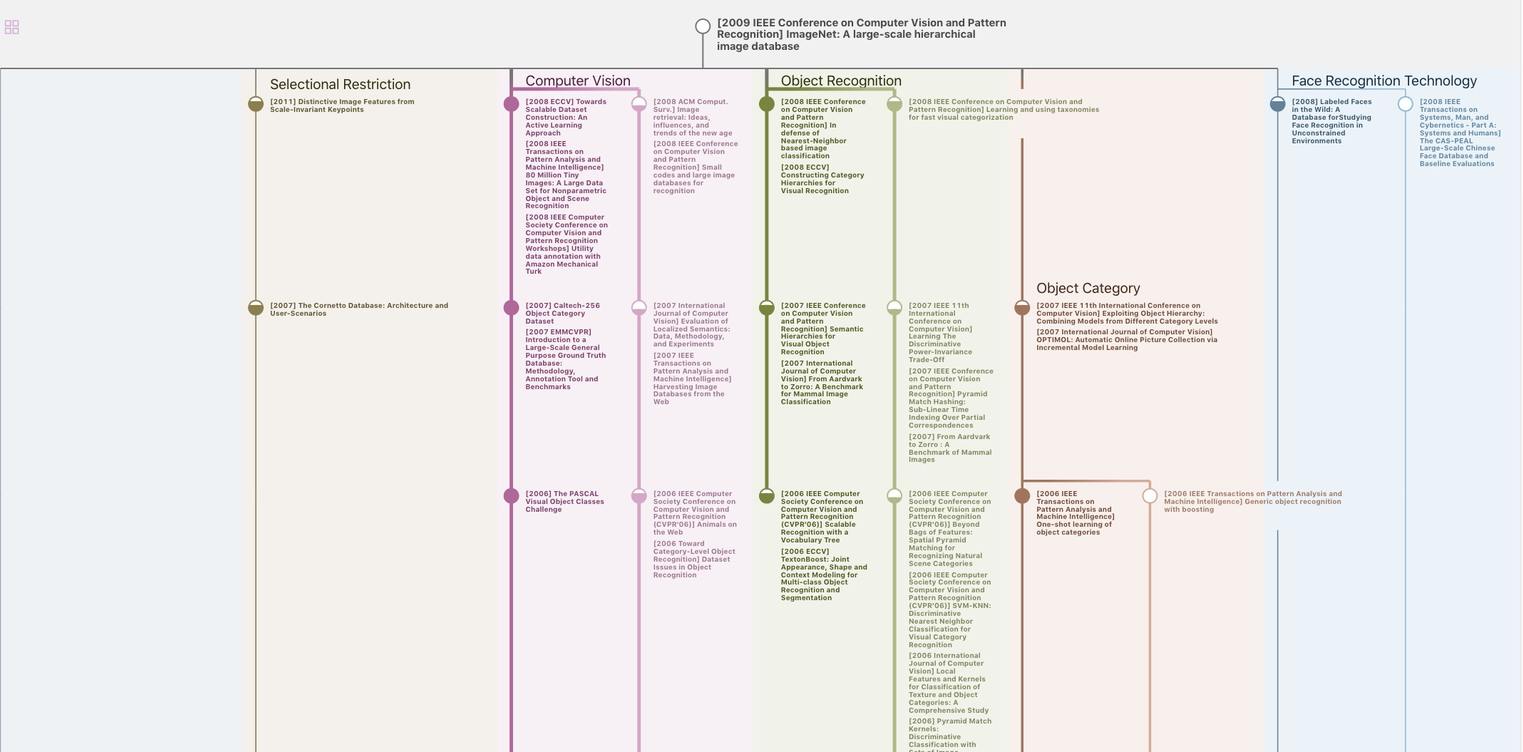
生成溯源树,研究论文发展脉络
Chat Paper
正在生成论文摘要