Online Intention Recognition With Incomplete Information Based on a Weighted Contrastive Predictive Coding Model in Wargame
IEEE transactions on neural networks and learning systems(2023)
摘要
The incomplete and imperfect essence of the battlefield situation results in a challenge to the efficiency, stability, and reliability of traditional intention recognition methods. For this problem, we propose a deep learning architecture that consists of a contrastive predictive coding (CPC) model, a variable-length long short-term memory network (LSTM) model, and an attention weight allocator for online intention recognition with incomplete information in wargame (W-CPCLSTM). First, based on the typical characteristics of intelligence data, a CPC model is designed to capture more global structures from limited battlefield information. Then, a variable-length LSTM model is employed to classify the learned representations into predefined intention categories. Next, a weighted approach to the training attention of CPC and LSTM is introduced to allow for the stability of the model. Finally, performance evaluation and application analysis of the proposed model for the online intention recognition task were carried out based on four different degrees of detection information and a perfect situation of ideal conditions in a wargame. Besides, we explored the effect of different lengths of intelligence data on recognition performance and gave application examples of the proposed model to a wargame platform. The simulation results demonstrate that our method not only contributes to the growth of recognition stability, but it also improves recognition accuracy by 7%–11%, 3%–7%, 3%–13%, and 3%–7%, the recognition speed by 6–
$32\times $
, 4–
$18\times $
, 13–*
$\times $
, and 1–
$6\times $
compared with the traditional LSTM, classical FCN, OctConv, and OctFCN models, respectively, which characterizes it as a promising reference tool for command decision-making.
更多查看译文
关键词
Target recognition,Feature extraction,Training,Task analysis,Neural networks,Data models,Atmospheric modeling,Attention weight allocator,contrastive predictive coding (CPC) model,incomplete information,online intention recognition,variable-length long short-term memory network (LSTM) model
AI 理解论文
溯源树
样例
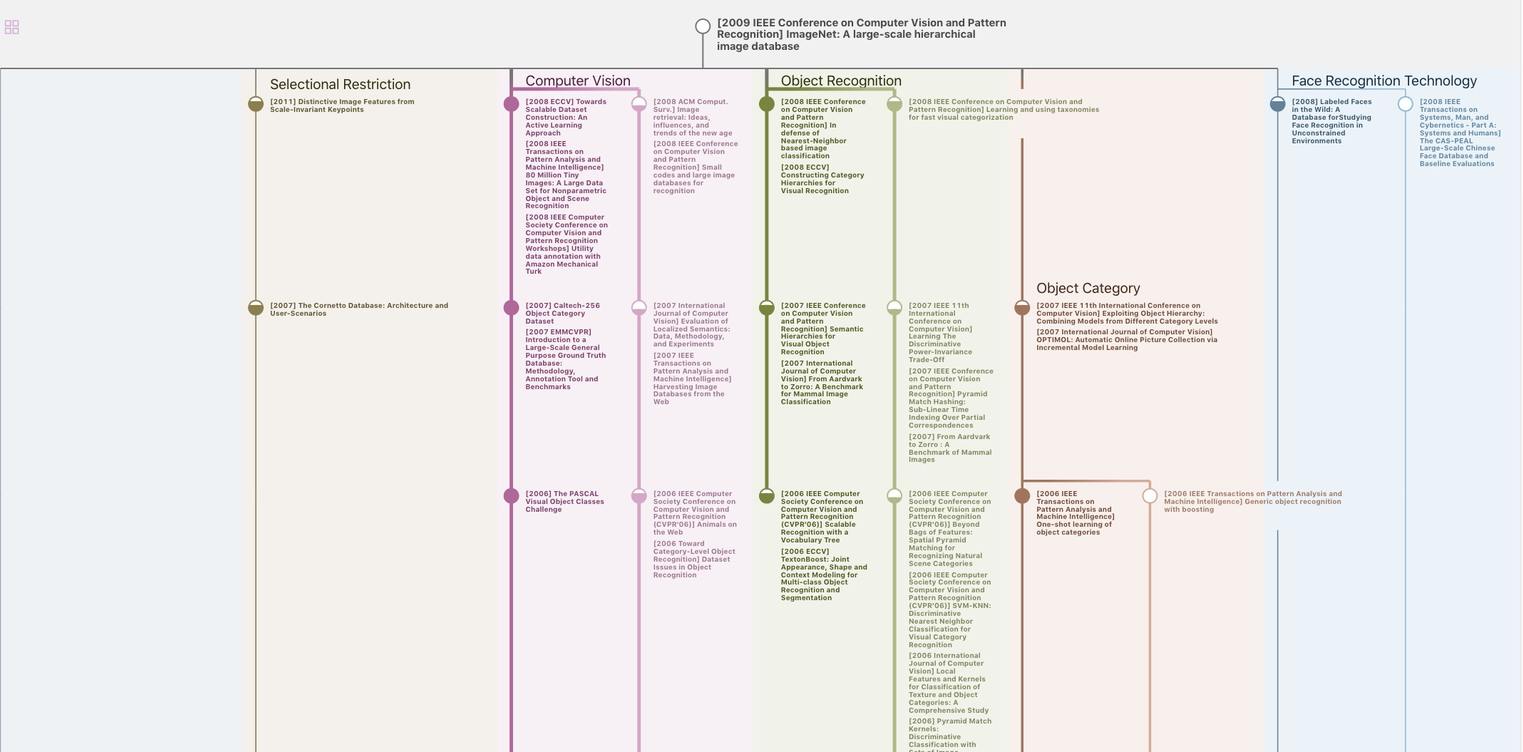
生成溯源树,研究论文发展脉络
Chat Paper
正在生成论文摘要