Data-Independent Acquisition Approach to Proteome: A Case Study and a Spectral Library for Mass Spectrometry-Based Investigation of Mycobacterium tuberculosis
OMICS-A JOURNAL OF INTEGRATIVE BIOLOGY(2022)
摘要
Currently, mass spectrometry-based data-dependent acquisition protocols require several micrograms to milligram amounts of proteins to start with, and needs fractionation and enrichment or depletion protocols to identify low abundant proteins and their modifications. However, a data-independent acquisition (DIA) approach can help us to identify a large number of proteins irrespective of their abundance, from even a very low amount of protein. In the DIA protocol, mass spectrometry data are matched against a previously established tandem mass spectrometry (MS/MS) spectra for each peptide. Therefore, establishing a spectral library is a prerequisite for successful DIA protocol. However, the DIA protocol becomes extremely important to investigate biological systems, where there is a difficulty in gathering reasonable amounts of proteins. In this context, DIA can become a valuable tool to investigate proteome dynamics of slow growing pathogen such as Mycobacterium tuberculosis that causes tuberculosis. We report here a case study of the DIA approach that is ideal for M. tuberculosis, which cannot be scaled up easily as it requires specific BSL3 laboratory facilities to be grown. We generated a spectral library for M. tuberculosis proteome using six publicly available proteomic data sets. The in-house M. tuberculosis proteome spectral library contains MS/MS spectra for peptides corresponding to 88% of proteins when compared with the M. tuberculosis H37Rv proteome. We believe that the public availability of the M. tuberculosis spectral library is an important step forward to facilitate the research community to adopt DIA approaches, for example, to investigate M. tuberculosis proteome with greater depth and efficiency.
更多查看译文
关键词
data-dependent acquisition, tuberculosis, proteomics, spectral library, global health, omics
AI 理解论文
溯源树
样例
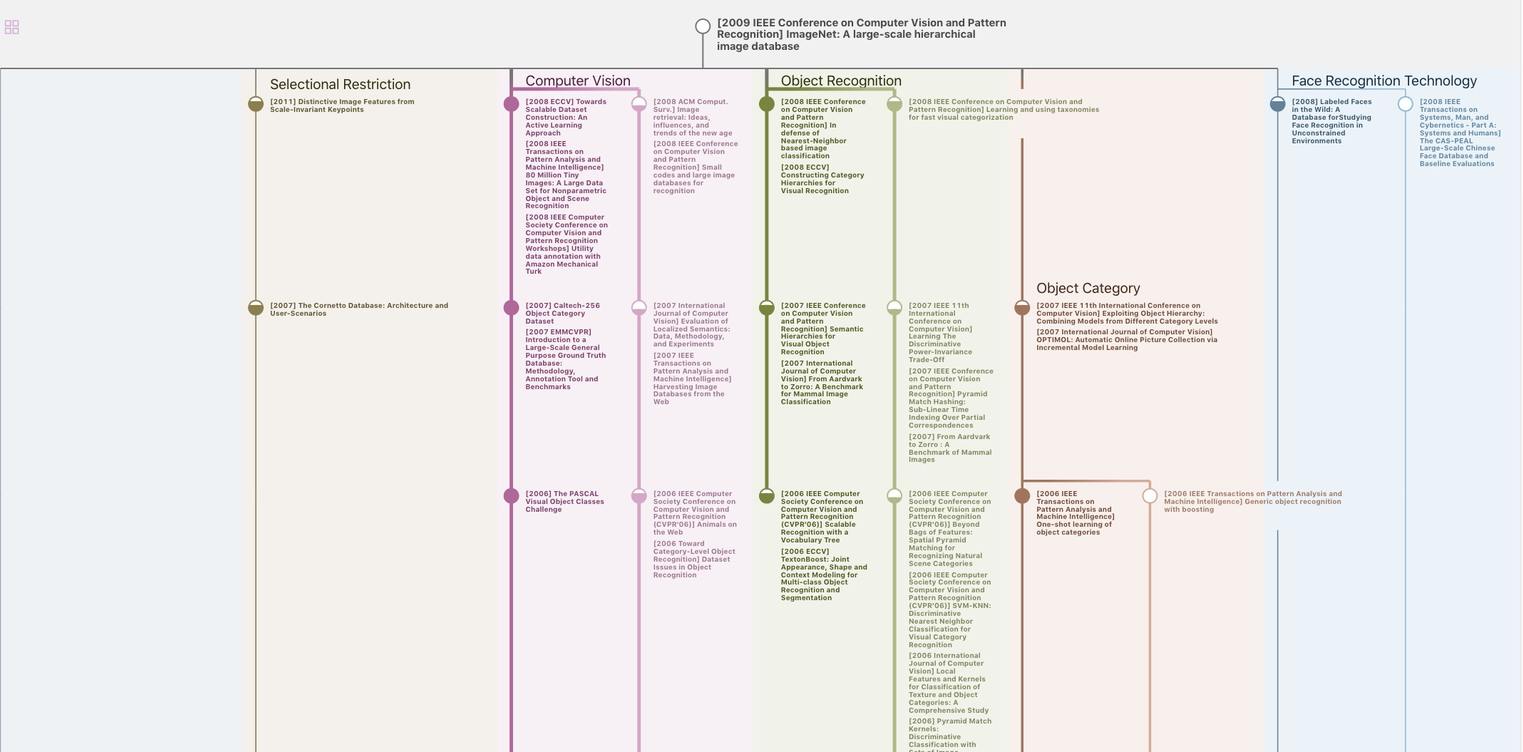
生成溯源树,研究论文发展脉络
Chat Paper
正在生成论文摘要