Performance Change with the Ratio of Training Data A Case Study on the Binary Classification of COVID-19 Chest X-Ray by using Convolutional Neural Networks.
COMPUTERS IN BIOLOGY AND MEDICINE(2023)
摘要
One of the features of artificial intelligence/machine learning-based medical devices resides in their ability to learn from real-world data. The performance may change after the market introduction. There are many aspects that contribute to the performance change relative to the real-world training data, such as the number and disease ratio. In actual clinical practice, the ratio of obtained training data varies from country to country, from region to region within each country, and from one hospital to another. Therefore, establishing a pre-change control plan at a premarket stage is essential to achieve safety and effectiveness through total product life cycles. In our previous work, we evaluated the performance change on the binary classification of coronavirus disease 2019 (COVID-19) and normal with the number of training data using two publicly large available chest X-ray (CXR) images. However, these results were obtained with the same ratio in the training data. Thus, this study aims to evaluate the performance change with a non-uniform ratio of COVID-19 CXR images based on the results of previous studies. We used the AlexNet and ResNet34 with and without the fine-tuning method as convolutional neural network (CNN) models. A total of 500, 1000, and 2000 CXR images were selected as training and validation datasets. These datasets represent states in which the performance change improves rapidly and those in which an equilibrium state is reached. Each dataset was divided into seven datasets, and the area under the curve was employed to evaluate the performance change for each dataset through independent 1000 test datasets with the same ratio. Our result shows that all performances indicate that there is an upward convex relationship to the ratio of COVID-19 CXR images, and the vertex is where the ratio is the same. This trend was remarkable for the rapidly improving state and the CNNs without a fine-tuning method. Moreover, the visual explanations technique called Grad-CAM for interpreting classification results of CNN models support these results.
更多查看译文
关键词
Performance change, Deep learning, Convolutional neural network, COVID-19, Chest X-ray, Medical device regulation
AI 理解论文
溯源树
样例
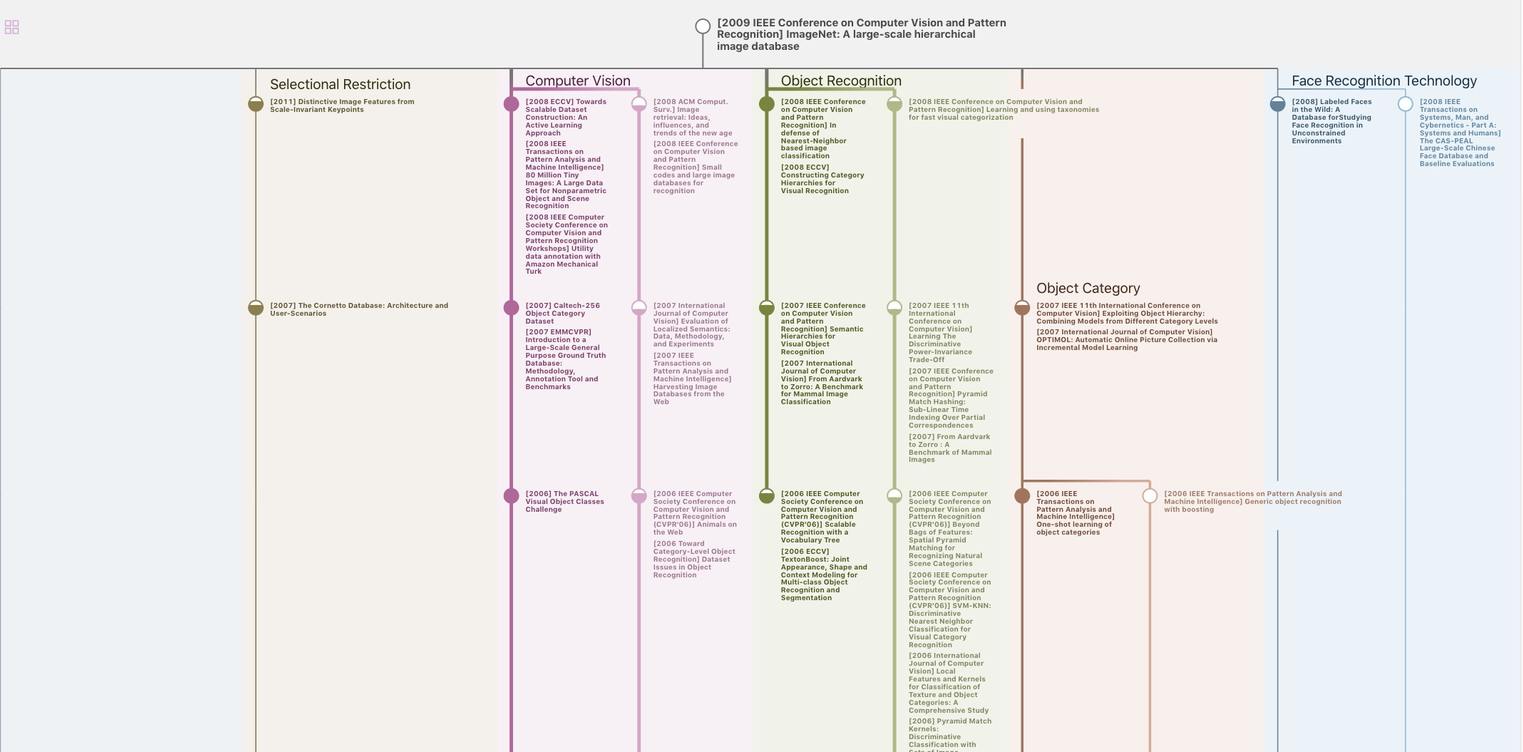
生成溯源树,研究论文发展脉络
Chat Paper
正在生成论文摘要