Won't you see my neighbor?: User predictions, mental models, and similarity-based explanations of AI classifiers
arxiv(2022)
摘要
Humans should be able work more effectively with artificial intelligence-based systems when they can predict likely failures and form useful mental models of how the systems work. We conducted a study of human's mental models of artificial intelligence systems using a high-performing image classifier, focusing on participants' ability to predict the classification result for a particular image. Participants viewed individual labeled images in one of two classes and then tried to predict whether the classifier would label them correctly. In this experiment we explored the effect of giving participants additional information about an image's nearest neighbors in a space representing the otherwise uninterpretable features extracted by the lower layers of the classifier's neural network. We found that providing this information did increase participants' prediction performance, and that the performance improvement could be related to the neighbor images' similarity to the target image. We also found indications that the presentation of this information may influence people's own classification of the target image -- that is, rather than just anthropomorphizing the system, in some cases the humans become "mechanomorphized" in their judgements.
更多查看译文
关键词
user predictions,mental models,ai,explanations,similarity-based
AI 理解论文
溯源树
样例
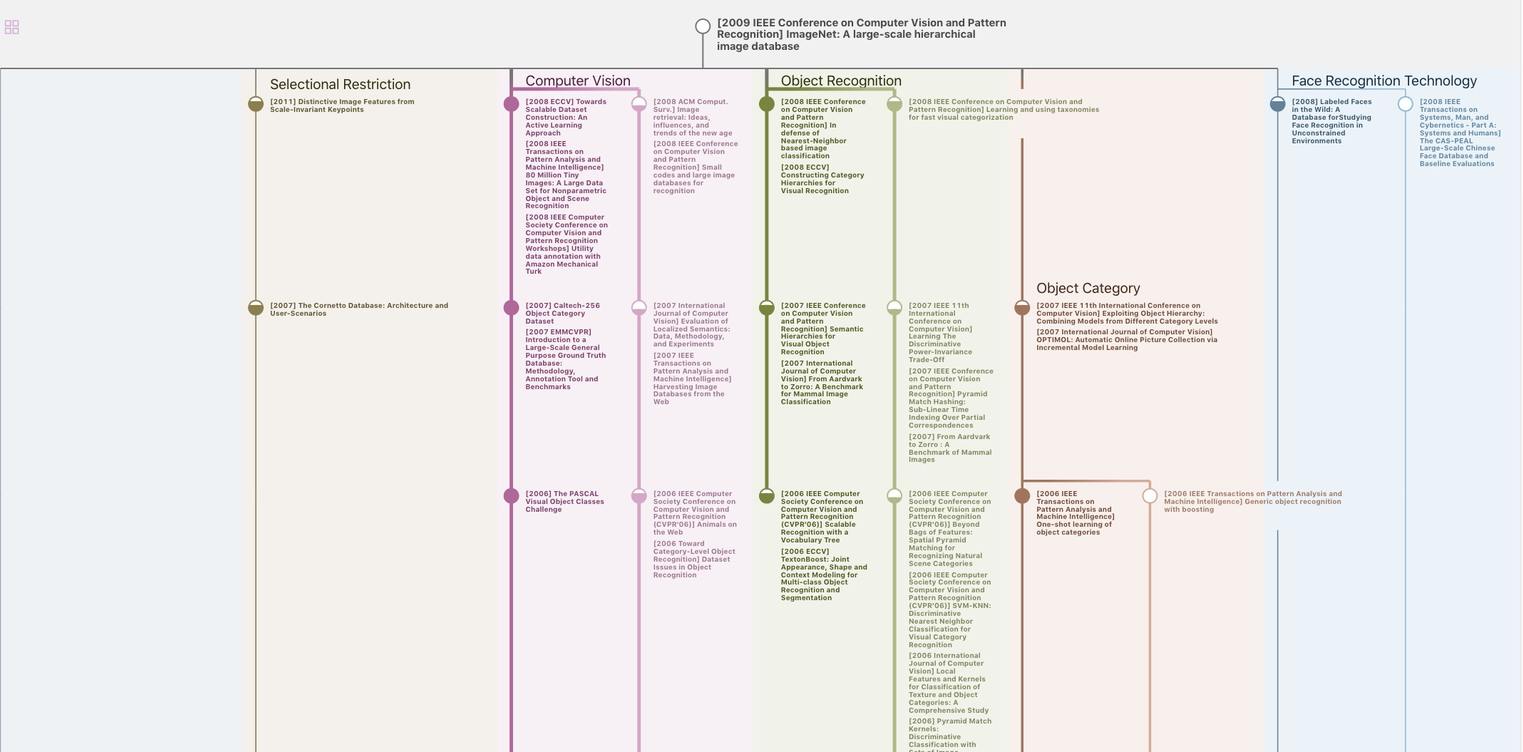
生成溯源树,研究论文发展脉络
Chat Paper
正在生成论文摘要