TRACE: Travel Reinforcement Recommendation Based on Location-Aware Context Extraction
ACM Transactions on Knowledge Discovery from Data(2022)
摘要
AbstractAs the popularity of online travel platforms increases, users tend to make ad-hoc decisions on places to visit rather than preparing the detailed tour plans in advance. Under the situation of timeliness and uncertainty of users’ demand, how to integrate real-time context into dynamic and personalized recommendations have become a key issue in travel recommender system. In this article, by integrating the users’ historical preferences and real-time context, a location-aware recommender system called TRACE (Travel Reinforcement Recommendations Based on Location-Aware Context Extraction) is proposed. It captures users’ features based on location-aware context learning model, and makes dynamic recommendations based on reinforcement learning. Specifically, this research: (1) designs a travel reinforcing recommender system based on an Actor-Critic framework, which can dynamically track the user preference shifts and optimize the recommender system performance; (2) proposes a location-aware context learning model, which aims at extracting user context from real-time location and then calculating the impacts of nearby attractions on users’ preferences; and (3) conducts both offline and online experiments. Our proposed model achieves the best performance in both of the two experiments, which demonstrates that tracking the users’ preference shifts based on real-time location is valuable for improving the recommendation results.
更多查看译文
关键词
Travel recommender system, reinforcement learning, location-aware context learning
AI 理解论文
溯源树
样例
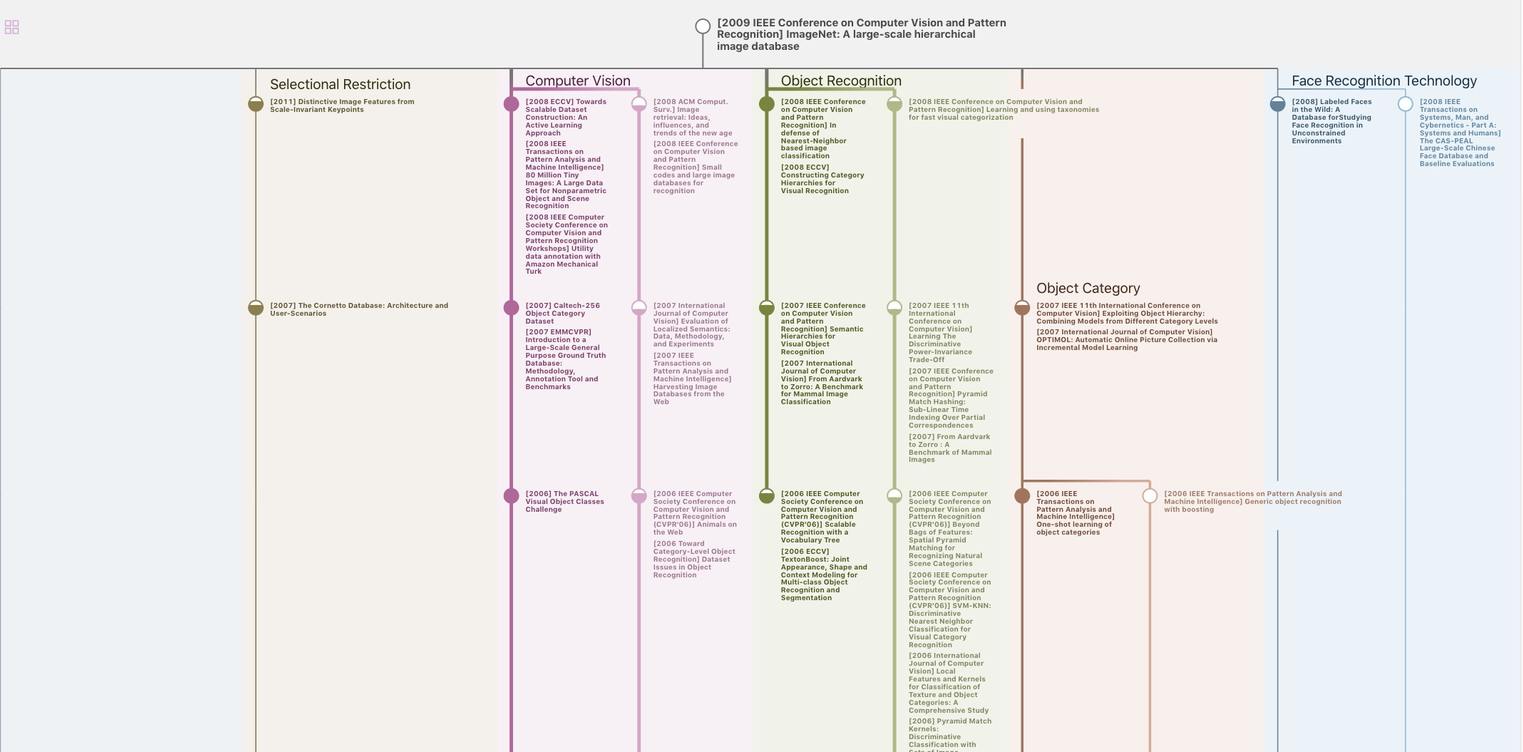
生成溯源树,研究论文发展脉络
Chat Paper
正在生成论文摘要