Knowledge Distillation with Metric Learning for Medical Dialogue Generation.
BIBM(2021)
摘要
In recent years, the research of the medical dialogue system has attracted much attention. Considering that in the dialogue system, queries with similar meanings tend to have similar replies. In the medical field, this phenomenon is even more prevalent. For queries of the same class, their corresponding replies typically have similar meanings and can be classified into the same category. Having observed that, we propose to improve the neural sequence-to-sequence (Seq2Seq) based medical dialogue system by utilizing this internal relationship of category information between queries and replies. In our model, we first cluster similar queries into the same category according to their query vectors obtained from the encoder. Then we put forward the indirect and direct distillation learning approach to transfer the category information and category center distance from the queries to the replies. In the indirect distillation process, we employ metric learning to learn better representations of replies, in which replies of corresponding queries in the same category are closely grouped together, whereas those with different categories are far apart. In the direct distillation, to transfer the inter-class relationship, we minimize the Kullback-Leibler (KL) divergence between the category center distance distribution of queries and replies. A large number of experimental results on medical datasets have proved that our method is superior to the most advanced one.
更多查看译文
关键词
Medical dialogue generation,Category,Seq2Seq,Knowledge distillation,Metric learning
AI 理解论文
溯源树
样例
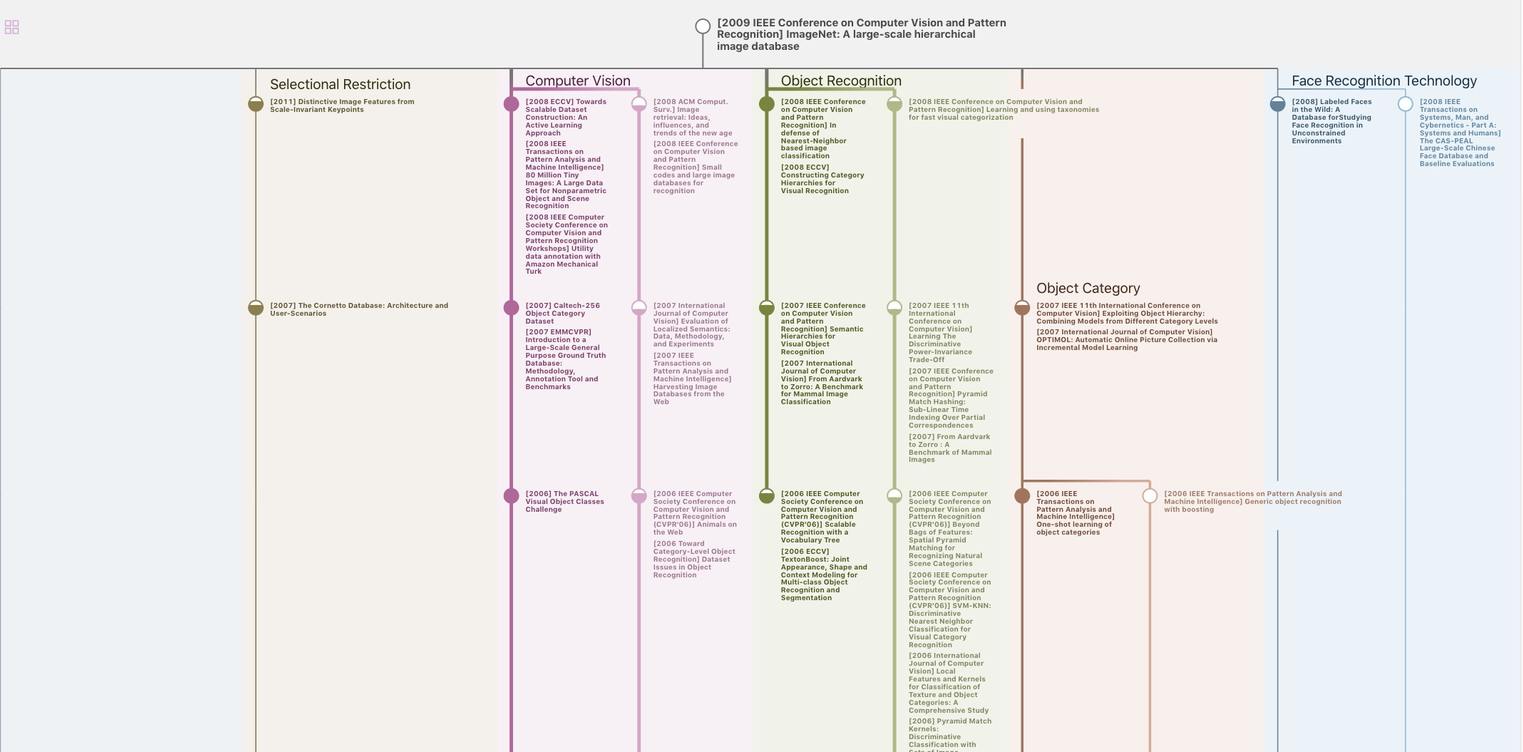
生成溯源树,研究论文发展脉络
Chat Paper
正在生成论文摘要