Low-Dimensional Depth Local Dual-View Features Embedded Transformer for Electrocardiogram Signal Quality Assessment.
BIBM(2021)
摘要
Electrocardiogram(ECG) signal quality assessment(SQA) plays an essential role in significantly improving the ECG signal quality. However, the reliability and accuracy of SQA based on wearables in free-living conditions are extremely limited by various noises such as motion artifacts(MA), which have morphology similar to some of the ECG signals. We propose a novel ECG SQA method based on low-dimensional depth local dual-view(DLDV) features and the Transformer model. First, the Empirical Mode Decomposition (EMD) and Fast Fourier Transform (FFT) are combined to achieve the DLDV (the DLDV features can distinguish the nuances between the ECG and MA) feature extraction. Then, the proposed Transformer based model can embed DLDV features and the ECG signal into low-dimensional space and then use the embedded feature vectors to train the classifier, which classifies signal segments into “acceptable” or “unacceptable” categories to achieve SQA. Note that the low-dimensional embedding makes it easier and faster for Transformer to learn features that can distinguish the local nuances between the ECG and MA. Finally, we conduct extensive experiments on the Physionet Computing in Cardiology Challenge 2011 database using both the original and balanced datasets. Experimental results show that the accuracy achieves 99.82%. We also test the contribution of different features to quality classification, and the experiment proved that the DLDV features are the most improved classification accuracy(from 97.53% to 99.82%). Compared with the state-of-the-art methods, the proposed method achieves the best classification result and shows superior performance.
更多查看译文
关键词
signal quality assessment,depth local dual-view(DLDV) features,motion artifacts,Transformer
AI 理解论文
溯源树
样例
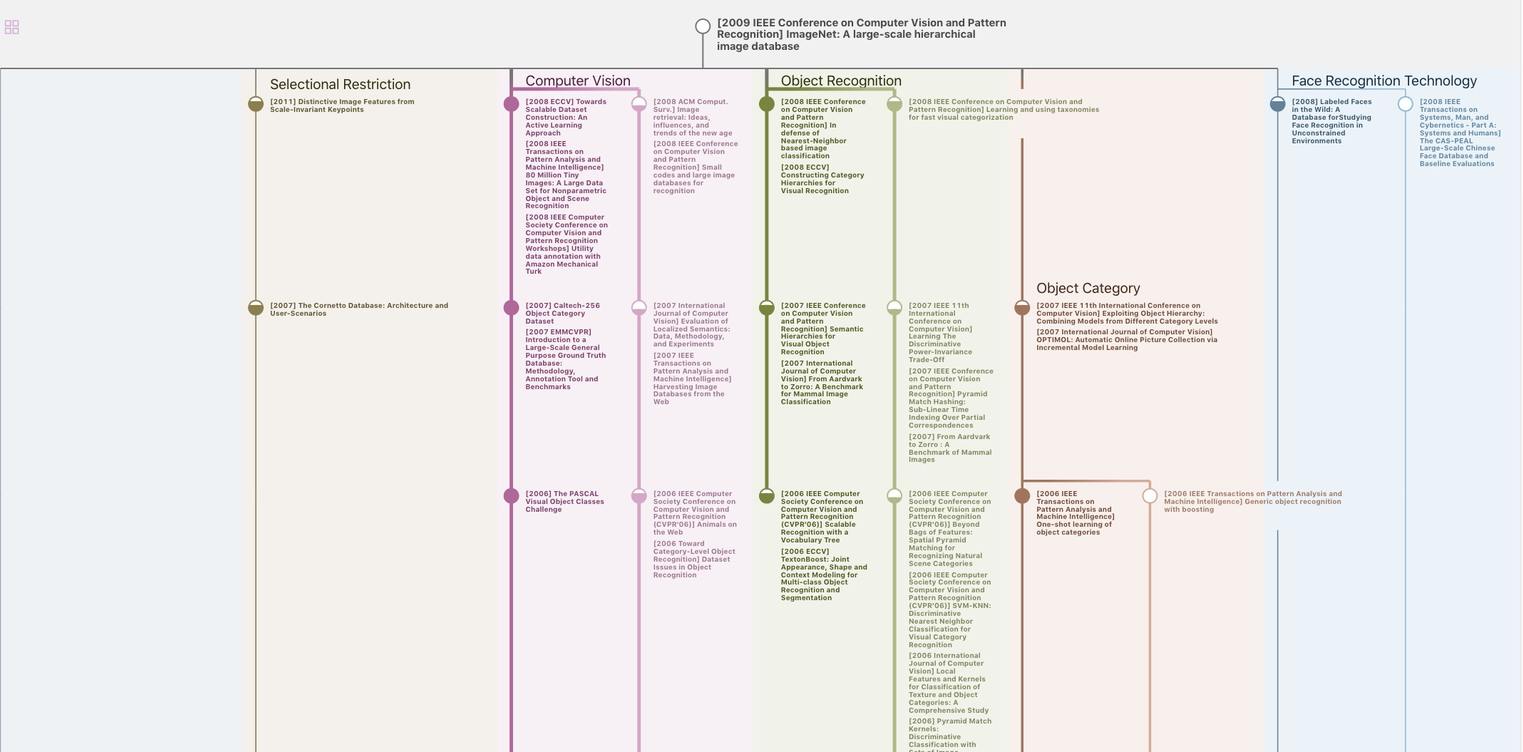
生成溯源树,研究论文发展脉络
Chat Paper
正在生成论文摘要