Optimizing Federated Learning on Non-IID Data Using Local Shapley Value.
CICAI(2021)
摘要
Federated learning (FL) was originally proposed as a new distributed machine learning paradigm that addresses the data security and privacy protection issues with a global model trained by ubiquitous local data. Currently, FL techniques have been applied in some data-sensitive areas such as finance, insurance, and healthcare. Although FL has broad application scenarios, there are still some significant and fundamental challenges, one of which is the training on Not independently and identically distributed (Non-IID) data. More concretely, the global model aggregation and collaboration of a massive number of participants on the Non-IID data remain an unsolved problem. We find that most of the model aggregation optimization algorithms in the literature suffer from significant accuracy loss in the Non-IID setting for FL . To this end, in this paper, we propose a novel model aggregation algorithm terms FedSV , which dynamically updates global model aggregation weights according to each local participant’s contribution in each training round. Furthermore, to evaluate the participants’ contribution, we propose a quantization algorithm based on Local Federated Shapley Value , which dynamically computes the contribution by the properties of the participant. Extensive experiments on Non-IID data partition, such as CIFAR-10 and MNIST, demonstrate that our approach can improve accuracy during training compared with existing methods.
更多查看译文
关键词
Federated learning, Model aggregation, Shapley value
AI 理解论文
溯源树
样例
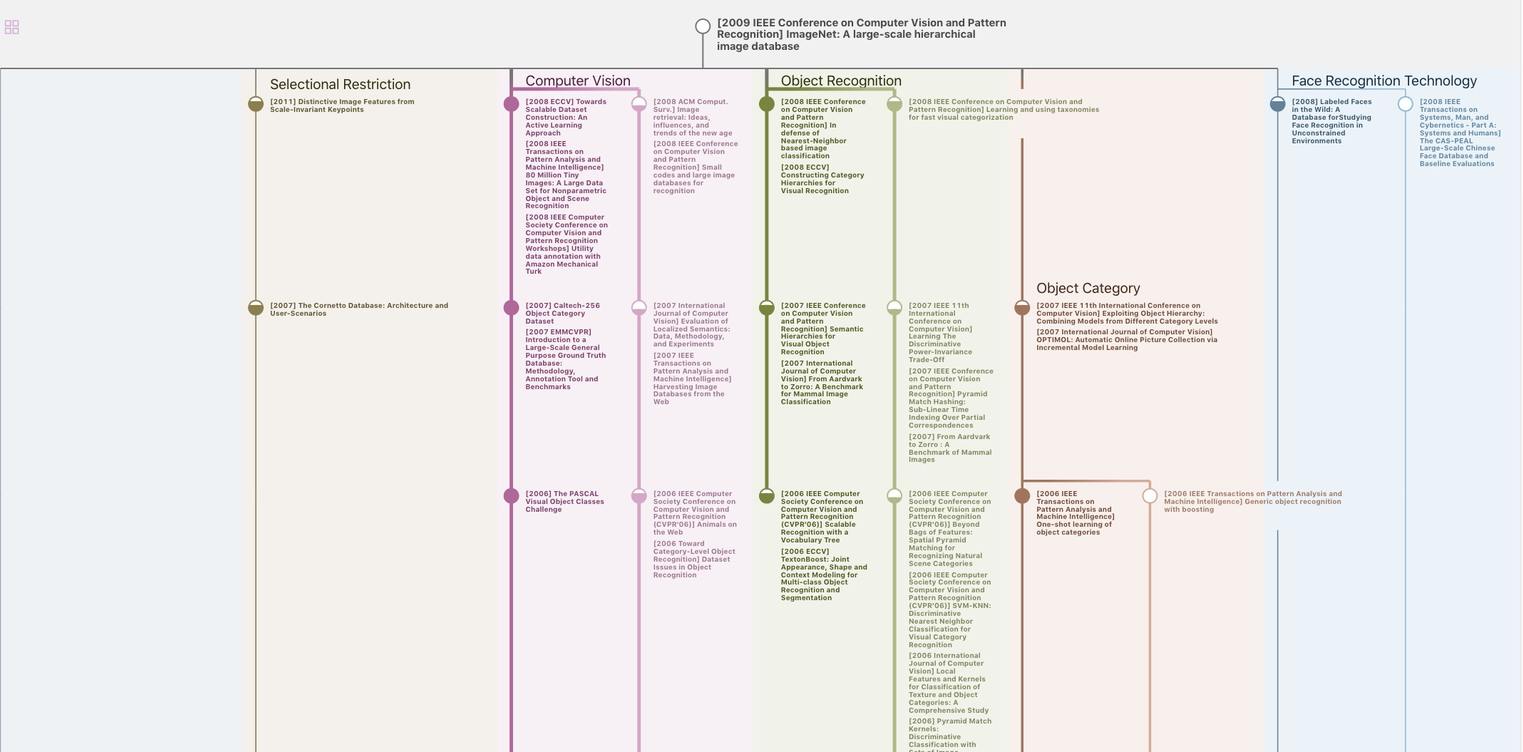
生成溯源树,研究论文发展脉络
Chat Paper
正在生成论文摘要