Fooling Neural Network Interpretations - Adversarial Noise to Attack Images.
CICAI(2021)
摘要
The accurate interpretation of neural network about how the network works is important. However, a manipulated explanation may have the potential to mislead human users not to trust a reliable network. Therefore, it is necessary to verify interpretation algorithms by designing effective attacks to simulate various possible threats in the real world. In this work, we mainly explore how to mislead interpretation. More specifically, we optimize the noise added to the input, which aims to highlight a certain area that we specify without changing the output category of network. With our proposed algorithm, we demonstrate that the state-of-the-art saliency maps based interpreters, e.g., Grad-CAM, Guided-Feature-Inversion, Grad-CAM++, Score-CAM and Full-Grad can be easily fooled. We propose two situations of fooling, Single-target attack and Multi-target attack, and show that the fooling can be transfered to different interpretation methods as well as generalized to the unseen samples with the universal noise. We also take image patches to fool Grad-CAM. Our results are proved in both qualitative and quantitative ways and we further propose a quantitative metric to measure the effectiveness of algorithm. We believe that our method can serve as an additional evaluation of robustness for future interpretation algorithms.
更多查看译文
关键词
adversarial noise,attack images,neural network
AI 理解论文
溯源树
样例
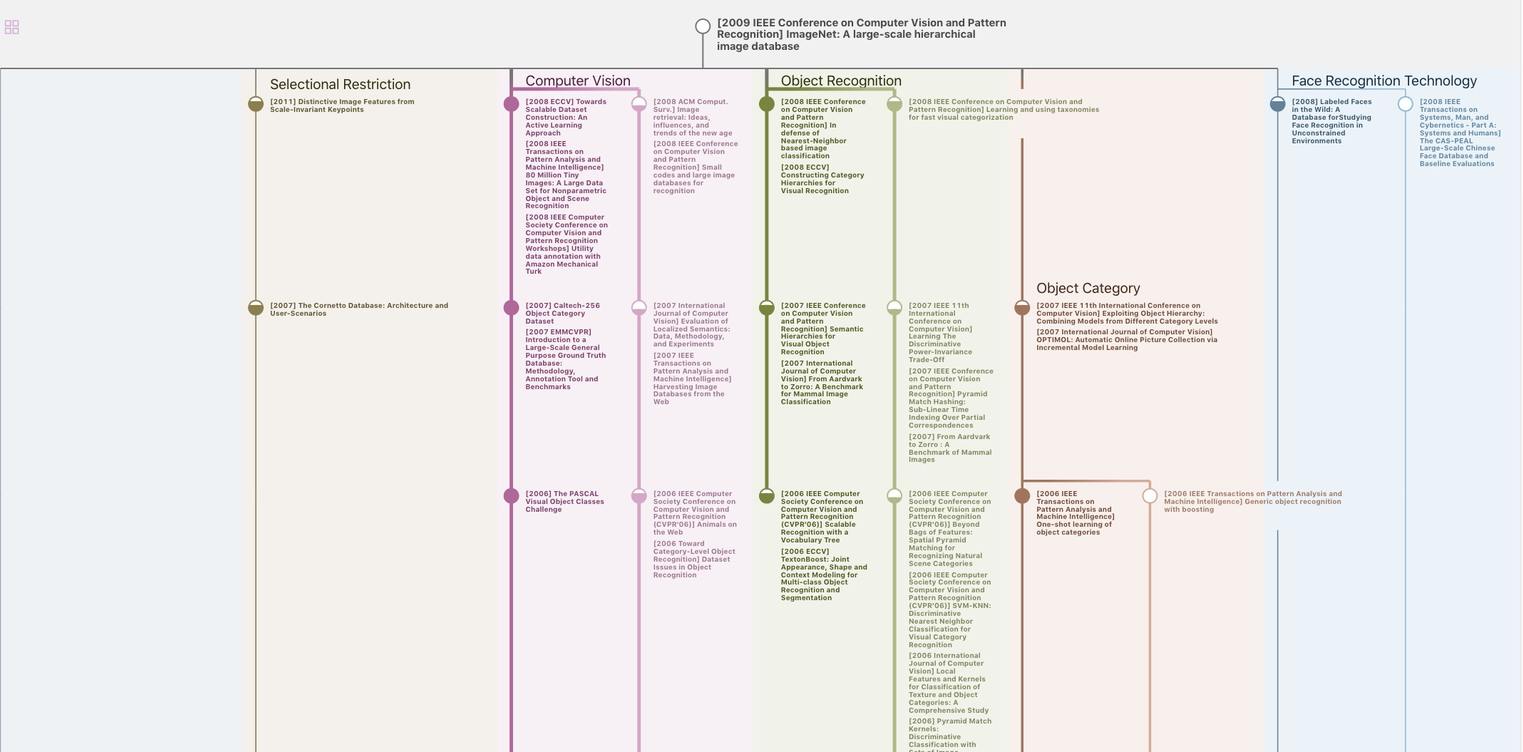
生成溯源树,研究论文发展脉络
Chat Paper
正在生成论文摘要