Matrix Profile Index Approximation for Streaming Time Series.
IEEE BigData(2021)
摘要
Discovery of motifs (repeated patterns) in time series is a key factor across numerous industries and scientific fields. These and related problems have effectively been solved for offline analysis of time series; however, these approaches are computationally intensive and do not lend themselves to streaming time series, where the sampling rate imposes real-time constraints on computation and there is strong desire to locate computation as close as possible to the sensor. One promising solution is to use low-cost machine learning models to provide approximate answers to these problems. For example, prior work has trained models to predict the similarity of the most recently sampled window of data points to a representative time series used for training. This work addresses a more challenging problem: to predict not only the "strength" of the match, but also the relative location in the representative time series where the match occurs. We evaluate our approach on two different real world datasets; we demonstrate speedups as high as 40x compared to exact computations, with predictive accuracy as high as 87.9%, depending on the granularity of the prediction.
更多查看译文
关键词
Streaming time series,Matrix Profile,Indexing
AI 理解论文
溯源树
样例
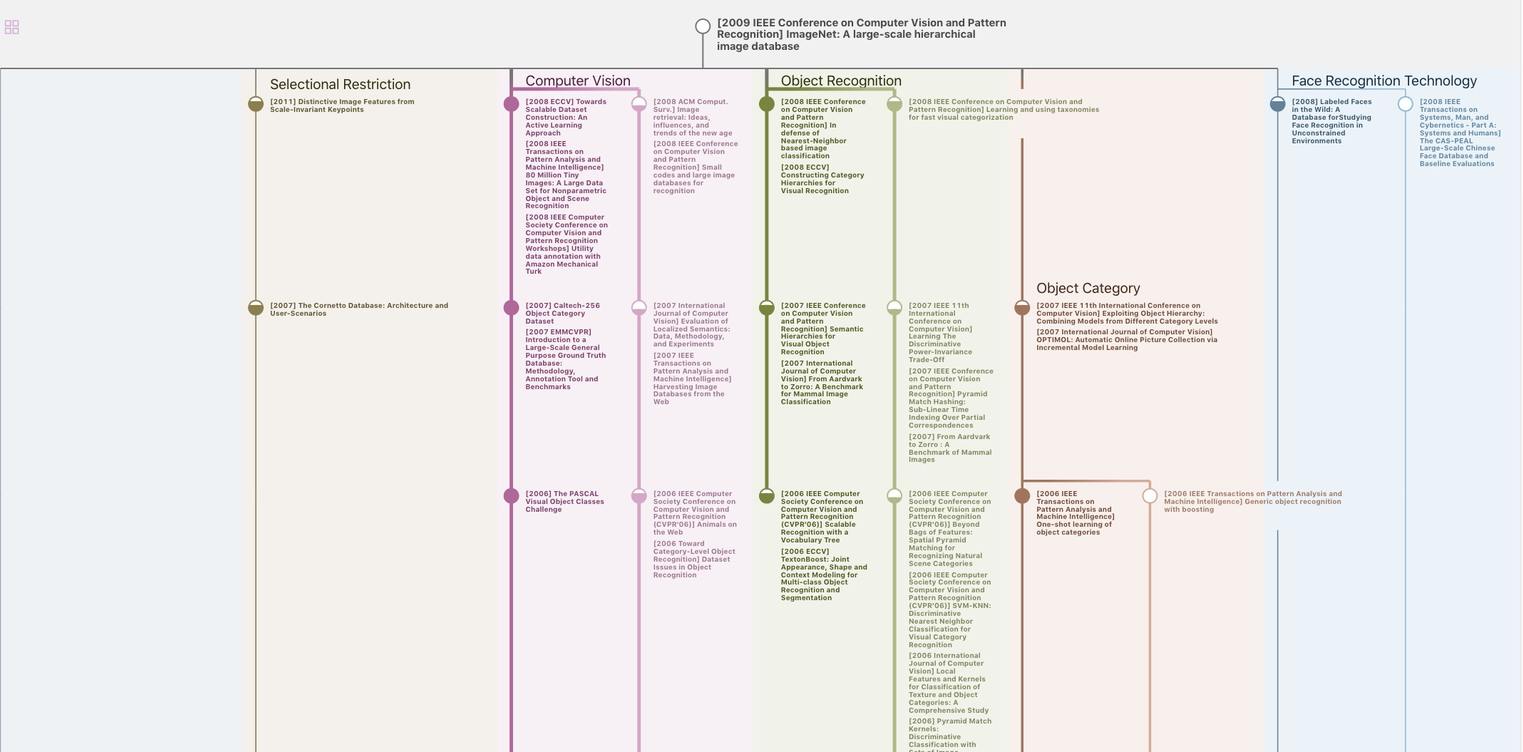
生成溯源树,研究论文发展脉络
Chat Paper
正在生成论文摘要