CyBERT - Contextualized Embeddings for the Cybersecurity Domain.
IEEE BigData(2021)
摘要
We present CyBERT, a domain-specific Bidirectional Encoder Representations from Transformers (BERT) model, fine-tuned with a large corpus of textual cybersecurity data. State-of-the-art natural language models that can process dense, fine-grained textual threat, attack, and vulnerability information can provide numerous benefits to the cybersecurity community. The primary contribution of this paper is providing the security community with an initial fine-tuned BERT model that can perform a variety of cybersecurity-specific downstream tasks with high accuracy and efficient use of resources. We create a cybersecurity corpus from open-source unstructured and semi-unstructured Cyber Threat Intelligence (CTI) data and use it to fine-tune a base BERT model with Masked Language Modeling (MLM) to recognize specialized cybersecurity entities. We evaluate the model using various downstream tasks that can benefit modern Security Operations Centers (SOCs). The fine-tuned CyBERT model outperforms the base BERT model in the domain-specific MLM evaluation. We also provide use-cases of CyBERT application in cybersecurity based downstream tasks.
更多查看译文
关键词
cybersecurity corpus,Masked Language Modeling,specialized cybersecurity entities,fine-tuned CyBERT model,domain-specific MLM evaluation,CyBERT application,contextualized embeddings,cybersecurity domain,textual cybersecurity data,natural language models,fine-grained textual threat,vulnerability information,cybersecurity community,security community,initial fine-tuned BERT model,cybersecurity-specific downstream tasks,semiunstructured cyber threat intelligence data,domain-specific bidirectional encoder representations from transformer model
AI 理解论文
溯源树
样例
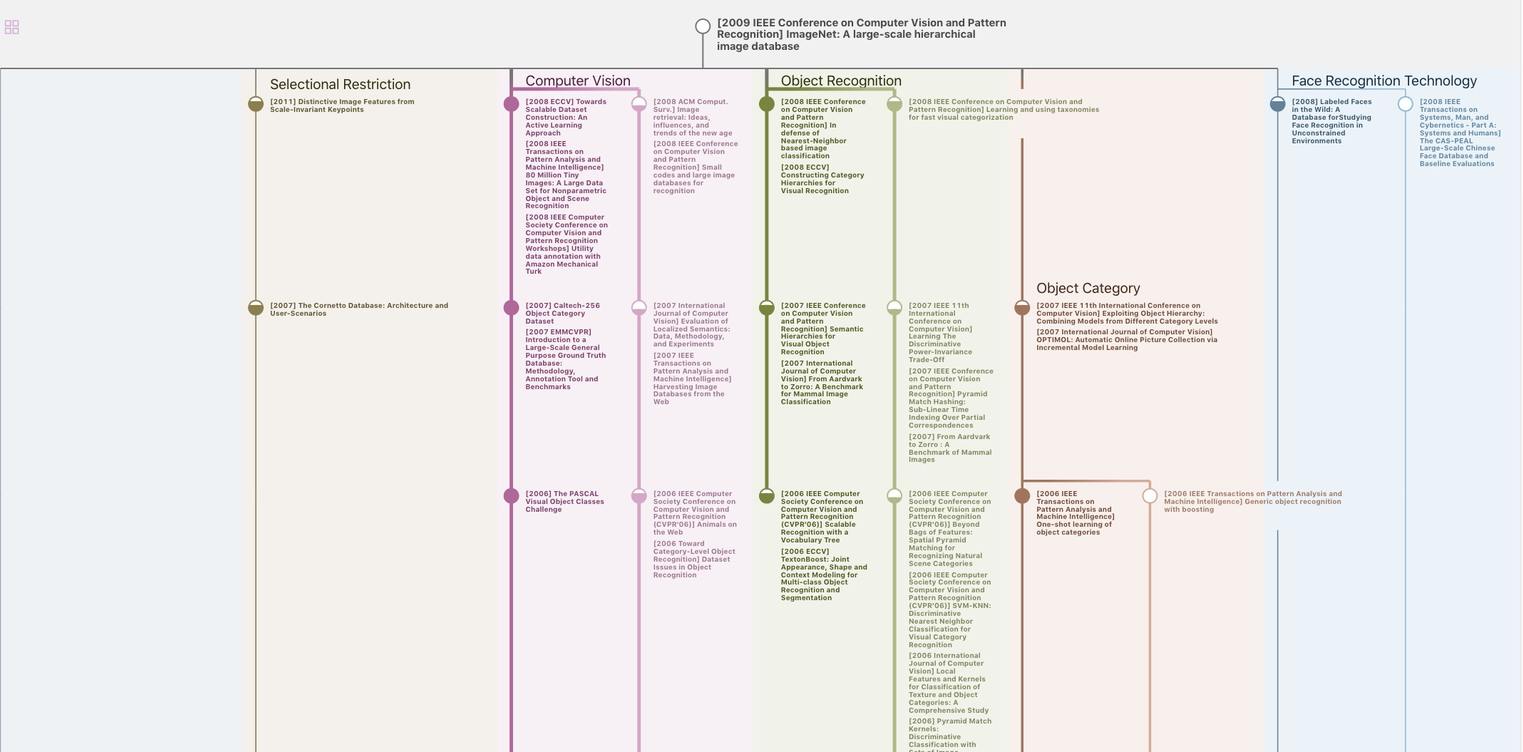
生成溯源树,研究论文发展脉络
Chat Paper
正在生成论文摘要