Customer Support Chat Intent Classification using Weak Supervision and Data Augmentation.
COMAD/CODS(2022)
摘要
Understanding the actual intent of customers is an essential step in automating the conversational experience on a chat platform. Typically, chatbots are powered by machine learning algorithms that rely on the acquisition of a large amount of high quality labeled training data which can be prohibitively expensive. To overcome this dependence on labeled training data, weaker forms of supervision have been recently exploited to generate samples in a more cost effective manner though the samples may be noisy. In this paper, we analyse a use-case specific to food delivery services where customer-agent conversations in incoherent English and code-mixed language (Hindi mixed with English, commonly referred to as Hinglish) are associated with a single customer chosen noisy label (referred to as “Conversation Level Intent” in the current paper) for the entire conversation. However, in reality, a conversation can have several messages and can be comprised of multiple labels. Moreover, each label may be associated with one or more messages in the conversation. In this paper, we demonstrate how simple light-weight word embeddings based weak supervision techniques can be used to tag individual customer messages with the most relevant label. We also show that simple augmentation techniques can significantly improve performance on code-mixed messages. On an internal benchmark dataset, we show that our sampling approach achieves an absolute performance gain of 33% in F1 score on random sampling strategy, 19% in F1 score over an approach using entire raw samples and 4% over a Snorkel (a state-of-the-art weak supervision framework) based approach.
更多查看译文
AI 理解论文
溯源树
样例
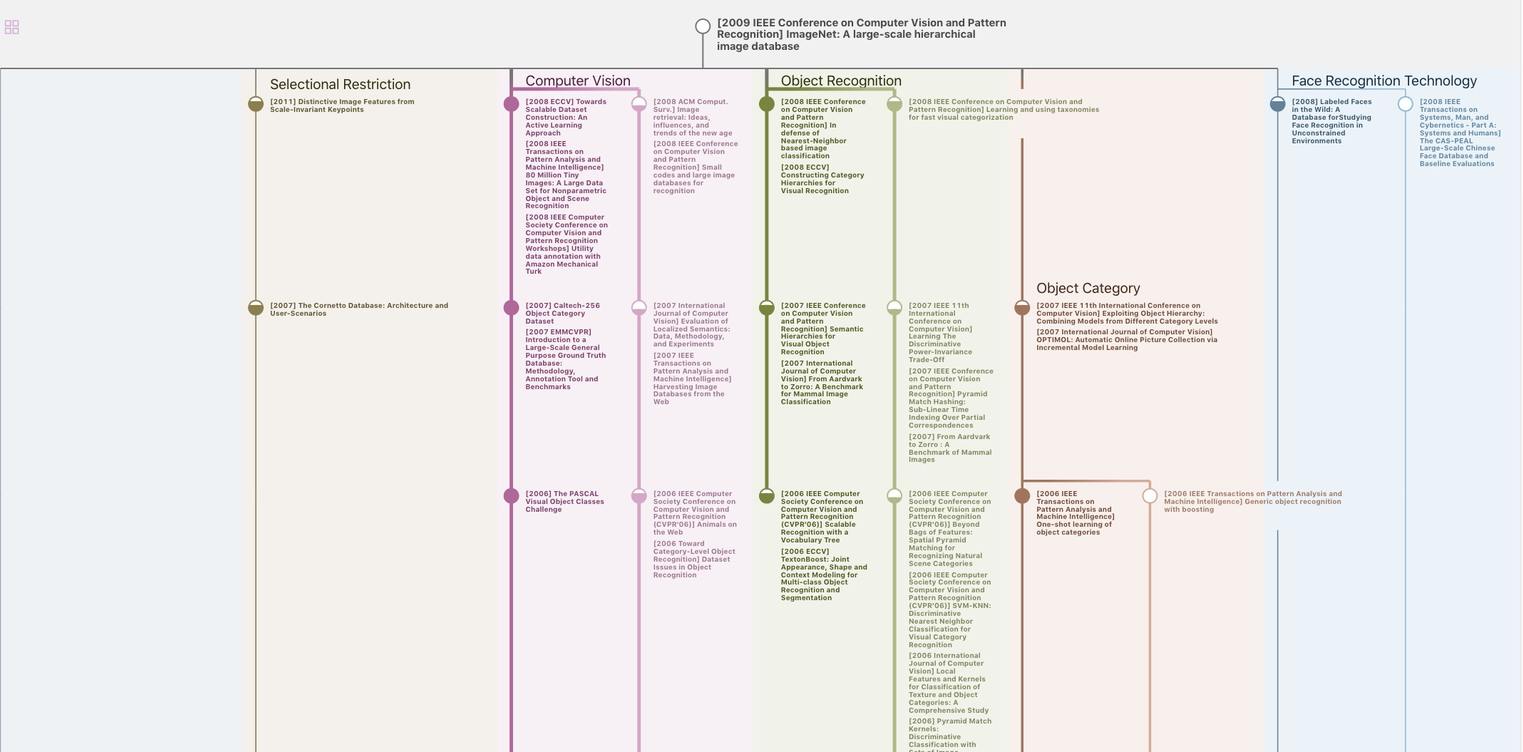
生成溯源树,研究论文发展脉络
Chat Paper
正在生成论文摘要