A holistic framework for prediction of routing attacks in IoT-LLNs
The Journal of Supercomputing(2021)
摘要
The IPv6 routing protocol for low power and lossy networks (RPL) has gained widespread application in the Internet of Things (IoT) environment. RPL has inherent security features to restrict external attacks. However, internal attacks in the IoT environment have continued to grow due to the lack of mechanisms to manage the secure identities and credentials of the billions of heterogeneous IoT devices. Weak credentials aid attackers in gaining access to IoT devices and further exploiting vulnerabilities stemming from the underlying routing protocols. Routing attacks degrade the performance of IoT networks by compromising the network resources, topology, and traffic. In this paper, we propose a holistic framework for the prediction of routing attacks in RPL-based IoT. The framework leverages Graph Convolution Network-based network embedding to capture and learn the latent state of the nodes in the IoT network. It uses a Long Short Term Memory model to predict network traffic. The framework incorporates a Feedforward Neural Network that uses network embedding and traffic prediction as input to predict routing attacks. The accuracy of any learning model depends on the integrity of the data provided to it as input. Therefore, the framework uses smart contract-fortified blockchain technology to establish secure channels for IoT data access. The smart contract within the blockchain generates warning impulses in the case of abnormal behavior of nodes. The framework predicts normal scenarios, resource attack scenarios, traffic attack scenarios, and topological attack scenarios with a fair accuracy of 94.5%, 82.46%, 91.88%, and 86.13%, respectively.
更多查看译文
关键词
IoT,RPL,Routing attacks,Network embeddings,Smart contract
AI 理解论文
溯源树
样例
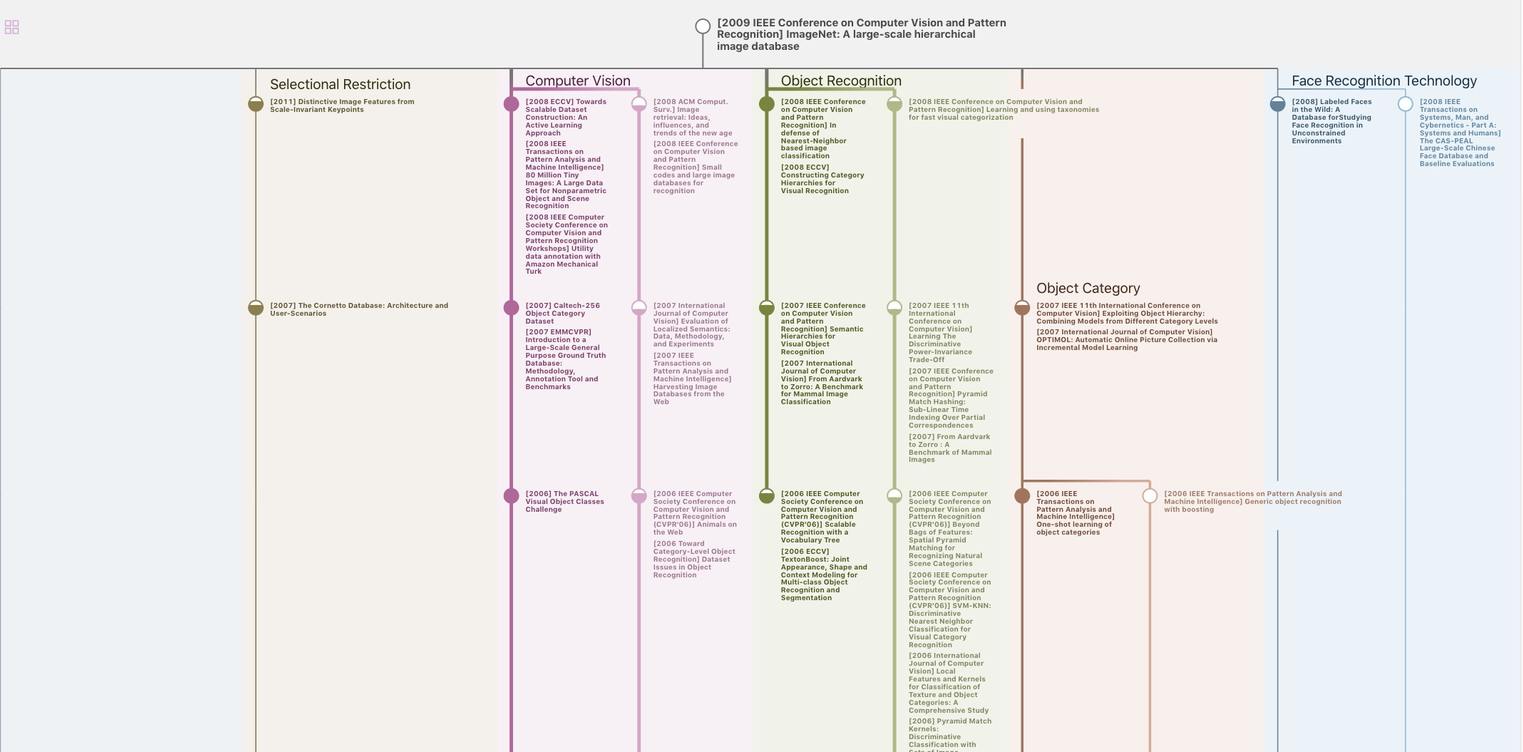
生成溯源树,研究论文发展脉络
Chat Paper
正在生成论文摘要