Density Peaks Clustering Based on Feature Reduction and Quasi-Monte Carlo
SCIENTIFIC PROGRAMMING(2022)
摘要
Density peaks clustering (DPC) is a well-known density-based clustering algorithm that can deal with nonspherical clusters well. However, DPC has high computational complexity and space complexity in calculating local density rho and distance delta, which makes it suitable only for small-scale data sets. In addition, for clustering high-dimensional data, the performance of DPC still needs to be improved. High-dimensional data not only make the data distribution more complex but also lead to more computational overheads. To address the above issues, we propose an improved density peaks clustering algorithm, which combines feature reduction and data sampling strategy. Specifically, features of the high-dimensional data are automatically extracted by principal component analysis (PCA), auto-encoder (AE), and t-distributed stochastic neighbor embedding (t-SNE). Next, in order to reduce the computational overhead, we propose a novel data sampling method for the low-dimensional feature data. Firstly, the data distribution in the low-dimensional feature space is estimated by the Quasi-Monte Carlo (QMC) sequence with low-discrepancy characteristics. Then, the representative QMC points are selected according to their cell densities. Next, the selected QMC points are used to calculate rho and delta instead of the original data points. In general, the number of the selected QMC points is much smaller than that of the initial data set. Finally, a two-stage classification strategy based on the QMC points clustering results is proposed to classify the original data set. Compared with current works, our proposed algorithm can reduce the computational complexity from O(n(2)) to O(Nn), where N denotes the number of selected QMC points and n is the size of original data set, typically N << n. Experimental results demonstrate that the proposed algorithm can effectively reduce the computational overhead and improve the model performance.
更多查看译文
关键词
clustering,feature reduction,density,quasi-monte
AI 理解论文
溯源树
样例
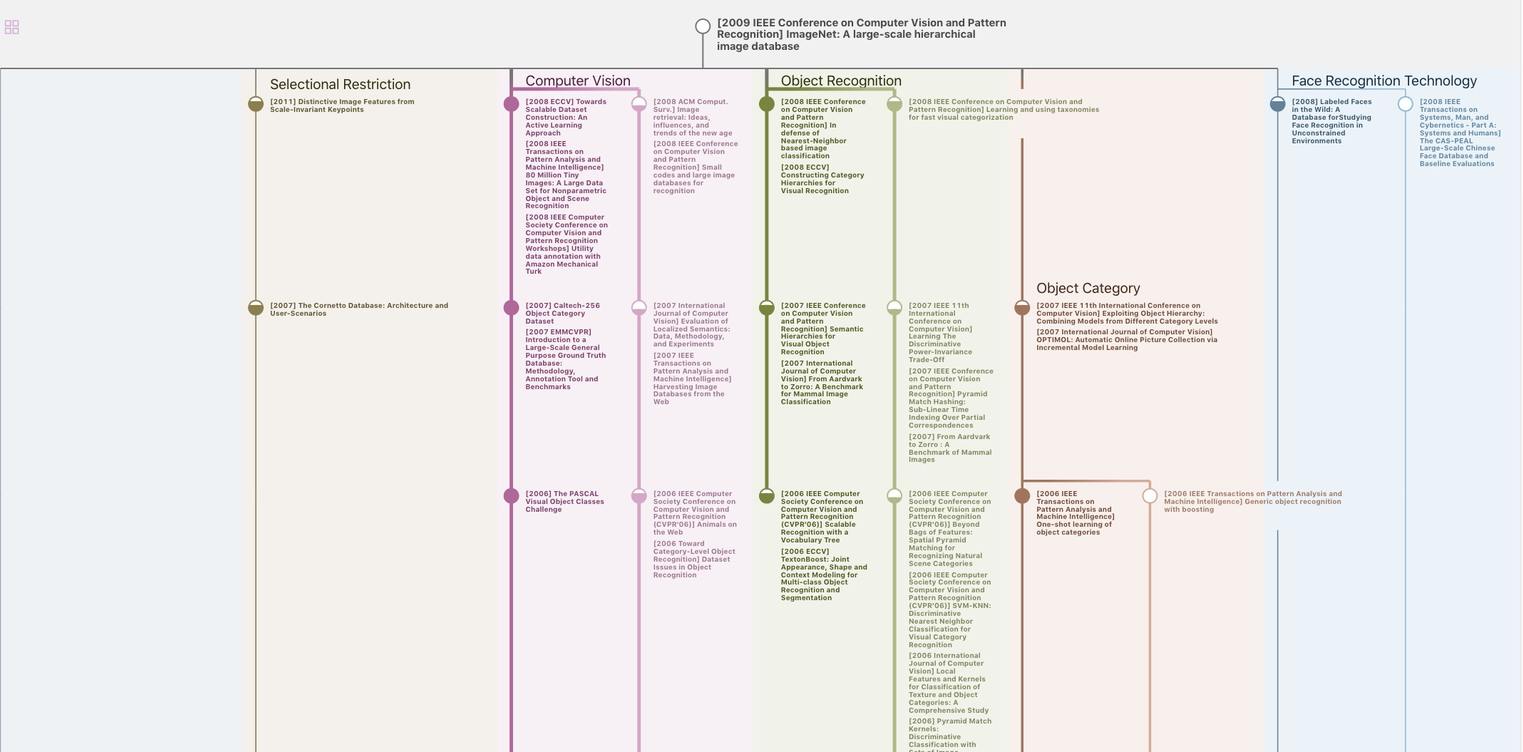
生成溯源树,研究论文发展脉络
Chat Paper
正在生成论文摘要