DeepFeat: Robust Large-Scale Multi-Features Outdoor Localization in LTE Networks Using Deep Learning
IEEE ACCESS(2022)
摘要
In today's Telecom market, Telecom operators find a big value in introducing valuable services to users based on their location, both for emergency and ordinary situations. This drives the research for outdoor localization using different wireless technologies. Long Term Evolution (LTE) is the dominant wireless technology for outdoor cellular networks. This paper introduces DeepFeat: A deep-learning-based framework for outdoor localization using a rich features set in LTE networks. DeepFeat works on the mobile operator side, and it leverages many mobile network features and other metrics to achieve high localization accuracy. In order to reduce computation and complexity, we introduce a feature selection module to choose the most appropriate features as inputs to the deep learning model. This module reduces the computation and complexity by around 20.6% while enhancing the system's accuracy. The feature selection module uses correlation and Chi-squared algorithms to reduce the feature set to 12 inputs only regardless of the area size. In order to enhance the accuracy of DeepFeat, a One-to-Many augmenter is introduced to extend the dataset and improve the system's overall performance. The results show the impact of the proper features selection adopted by DeepFeat on the system's performance. DeepFeat achieved median localization accuracy of 13.179m in an outdoor environment in a mid-scale area of 6.27Km(2). In a large-scale area of 45Km(2), the median localization accuracy is 13.7m. DeepFeat was compared to other state-of-the-art deep-learning-based localization systems that leverage a small number of features. We show that using DeepFeat's carefully selected features set enhances the localization accuracy compared to the state-of-the-art systems by at least 286%.
更多查看译文
关键词
Data augmentation, deep learning, long-term-evolution localization, multi-features localization, outdoor localization
AI 理解论文
溯源树
样例
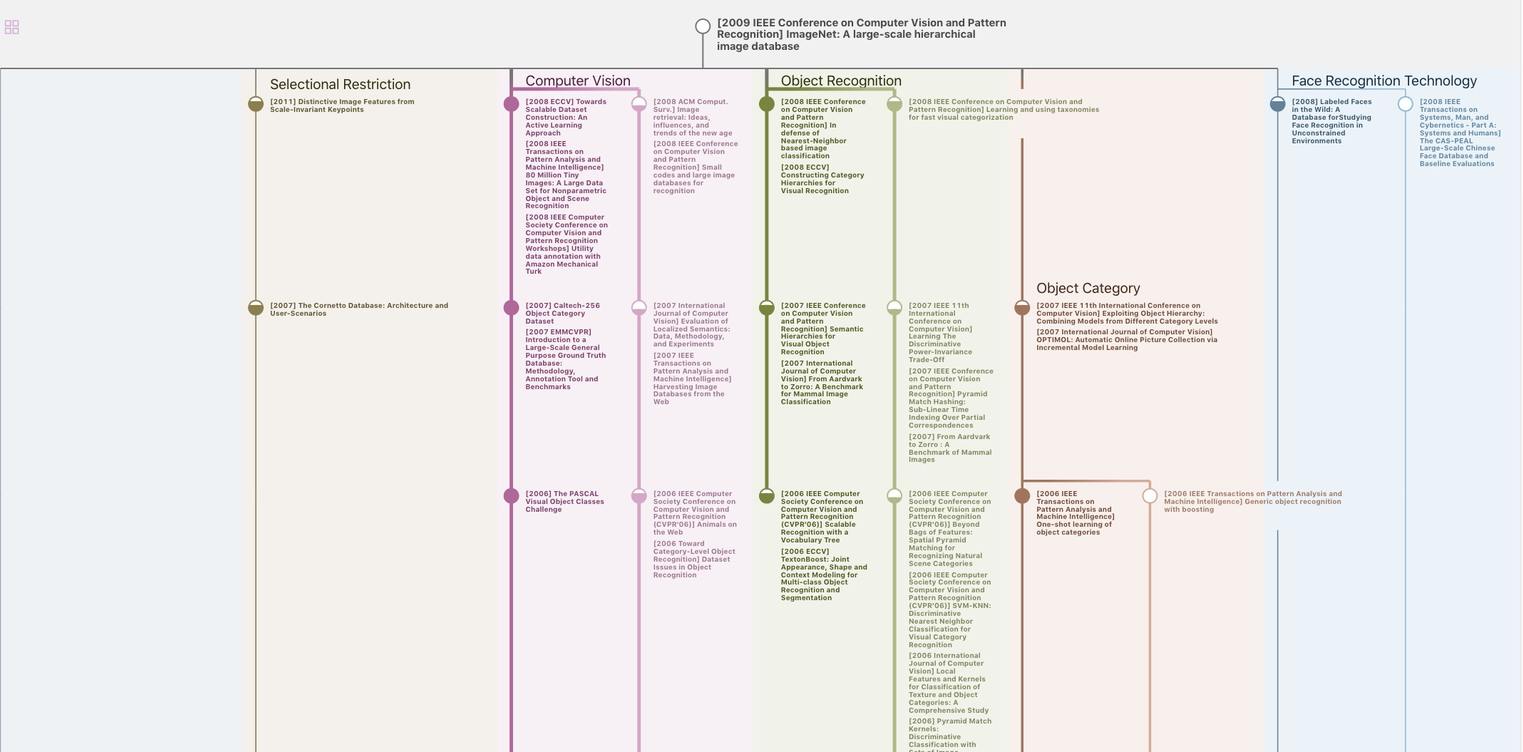
生成溯源树,研究论文发展脉络
Chat Paper
正在生成论文摘要