Improving Factored Hybrid HMM Acoustic Modeling without State Tying.
IEEE International Conference on Acoustics, Speech, and Signal Processing (ICASSP)(2022)
摘要
In this work, we show that a factored hybrid hidden Markov model (FH-HMM) which is defined without any phonetic state-tying outperforms a state-of-the-art hybrid HMM. The factored hybrid HMM provides a link to transducer models in the way it models phonetic (label) context while preserving the strict separation of acoustic and language model of the hybrid HMM approach. Furthermore, we show that the factored hybrid model can be trained from scratch without using phonetic state-tying in any of the training steps. Our modeling approach enables triphone context while avoiding phonetic state-tying by a decomposition into locally normalized factored posteriors for monophones/HMM states in phoneme context. Experimental results are provided for Switchboard 300h and LibriSpeech. On the former task we also show that by avoiding the phonetic state-tying step, the factored hybrid can take better advantage of regularization techniques during training, compared to the standard hybrid HMM with phonetic state-tying based on classification and regression trees (CART).
更多查看译文
关键词
CART-free hybrid HMM,BLSTM acoustic model,regularization,Switchboard,LibriSpeech
AI 理解论文
溯源树
样例
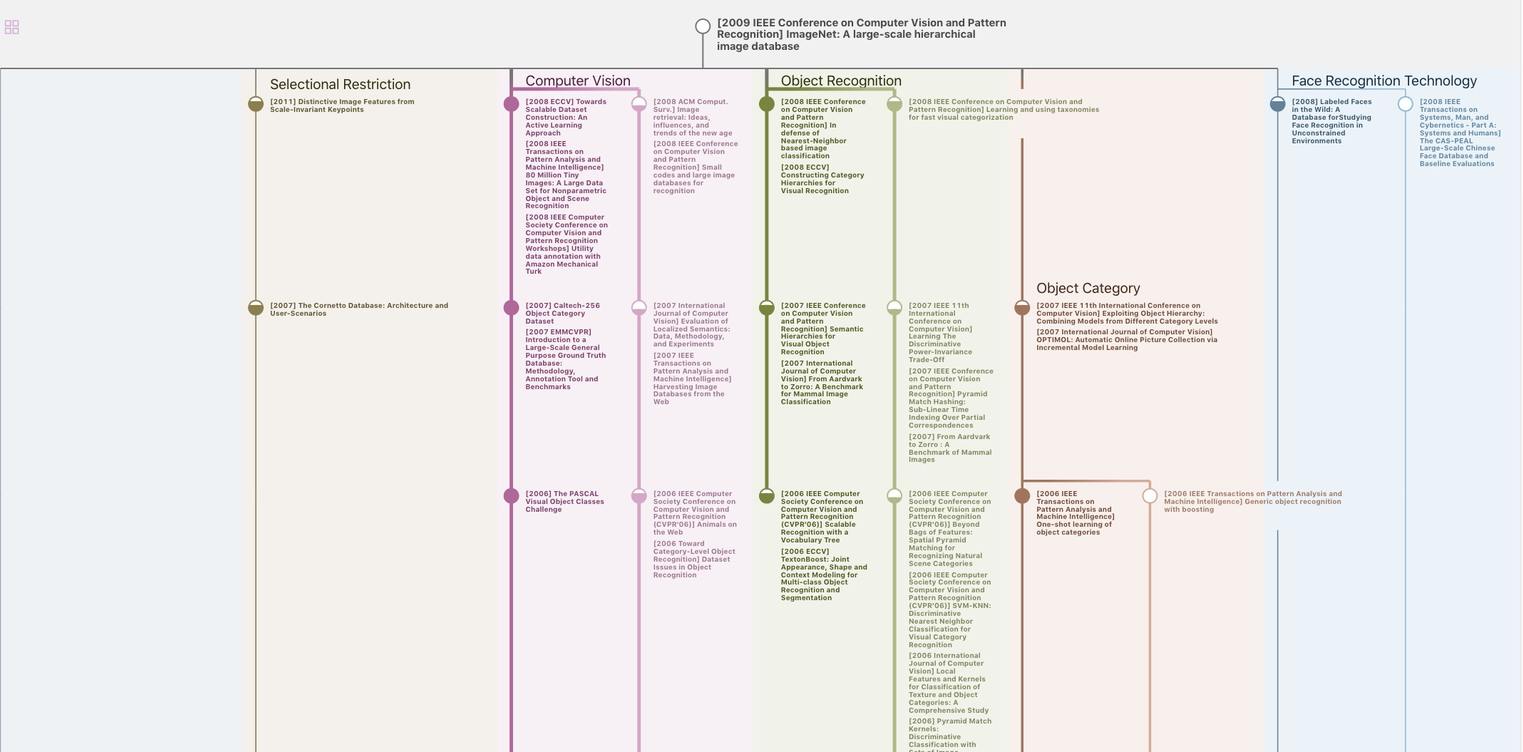
生成溯源树,研究论文发展脉络
Chat Paper
正在生成论文摘要