Deep Graph Convolutional Network and LSTM based approach for predicting drug-target binding affinity
arxiv(2022)
摘要
Development of new drugs is an expensive and time-consuming process. Due to the world-wide SARS-CoV-2 outbreak, it is essential that new drugs for SARS-CoV-2 are developed as soon as possible. Drug repurposing techniques can reduce the time span needed to develop new drugs by probing the list of existing FDA-approved drugs and their properties to reuse them for combating the new disease. We propose a novel architecture DeepGLSTM, which is a Graph Convolutional network and LSTM based method that predicts binding affinity values between the FDA-approved drugs and the viral proteins of SARS-CoV-2. Our proposed model has been trained on Davis, KIBA (Kinase Inhibitor Bioactivity), DTC (Drug Target Commons), Metz, ToxCast and STITCH datasets. We use our novel architecture to predict a Combined Score (calculated using Davis and KIBA score) of 2,304 FDA-approved drugs against 5 viral proteins. On the basis of the Combined Score, we prepare a list of the top-18 drugs with the highest binding affinity for 5 viral proteins present in SARS-CoV-2. Subsequently, this list may be used for the creation of new useful drugs.
更多查看译文
关键词
deepglstm graph convolutional network,affinity,drug-target
AI 理解论文
溯源树
样例
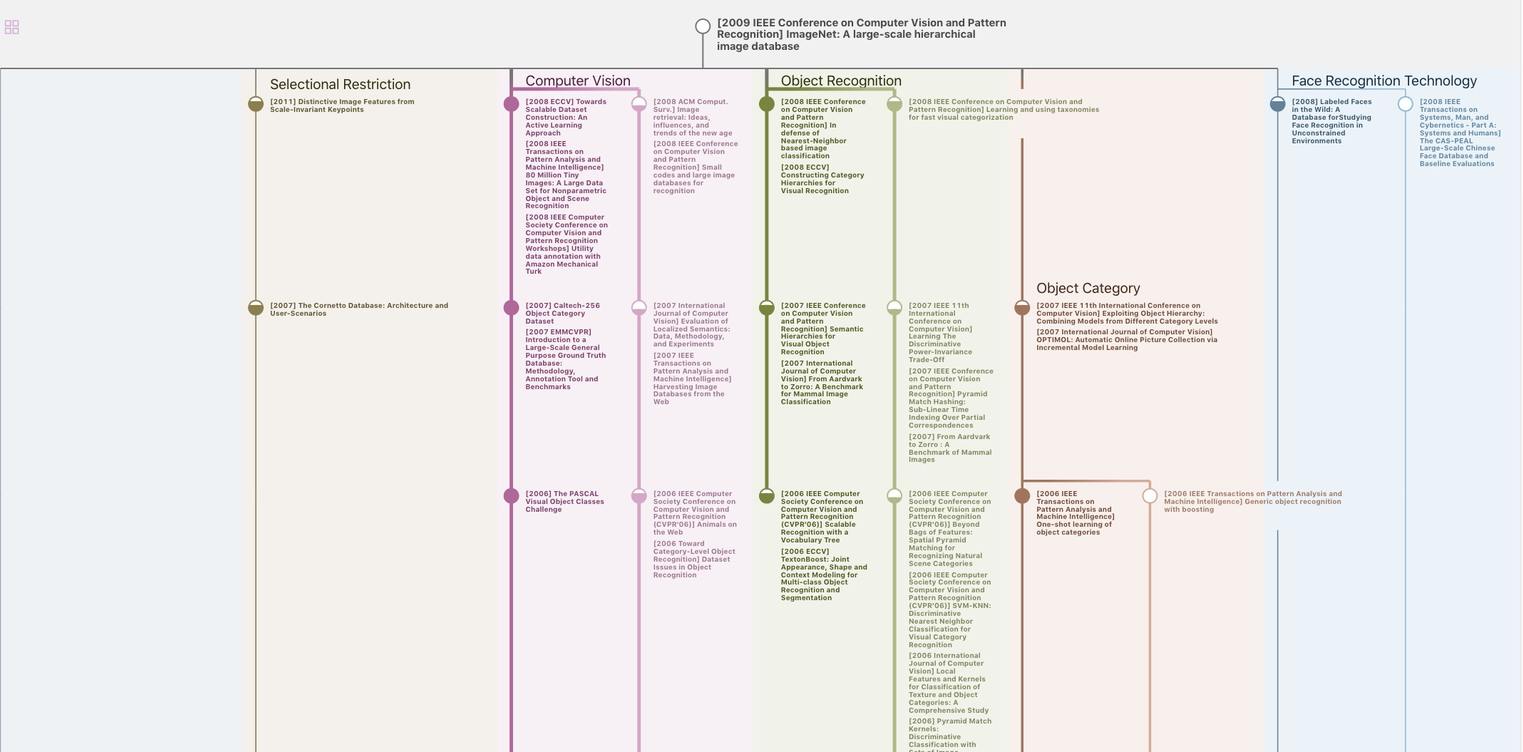
生成溯源树,研究论文发展脉络
Chat Paper
正在生成论文摘要