Efficient Training of Transfer Mapping in Physics-Infused Machine Learning Models of UAV Acoustic Field
arxiv(2022)
摘要
Physics-Infused Machine Learning (PIML) architectures aim at integrating machine learning with computationally-efficient, low-fidelity (partial) physics models, leading to improved generalizability, extrapolability, and robustness to noise, compared to pure data-driven approximation models. Recently a new PIML architecture was reported by the same authors, known as Opportunistic Physics-mining Transfer Mapping Architecture or OPTMA, which transfers the original inputs into latent features using a transfer neural network; the partial physics model then uses the latent features to generate the final output that is as close as possible to the high-fidelity output. While gradient-free solvers and back-propagation with supervised learning was earlier used to train OPTMA, that approach is computationally inefficient and challenging to generalize across different problems or popular ML implementations. This paper aims to alleviate these issues by infusing the partial physics model inside the neural network, as described via tensors in the popular ML framework, PyTorch. Such a description also naturally allows auto-differentiation (AD) of the partial physics model, thereby enabling the use of efficient back-propagation methods to train the transfer network. The benefits of the upgraded OPTMA architecture with AD (OPTMA-Net) is demonstrated by applying it to the problem of modeling the sound pressure field created by a hovering unmanned aerial vehicle (UAV). Ground truth data for this problem was obtained from an indoor UAV noise measurement setup. Here, the partial physics model is based on the interference of acoustic pressure waves generated by an arbitrary number of acoustic monopole sources. Case studies show that OPTMA-Net provides generalization performance close to, and extrapolation performance that is 4 times better than, those given by a pure data-driven model.
更多查看译文
关键词
uav acoustic field,transfer mapping,efficient training,learning models,physics-infused
AI 理解论文
溯源树
样例
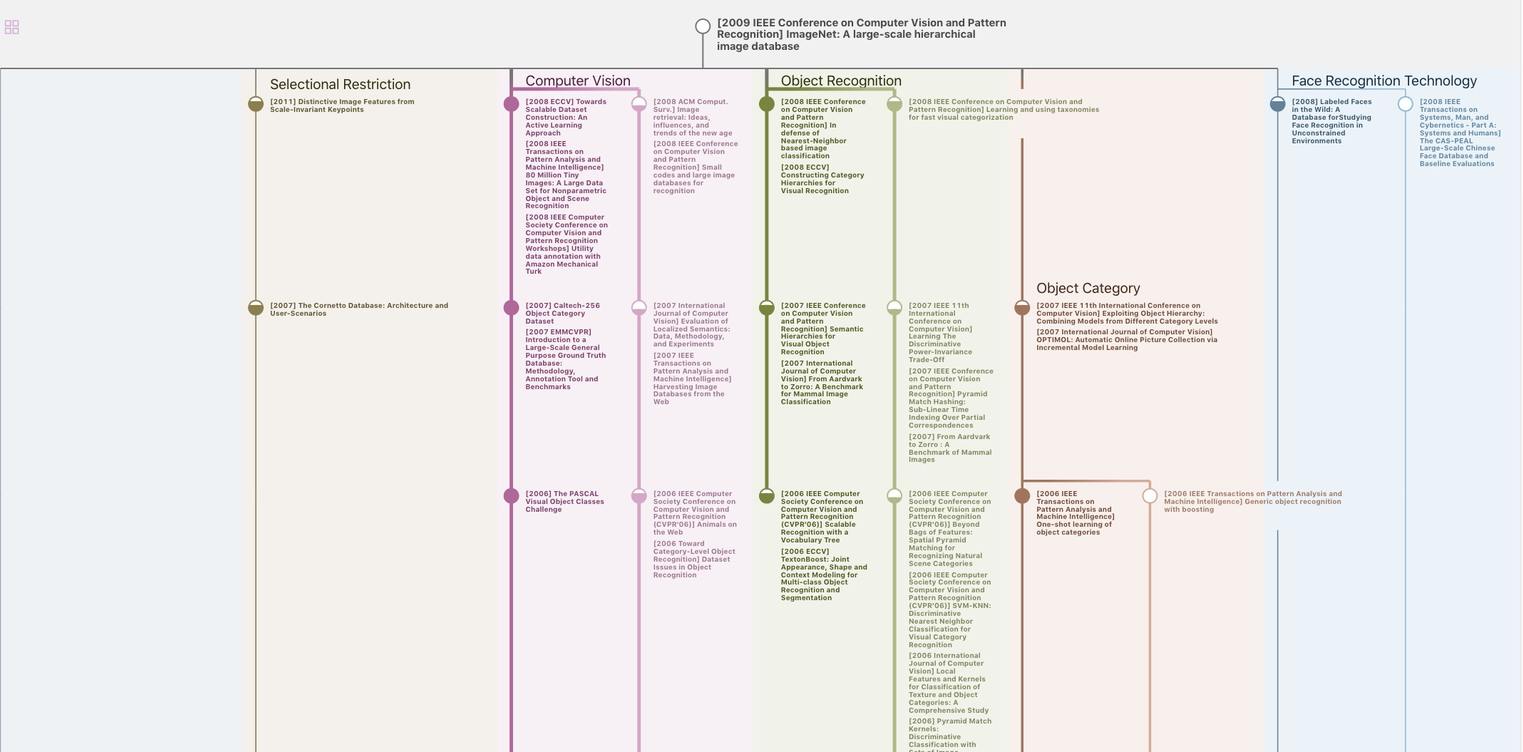
生成溯源树,研究论文发展脉络
Chat Paper
正在生成论文摘要