Towards a Data Privacy-Predictive Performance Trade-off
arxiv(2022)
摘要
Machine learning is increasingly used in the most diverse applications and domains, whether in healthcare, to predict pathologies, or in the financial sector to detect fraud. One of the linchpins for efficiency and accuracy in machine learning is data utility. However, when it contains personal information, full access may be restricted due to laws and regulations aiming to protect individuals' privacy. Therefore, data owners must ensure that any data shared guarantees such privacy. Removal or transformation of private information (de-identification) are among the most common techniques. Intuitively, one can anticipate that reducing detail or distorting information would result in losses for model predictive performance. However, previous work concerning classification tasks using de-identified data generally demonstrates that predictive performance can be preserved in specific applications. In this paper, we aim to evaluate the existence of a trade-off between data privacy and predictive performance in classification tasks. We leverage a large set of privacy-preserving techniques and learning algorithms to provide an assessment of re-identification ability and the impact of transformed variants on predictive performance. Unlike previous literature, we confirm that the higher the level of privacy (lower re-identification risk), the higher the impact on predictive performance, pointing towards clear evidence of a trade-off.
更多查看译文
关键词
Data privacy,Re-identification risk,Classification tasks,Predictive performance
AI 理解论文
溯源树
样例
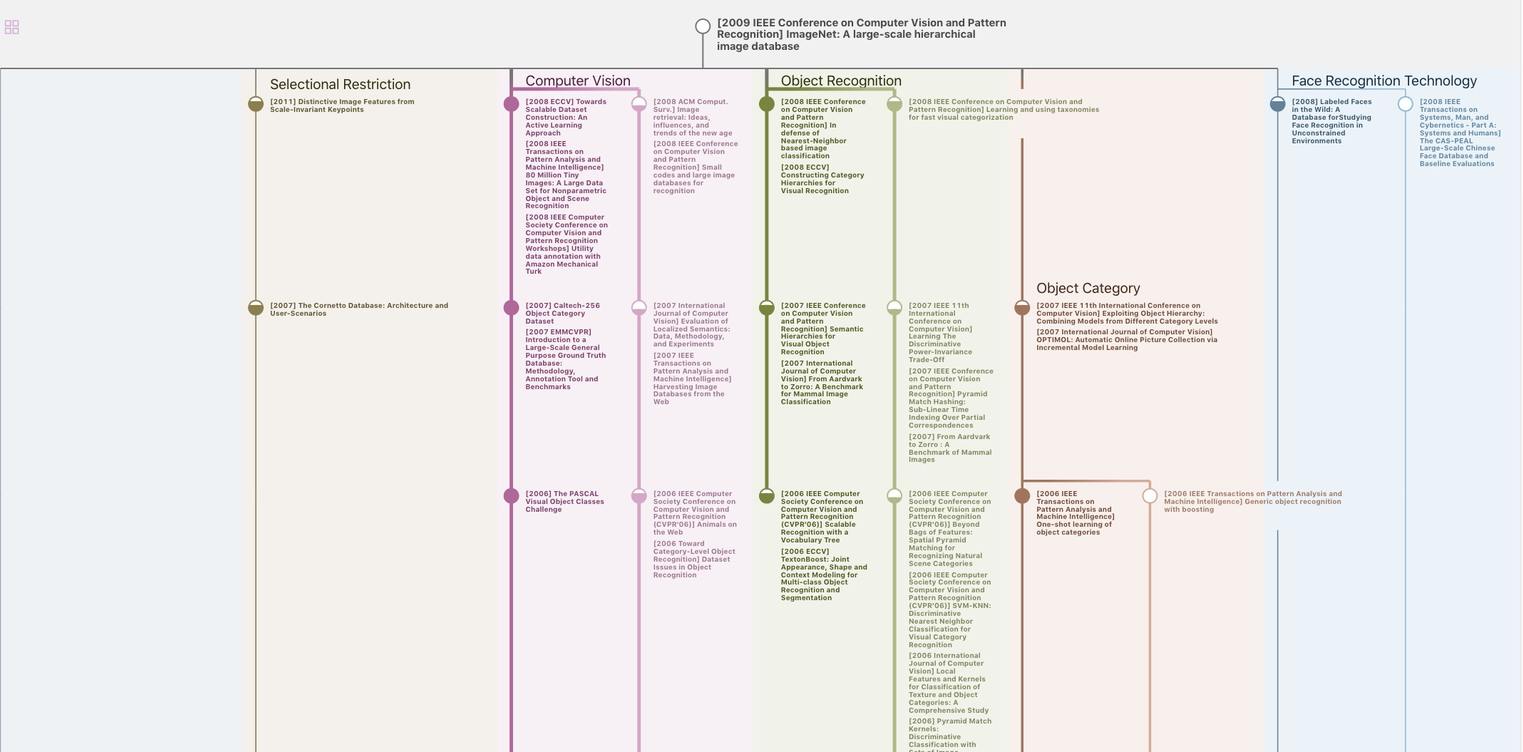
生成溯源树,研究论文发展脉络
Chat Paper
正在生成论文摘要