Robust Contrastive Learning against Noisy Views
IEEE Conference on Computer Vision and Pattern Recognition(2022)
摘要
Contrastive learning relies on an assumption that positive pairs contain related views that share certain underlying information about an instance, e.g., patches of an image or co-occurring multimodal signals of a video. What if this assumption is violated? The literature suggests that contrastive learning produces suboptimal representations in the presence of noisy views, e.g., false positive pairs with no apparent shared information. In this work, we pro-pose a new contrastive loss function that is robust against noisy views. We provide rigorous theoretical justifications by showing connections to robust symmetric losses for noisy binary classification and by establishing a new contrastive bound for mutual information maximization based on the Wasserstein distance measure. The proposed loss is completely modality-agnostic and a simple drop-in replacement for the InfoNCE loss, which makes it easy to apply to ex-isting contrastive frameworks. We show that our approach provides consistent improvements over the state-of-the-art on image, video, and graph contrastive learning bench-marks that exhibit a variety of real-world noise patterns.
更多查看译文
关键词
Representation learning, Machine learning
AI 理解论文
溯源树
样例
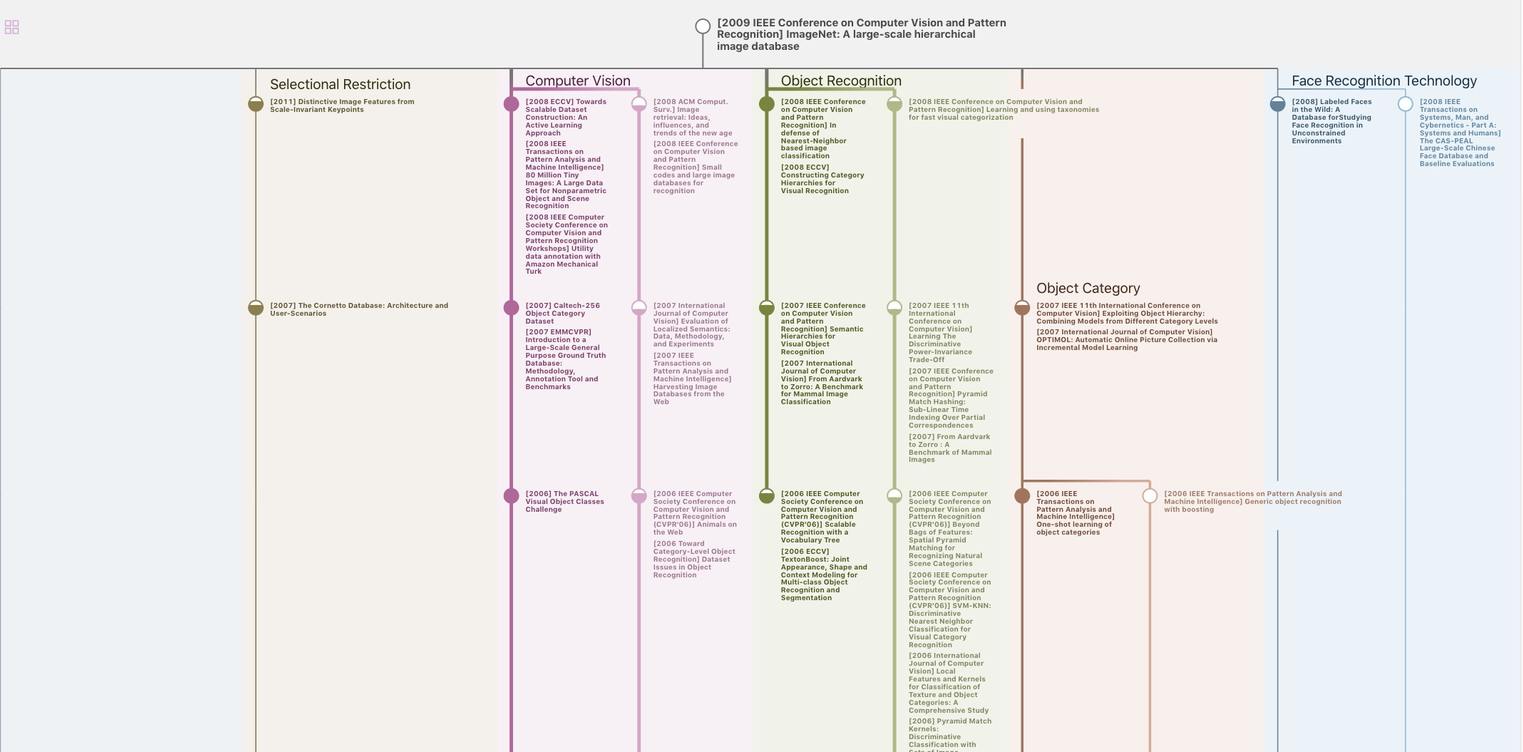
生成溯源树,研究论文发展脉络
Chat Paper
正在生成论文摘要