gDNA: Towards Generative Detailed Neural Avatars
IEEE Conference on Computer Vision and Pattern Recognition(2022)
Abstract
To make 3D human avatars widely available, we must be able to generate a variety of 3D virtual humans with varied identities and shapes in arbitrary poses. This task is chal-lenging due to the diversity of clothed body shapes, their complex articulations, and the resulting rich, yet stochas-tic geometric detail in clothing. Hence, current methods that represent 3D people do not provide a full generative model of people in clothing. In this paper, we propose a novel method that learns to generate detailed 3D shapes of people in a variety of garments with corresponding skin-ning weights. Specifically, we devise a multi-subject forward skinning module that is learned from only a few posed, unrigged scans per subject. To capture the stochastic nature of high-frequency details in garments, we leverage an adversarial loss formulation that encourages the model to capture the underlying statistics. We provide empirical evi-dence that this leads to realistic generation of local details such as wrinkles. We show that our model is able to gen-erate natural human avatars wearing diverse and detailed clothing. Furthermore, we show that our method can be used on the task of fitting human models to raw scans, out-performing the previous state-of-the-art.
MoreTranslated text
Key words
Face and gestures
AI Read Science
Must-Reading Tree
Example
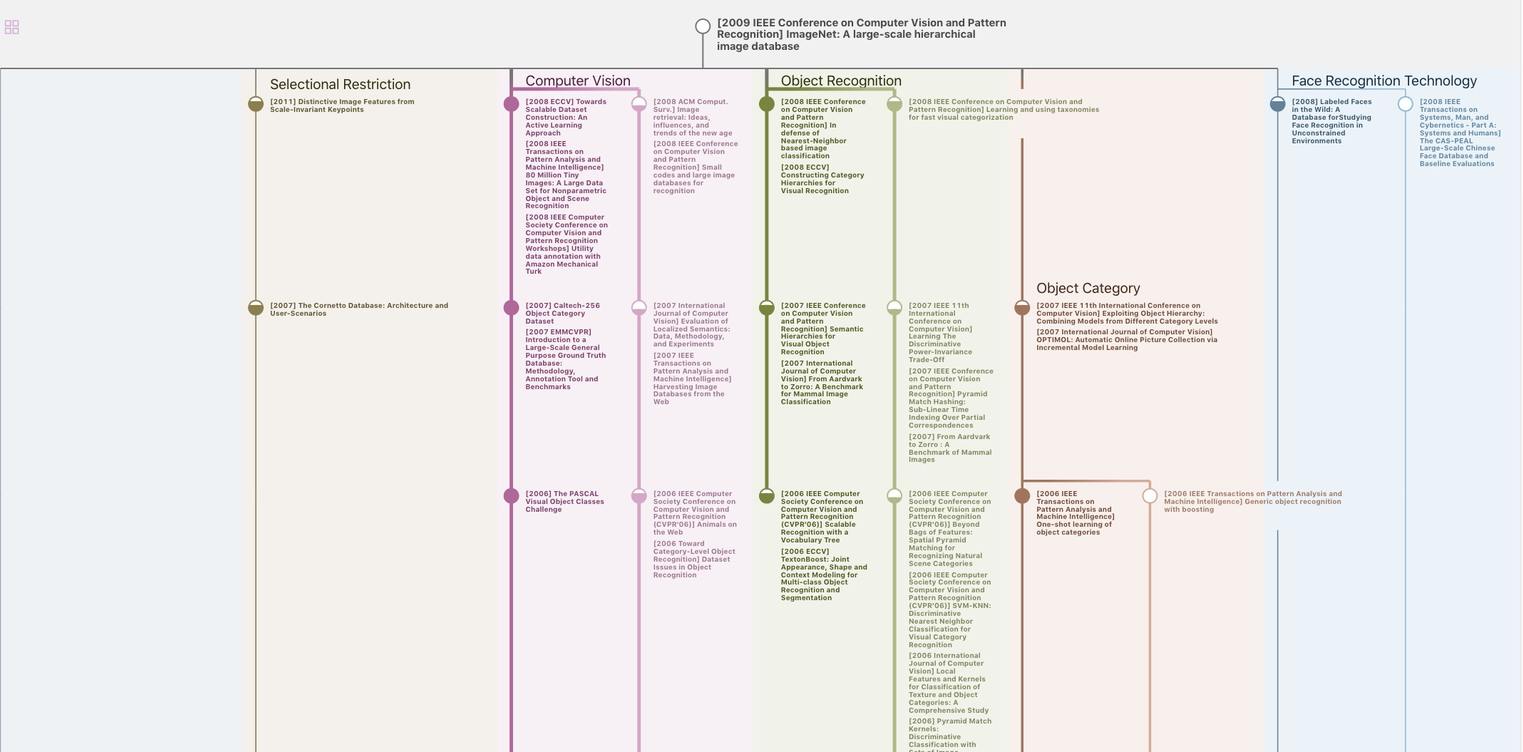
Generate MRT to find the research sequence of this paper
Chat Paper
Summary is being generated by the instructions you defined