Analysis of Multifocal Visual Evoked Potentials Using Artificial Intelligence Algorithms
TRANSLATIONAL VISION SCIENCE & TECHNOLOGY(2022)
摘要
Purpose: Clinical trials for remyelination in multiple sclerosis (MS) require an imaging biomarker. The multifocal visual evoked potential (mfVEP) is an accurate technique for measuring axonal conduction; however, it produces large datasets requiring lengthy analysis by human experts to detect measurable responses versus noisy traces. This study aimed to develop a machine-learning approach for the identification of true responses versus noisy traces and the detection of latency peaks in measurable signals. Methods: We obtained 2240 mfVEP traces from 10 MS patients using the VS-1 mfVEP machine, and they were classified by a skilled expert twice with an interval of 1 week. Of these, 2025 (90%) were classified consistently and used for the study. ResNet-50 and VGG16 models were trained and tested to produce three outputs: no signal, up-sloped signal, or down-sloped signal. Each model ran 1000 iterations with a stochastic gradient descent optimizer with a learning rate of 0.0001. Results: ResNet-50 and VGG16 had false-positive rates of 1.7% and 0.6%, respectively, when the testing dataset was analyzed (n=612). The false-negative rates were 8.2% and 6.5%, respectively, against the same dataset. The latency measurements in the validation and testing cohorts in the study were similar. Conclusions: Our models efficiently analyze mfVEPs with <2% false positives compared with human false positives of <8%. Translational Relevance: mfVEP, a safe neurophysiological technique, analyzed using artificial intelligence, can serve as an efficient biomarker in MS clinical trials and signal latency measurement.
更多查看译文
关键词
visual evoked potential, computational modeling, clinical trial, autoimmune response/disease, anti-inflammatory agents
AI 理解论文
溯源树
样例
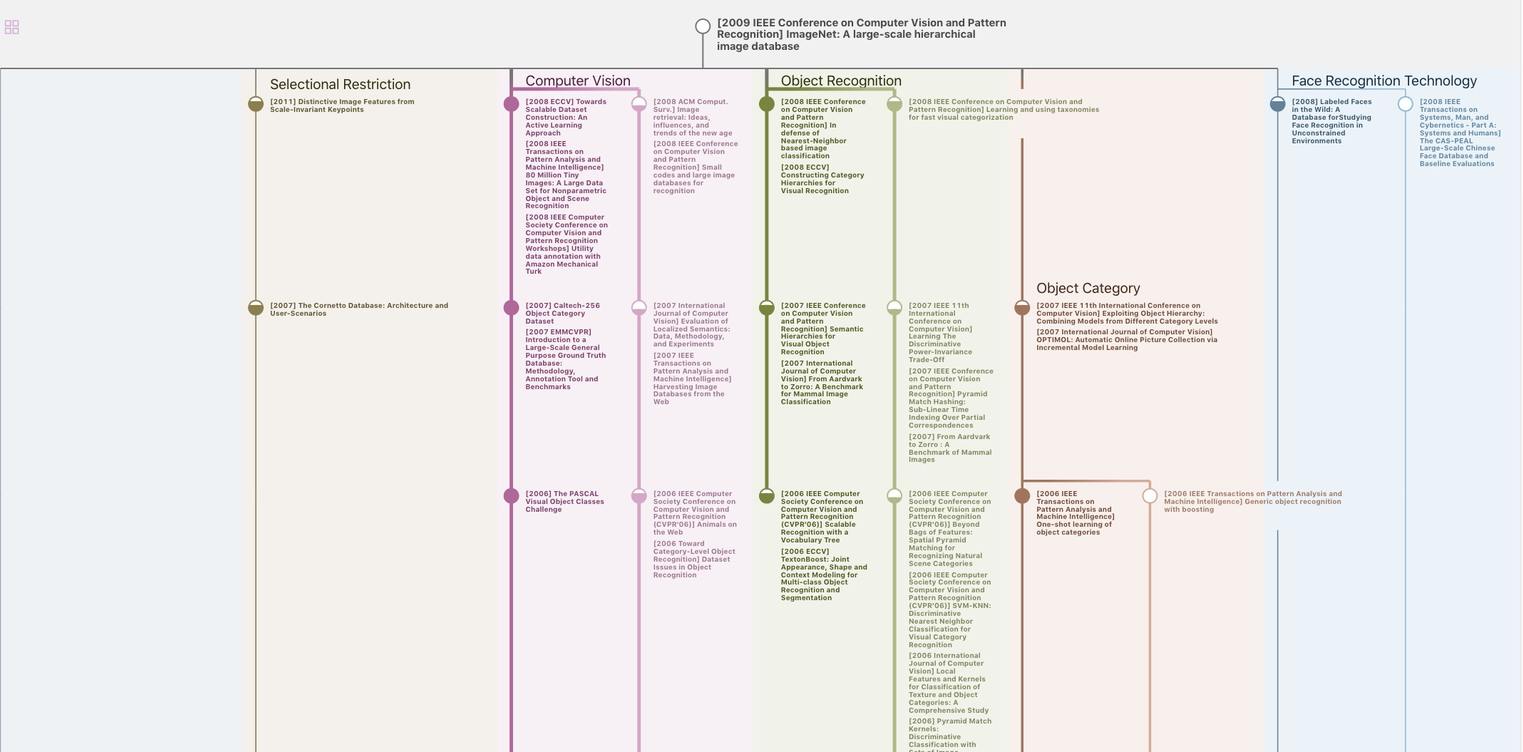
生成溯源树,研究论文发展脉络
Chat Paper
正在生成论文摘要