Towards the Next 1000 Languages in Multilingual Machine Translation: Exploring the Synergy Between Supervised and Self-Supervised Learning
arxiv(2022)
摘要
Achieving universal translation between all human language pairs is the holy-grail of machine translation (MT) research. While recent progress in massively multilingual MT is one step closer to reaching this goal, it is becoming evident that extending a multilingual MT system simply by training on more parallel data is unscalable, since the availability of labeled data for low-resource and non-English-centric language pairs is forbiddingly limited. To this end, we present a pragmatic approach towards building a multilingual MT model that covers hundreds of languages, using a mixture of supervised and self-supervised objectives, depending on the data availability for different language pairs. We demonstrate that the synergy between these two training paradigms enables the model to produce high-quality translations in the zero-resource setting, even surpassing supervised translation quality for low- and mid-resource languages. We conduct a wide array of experiments to understand the effect of the degree of multilingual supervision, domain mismatches and amounts of parallel and monolingual data on the quality of our self-supervised multilingual models. To demonstrate the scalability of the approach, we train models with over 200 languages and demonstrate high performance on zero-resource translation on several previously under-studied languages. We hope our findings will serve as a stepping stone towards enabling translation for the next thousand languages.
更多查看译文
关键词
multilingual machine translation
AI 理解论文
溯源树
样例
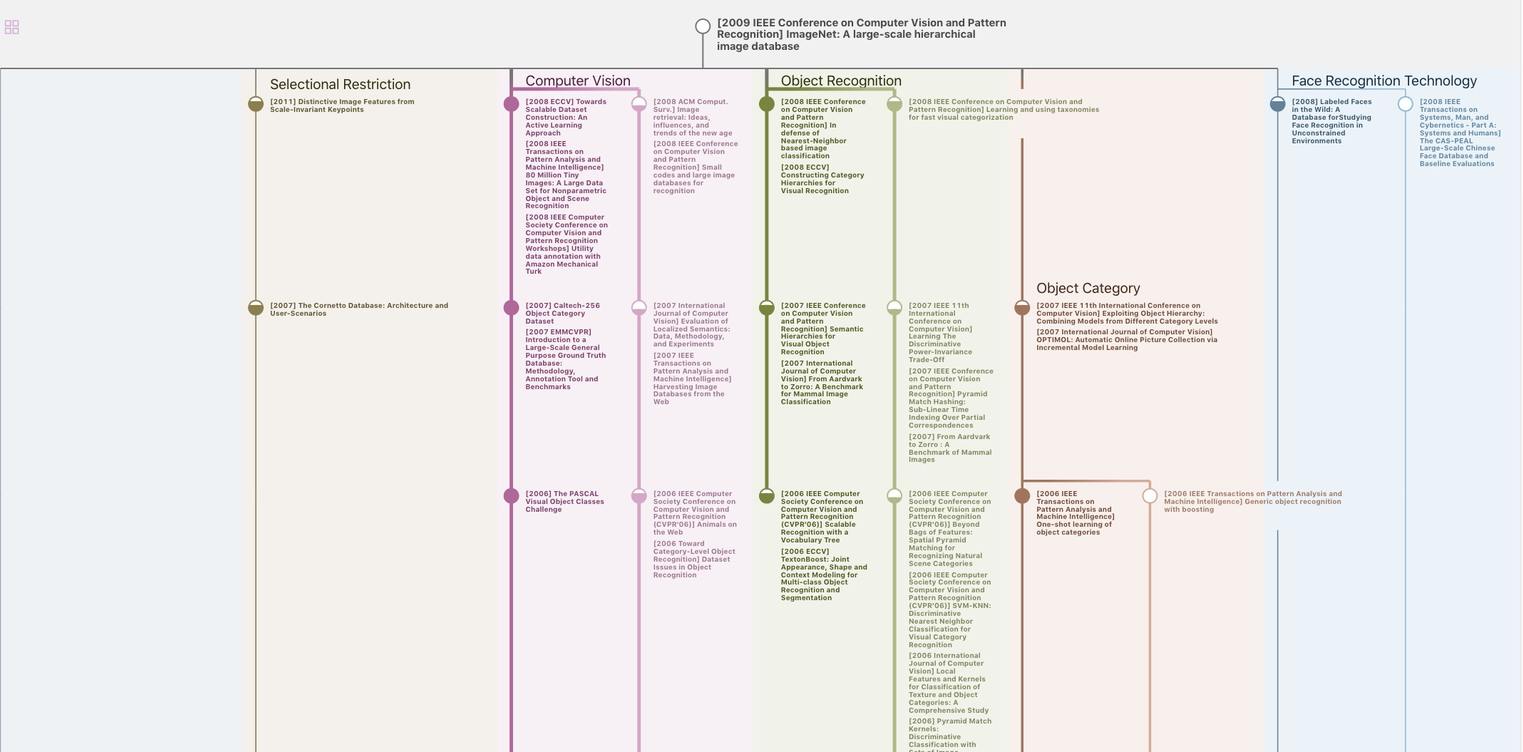
生成溯源树,研究论文发展脉络
Chat Paper
正在生成论文摘要