Sensitivity of estimated tacrolimus population pharmacokinetic profile to assumed dose timing and absorption in real-world data and simulated data
BRITISH JOURNAL OF CLINICAL PHARMACOLOGY(2022)
摘要
Aims Use of electronic health record (EHR) data to estimate population pharmacokinetic (PK) profiles necessitates several assumptions. We sought to investigate sensitivity to some of these assumptions about dose timing and absorption rates. Methods A population PK study with 363 subjects was performed using real-world data extracted from EHRs to estimate the tacrolimus population PK profile. Data were extracted and built using our automated system, EHR2PKPD, suitable for quickly constructing large PK datasets from the EHR. Population PK studies for oral medications performed using EHR data often assume a regular dosing schedule as prescribed without incorporating exact dosing time. We assessed the sensitivity of the PK parameter estimates to assumptions about dose timing using last-dose times extracted by our own natural language processing system, medExtractR. We also investigated the sensitivity of estimates to absorption rate constants that are often fixed at a published value in tacrolimus population PK analyses. We conducted simulation studies to investigate how drug PK profiles and experimental designs such as concentration measurements design affect sensitivity to incorrect assumptions about dose timing and absorption rates. Results There was no appreciable difference in parameter estimates with assumed versus extracted last-dose time, and our sensitivity analysis revealed little difference between parameters estimated across a range of assumed absorption rate constants. Conclusion Our findings suggest that drugs with a slower elimination rate (or a longer half-life) are less sensitive to dose timing errors and that experimental designs which only allow for trough blood concentrations are usually insensitive to deviation in absorption rate.
更多查看译文
关键词
absorption, biostatistics, therapeutic drug monitoring
AI 理解论文
溯源树
样例
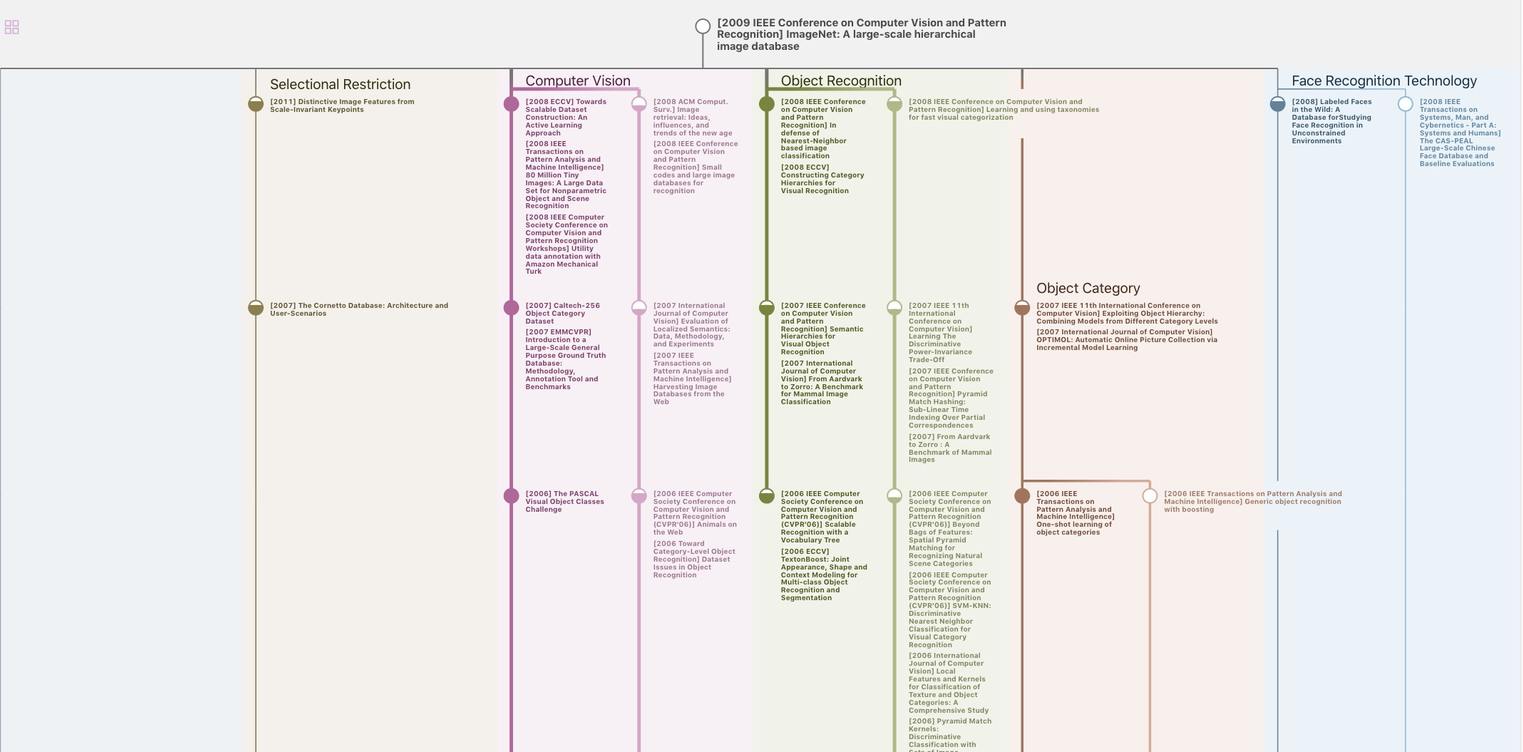
生成溯源树,研究论文发展脉络
Chat Paper
正在生成论文摘要