Deep structured neural networks for turbulence closure modeling
PHYSICS OF FLUIDS(2022)
摘要
Despite well-known limitations of Reynolds-averaged Navier-Stokes (RANS) simulations, this methodology remains the most widely used tool for predicting many turbulent flows due to computational efficiency. Machine learning is a promising approach to improve the accuracy of RANS simulations. One major area of improvement is using machine learning models to represent the complex relationship between the mean flow field gradients and the Reynolds stress tensor. In the present work, modifications to improve the stability of previous optimal eddy viscosity approaches for RANS simulations are presented and evaluated. The optimal eddy viscosity is reformulated with a non-negativity constraint, which promotes numerical stability. We demonstrate that the new formulation of the optimal eddy viscosity improves conditioning of RANS equations for a periodic hills test case. To demonstrate the suitability of this proportional/orthogonal tensor decomposition for use in a physics-informed data-driven turbulence closure, we use two neural networks (structured on this specific tensor decomposition, which is incorporated as an inductive bias into the network design) to predict the newly reformulated linear and non-linear parts of the Reynolds stress tensor. Injecting these network model predictions for the Reynolds stresses into RANS simulation improves predictions of the velocity field, even when compared to a sophisticated (state of the art) physics-based turbulence closure model. Finally, we apply shapley additive explanations values to obtain insights from the learned representation for inner workings of the neural network used to predict the optimal eddy viscosity from the input feature data. Published under an exclusive license by AIP Publishing.
更多查看译文
关键词
turbulence,structured neural networks,neural networks,closure
AI 理解论文
溯源树
样例
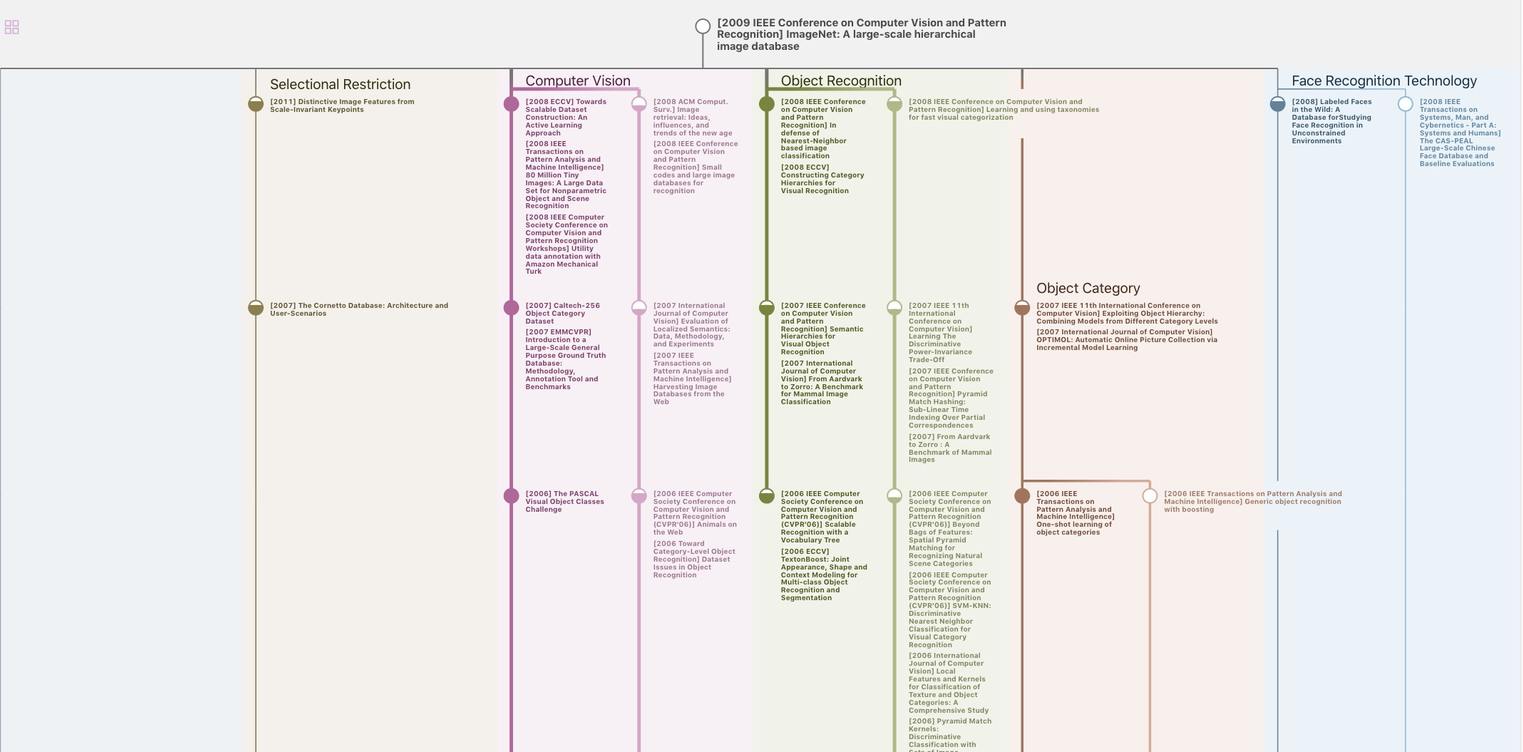
生成溯源树,研究论文发展脉络
Chat Paper
正在生成论文摘要