Offsetting Unequal Competition Through RL-Assisted Incentive Schemes
IEEE Transactions on Computational Social Systems(2023)
摘要
This article investigates the dynamics of competition among organizations with unequal expertise. Multiagent reinforcement learning (MARL) has been used to simulate and understand the impact of various incentive schemes designed to offset such inequality. We design Touch-Mark, a game based on well-known multiagent particle environment, where two teams (weak and strong) with unequal but changing skill levels compete against each other. For training such a game, we propose a novel controller-assisted MARL algorithm C-MADDPG, which empowers each agent with an ensemble of policies along with a supervised controller that by selectively partitioning the sample space and triggers intelligent role division among the teammates. Using C-MADDPG as an underlying framework, we propose an incentive scheme for the weak team such that the final rewards of both teams become the same. We find that despite the incentive, the final reward of the weak team falls short of the strong team. On inspecting, we realize that an overall incentive scheme for the weak team does not incentivize the weaker agents within that team to learn and improve. To offset this, we now specially incentivize the weaker player to learn and, as a result, observe that the weak team beyond an initial phase performs at par with the stronger team. The final goal of this article has been to formulate a dynamic incentive scheme that continuously balances the reward of the two teams. This is achieved by devising an incentive scheme enriched with an RL agent, which takes minimum information from the environment.
更多查看译文
关键词
Adaptive systems,artificial intelligence,collaborative intelligence computational and artificial intelligence,cybernetics,machine learning,man and cybernetics,multi-agent systems,reinforcement learning system,systems engineering and theory
AI 理解论文
溯源树
样例
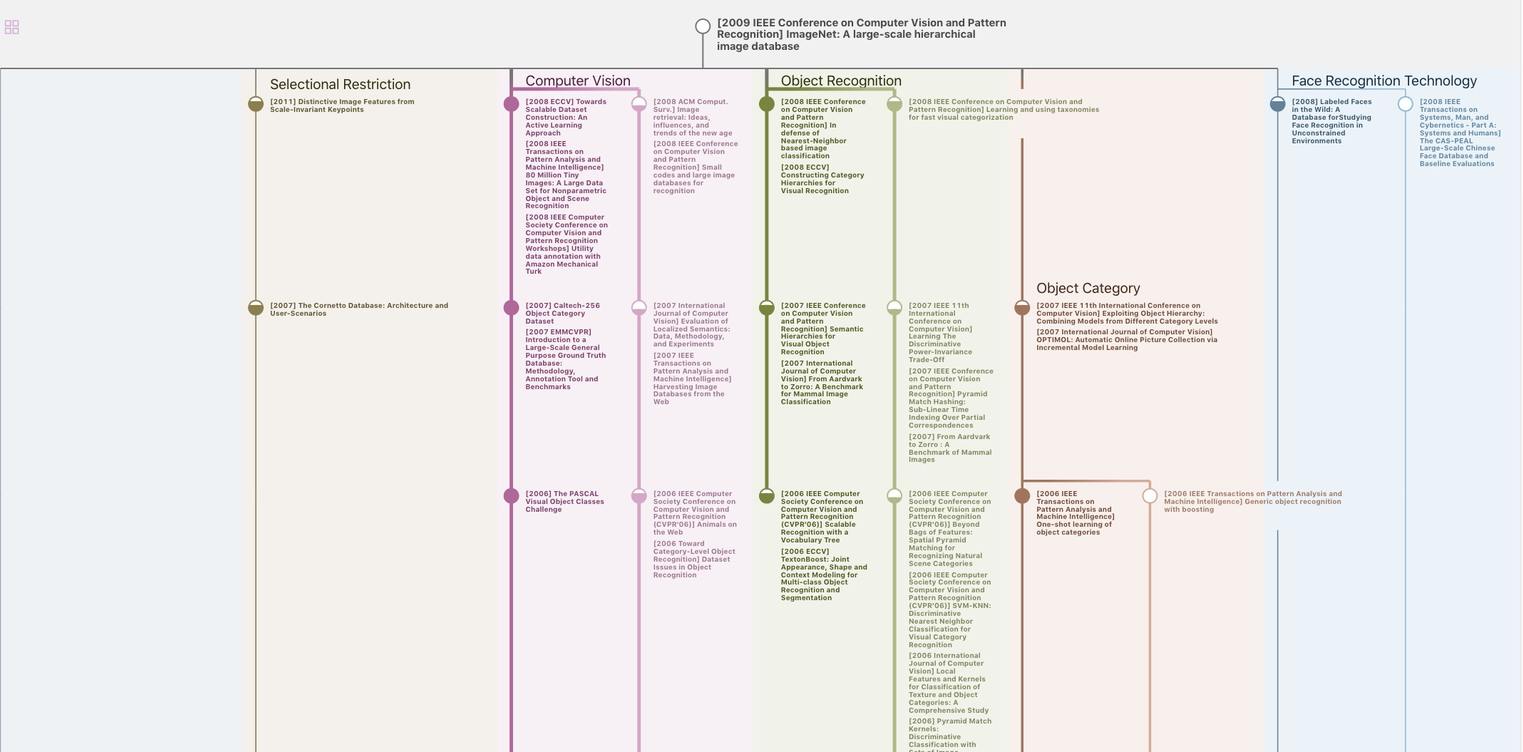
生成溯源树,研究论文发展脉络
Chat Paper
正在生成论文摘要