Swift and Sure: Hardness-aware Contrastive Learning for Low-dimensional Knowledge Graph Embeddings
International World Wide Web Conference(2022)
摘要
ABSTRACT Knowledge graph embedding (KGE) has shown great potential in automatic knowledge graph (KG) completion and knowledge-driven tasks. However, recent KGE models suffer from high training cost and large storage space, thus limiting their practicality in real-world applications. To address this challenge, based on the latest findings in the field of Contrastive Learning, we propose a novel KGE training framework called Hardness-aware Low-dimensional Embedding (HaLE). Instead of the traditional Negative Sampling, we design a new loss function based on query sampling that can balance two important training targets, Alignment and Uniformity. Furthermore, we analyze the hardness-aware ability of recent low-dimensional hyperbolic models and propose a lightweight hardness-aware activation mechanism, which can help the KGE models focus on hard instances and speed up convergence. The experimental results show that in the limited training time, HaLE can effectively improve the performance and training speed of KGE models on five commonly-used datasets. After training just a few minutes, the HaLE-trained models are competitive compared to the state-of-the-art models in both low- and high-dimensional conditions.
更多查看译文
关键词
Knowledge Graph Embedding, Contrastive Learning, Link Prediction, Knowledge Graph
AI 理解论文
溯源树
样例
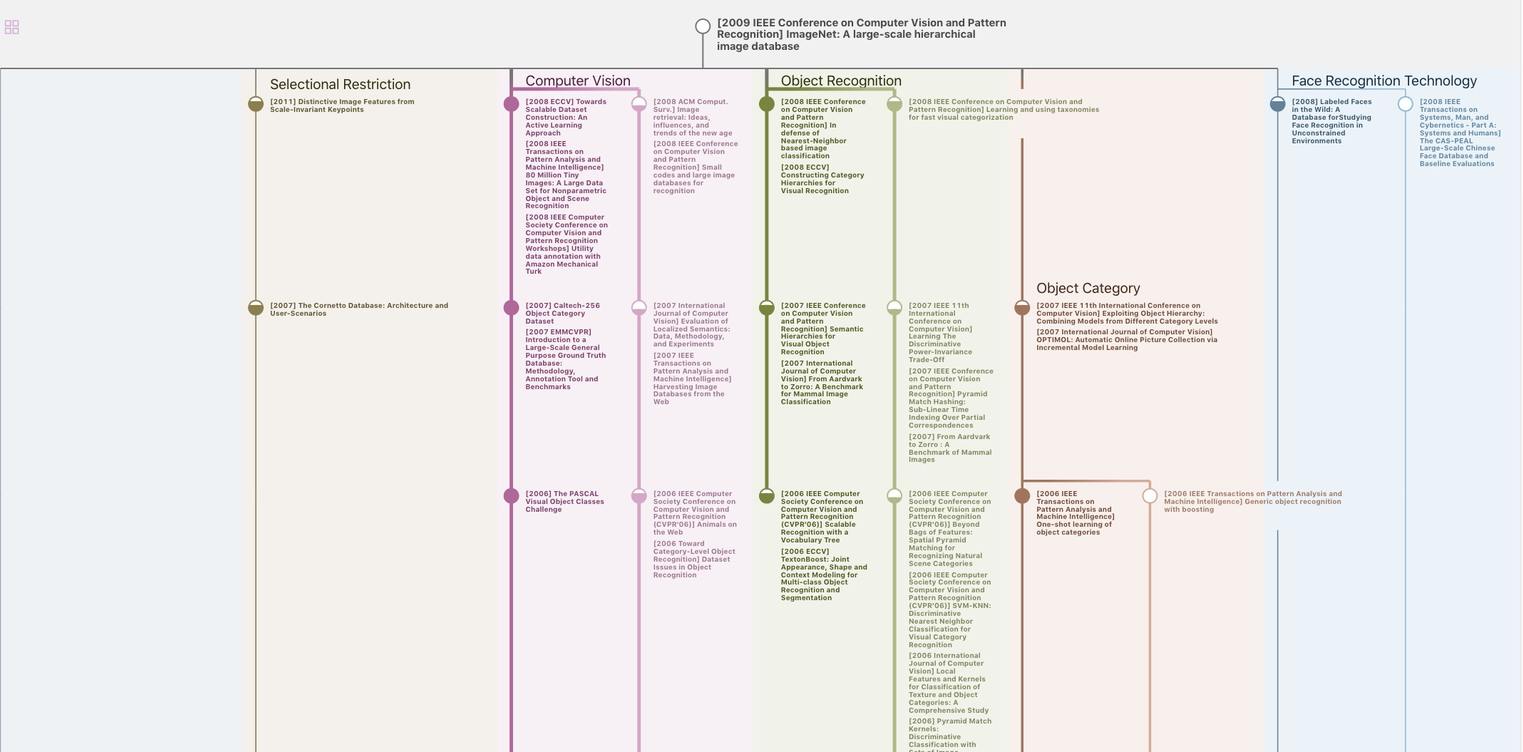
生成溯源树,研究论文发展脉络
Chat Paper
正在生成论文摘要