A Probabilistic Approach to Surgical Tasks and Skill Metrics
IEEE Transactions on Biomedical Engineering(2022)
摘要
Identifying and quantifying the activities that compose surgery is essential for effective interventions, computer-aided analyses and the advancement of surgical data science. For example, recent studies have shown that objective metrics (referred to as objective performance indicators, OPIs) computed during key surgical tasks correlate with surgeon skill and clinical outcomes. Unambiguous identification of these surgical tasks can be particularly challenging for both human annotators and algorithms. Each surgical procedure has multiple approaches, each surgeon has their own level of skill, and the initiation and termination of surgical tasks can be subject to interpretation. As such, human annotators and machine learning models face the same basic problem, accurately identifying the boundaries of surgical tasks despite variable and unstructured information. For use in surgeon feedback, OPIs should also be robust to the variability and diversity in this data. To mitigate this difficulty, we propose a probabilistic approach to surgical task identification and calculation of OPIs. Rather than relying on tasks that are identified by hard temporal boundaries, we demonstrate an approach that relies on distributions of start and stop times, for a probabilistic interpretation of when the task was performed. We first use hypothetical data to outline how this approach is superior to other conventional approaches. Then we present similar analyses on surgical data. We find that when surgical tasks are identified by their individual probabilities, the resulting OPIs are less sensitive to noise in the identification of the start and stop times. These results suggest that this probabilistic approach holds promise for the future of surgical data science.
更多查看译文
关键词
Benchmarking,Clinical Competence,Feedback,Humans,Machine Learning,Surgeons
AI 理解论文
溯源树
样例
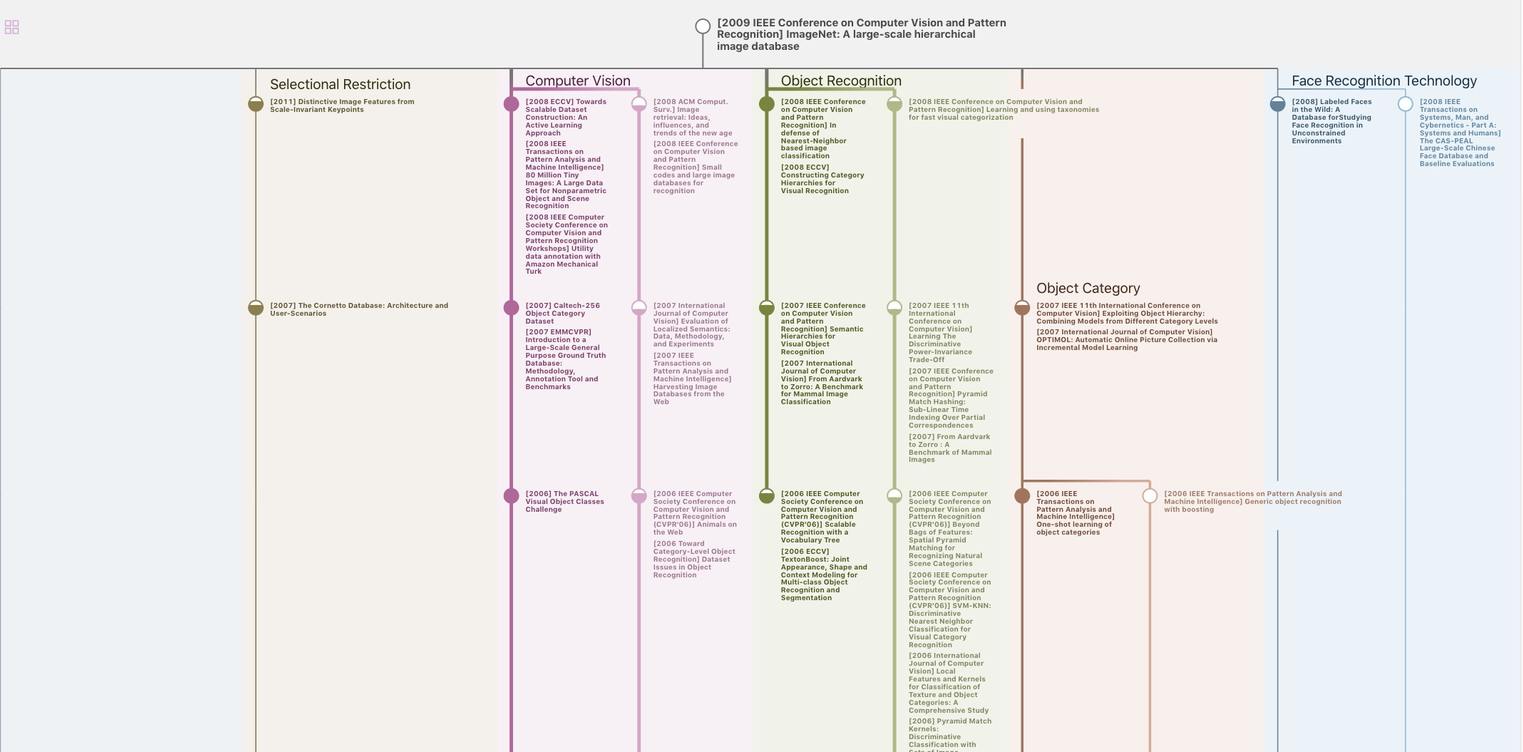
生成溯源树,研究论文发展脉络
Chat Paper
正在生成论文摘要