Empirical robustification of pre-trained classifiers
semanticscholar(2021)
摘要
Most pre-trained classifiers, though they may work extremely well on the domain they were trained upon, are not trained in a robust fashion, and therefore are susceptible to adversarial attacks. A recent technique, denoised-smoothing, demonstrated that it was possible to create certifiably robust classifiers from a pre-trained classifier (without any retraining) by pre-pending a denoising network and wrapping the entire pipeline within randomized smoothing. However, this is a costly procedure, which requires multiple queries due to the randomized smoothing element, and which ultimately is very dependent on the quality of the denoiser. In this paper, we demonstrate that a more conventional adversarial training approach also works when applied to this robustification process. Specifically, we show that by training an image-to-image translation model, prepended to a pre-trained classifier, with losses that optimize for both the fidelity of the image reconstruction and the adversarial performance of the end-to-end system, we can robustify pre-trained classifiers to a higher empirical degree of accuracy than denoised smoothing, while being more efficient at inference time. Furthermore, these robustifers are also transferable to some degree across multiple classifiers and even some architectures, illustrating that in some real sense they are removing the “adversarial manifold” from the input data, a task that has traditionally been very challenging for “conventional” preprocessing methods. This work is sponsored by DARPA grant HR11002020006 Bosch Center for Artificial Intelligence, Pittsburgh, USA Carnegie-Mellon University, Pittsburgh, USA. Correspondence to: Mohammad Norouzzadeh . Accepted by the ICML 2021 workshop on A Blessing in Disguise: The Prospects and Perils of Adversarial Machine Learning. Copyright 2021 by the author(s).
更多查看译文
AI 理解论文
溯源树
样例
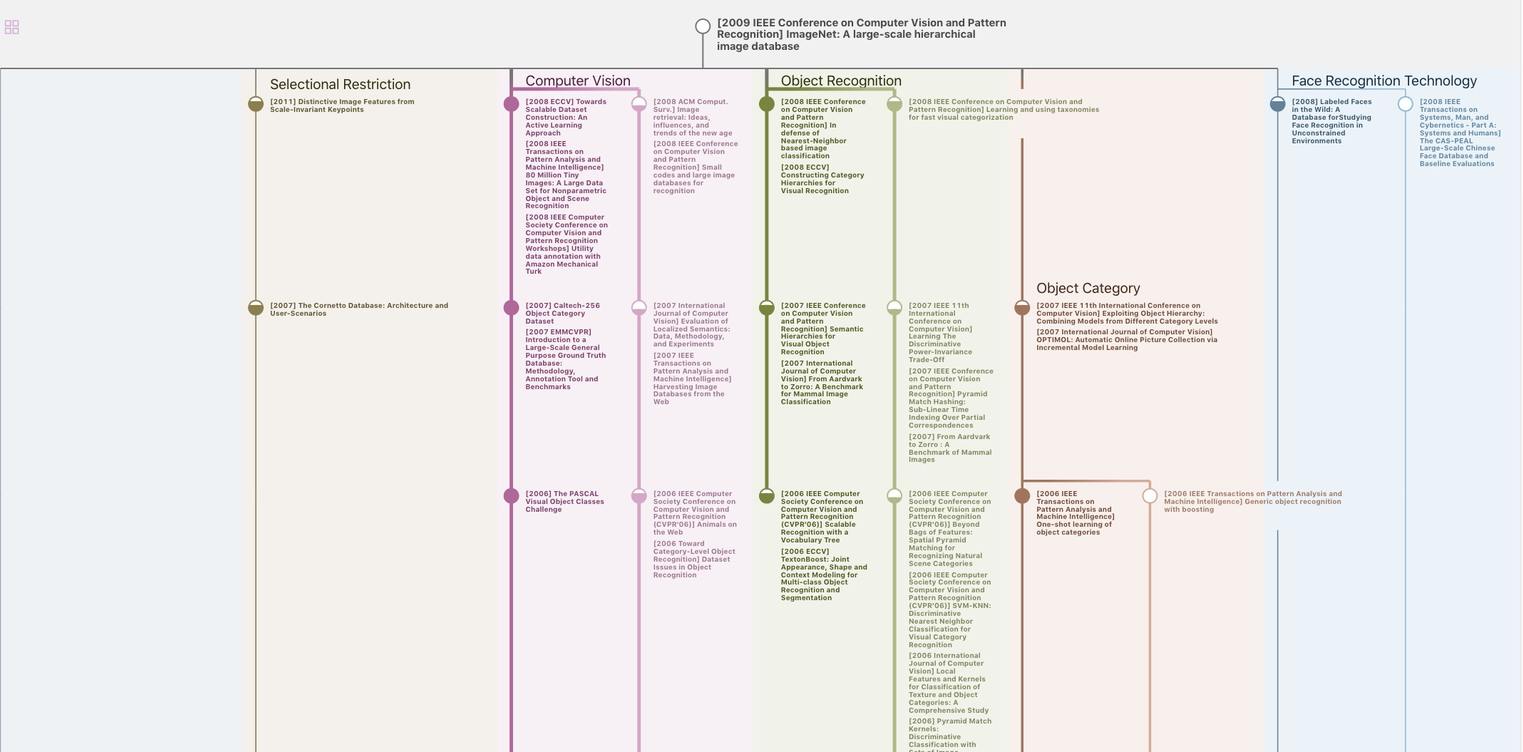
生成溯源树,研究论文发展脉络
Chat Paper
正在生成论文摘要