Modelling time series of count data using hidden markov models
semanticscholar(2020)
摘要
Poisson distribution is normally used to model count data. However, in practical situations, the equidispersion feature of Poisson tends to go wrong. In such cases, mixture models are usually used to fit the data. But serial dependence in the observations does not agree with the mixture models and hence, they assume independence. The Hidden Markov Model (HMM) comes in handy in such a scenario as it handles both overdispersion (variance greater than mean) and serial dependence in the data. HMM is an extension of Markov models and it can be considered as a generalization of mixture model. In this study, we generate two sets of count data (i) independent and overdispersed and (ii) serially-dependent and overdispersed and conduct a simulation study for proving the performance of HMM. For the study, the Poisson-Hidden Markov (P-HMM) Model is applied to a real data on the monthly cases of Hepatitis-B in the state of Kerala in South India, which is overdispersed and serially dependent. Viterbi algorithm is used to obtain the best estimate of the state sequence. By applying Akaike information criterion (AIC) and Bayesian information criterion (BIC) model selection criteria, we could find that the prevalence of Hepatitis-B can be modelled by 5-state P-HMM.
更多查看译文
AI 理解论文
溯源树
样例
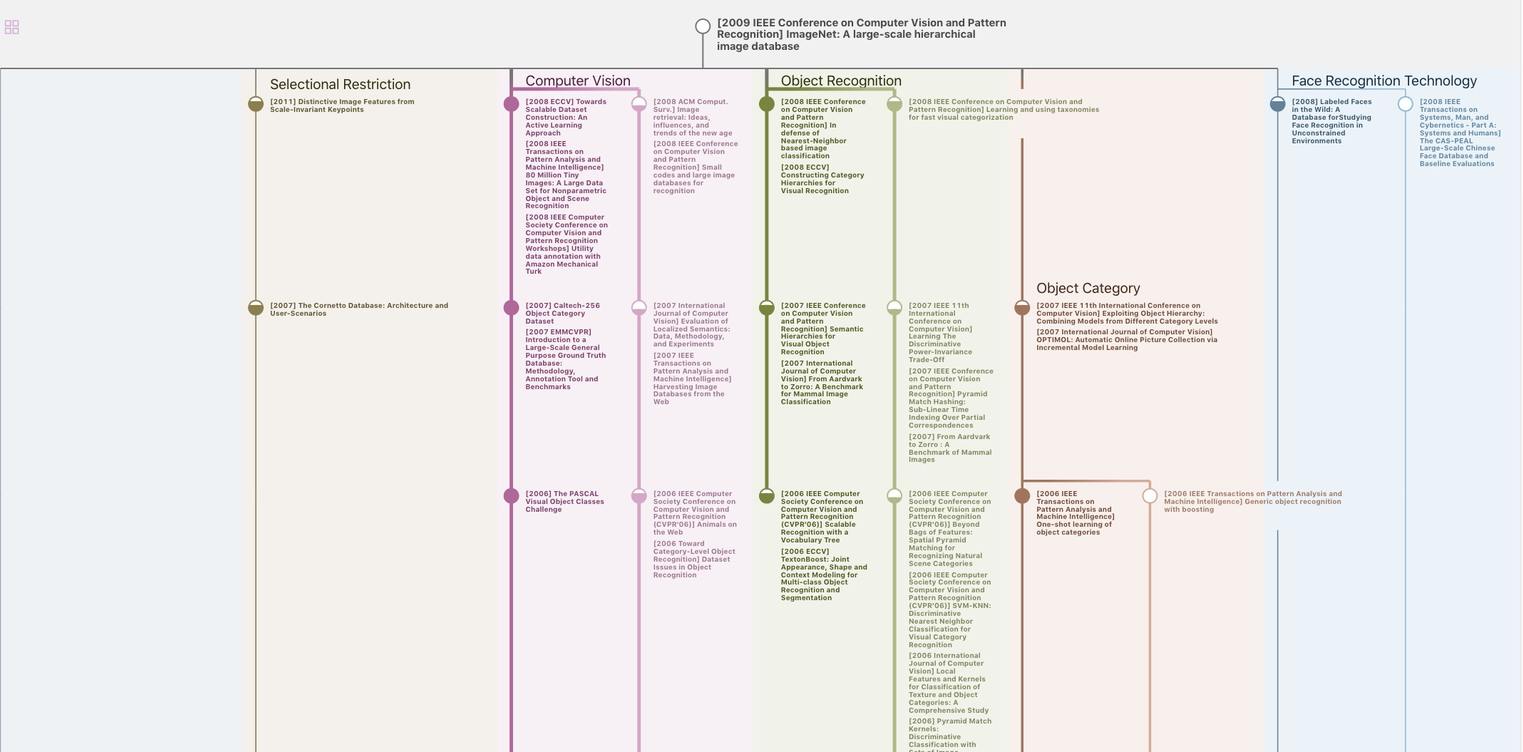
生成溯源树,研究论文发展脉络
Chat Paper
正在生成论文摘要