Design of Experiments in mixed continuous and dis- crete space
semanticscholar(2021)
摘要
Design of experiments (DoE) are used in various contexts such as optimization or uncertainty quantification based on a time-consuming numerical simulator. It aims to select a limited number of values to assign to the simulator input variables that give a maximal knowledge on the simulator outputs of interest. One motivating application is the optimal design of turbine blades in an helicopter engine [1], which takes as inputs mixed continuous and binary variables. In this study, we propose two new methods for space-filling designs in mixed continuous and discrete space defined as D = {z = (x, y) ∈ R × I}, (1) where x ∈ R, y ∈ I are the continuous and discrete variables, respectively, and I denotes the discrete space (e.g. integer, binary or categorical variables). Although the literature on space-filling DoEs is vast in the continuous case, we focus here on a particular setting relying on kernel-embedding of probability distributions, since it can be more easily generalized to the mixed variables case, as we will see below. In this framework, several previous works reformulated the space-filling DoE problem as the minimization of the maximum mean discrepancy (MMD) between an empirical measure (corresponding to the DoE) and a target distribution. For two probability distributions P and Q and a Reproducing Kernel Hilbert Space (RKHS) H with positive definite kernel k, the MMD distance between P and Q is defined as MMD(P,Q) = ‖μP − μQ‖H (2) where μP = ∫ k(x, .)dP(x) and μQ = ∫ k(x, .)dQ(x) are the kernel embeddings of P and Q, respectively, and serve as representations of the probability distributions. The RKHS framework makes it possible to write the distance with only expectations of kernel functions: MMD(P,Q) = Eξ,ξ′∼Pk(ξ, ξ′) + Eζ,ζ′∼Qk(ζ, ζ ′)− 2Eξ∼P,ζ∼Qk(ξ, ζ). Coming back to our DoE setting, when P is an empirical measure 1 n ∑n i=1 δsi of n points si ∈ [a, b] in a hypercube and the target measure Q is the uniform distribution on [a, b], the squared MMD actually writes as well-known discrepancy measures, depending on the choice of the kernel k [2]. But the choice of the target Q is not restricted, and the case where it is also given as an empirical measure 1 N ∑N i=1 δti with N typically much larger than n is of particular interest. Indeed this case corresponds to applications where one is given a large sample from a probability distribution and the goal is to find a small subset of samples which best represents the underlying unknown distribution [3]. The problem then writes as the optimization problem min s1,s2,...,sn MMD( 1 n n ∑
更多查看译文
AI 理解论文
溯源树
样例
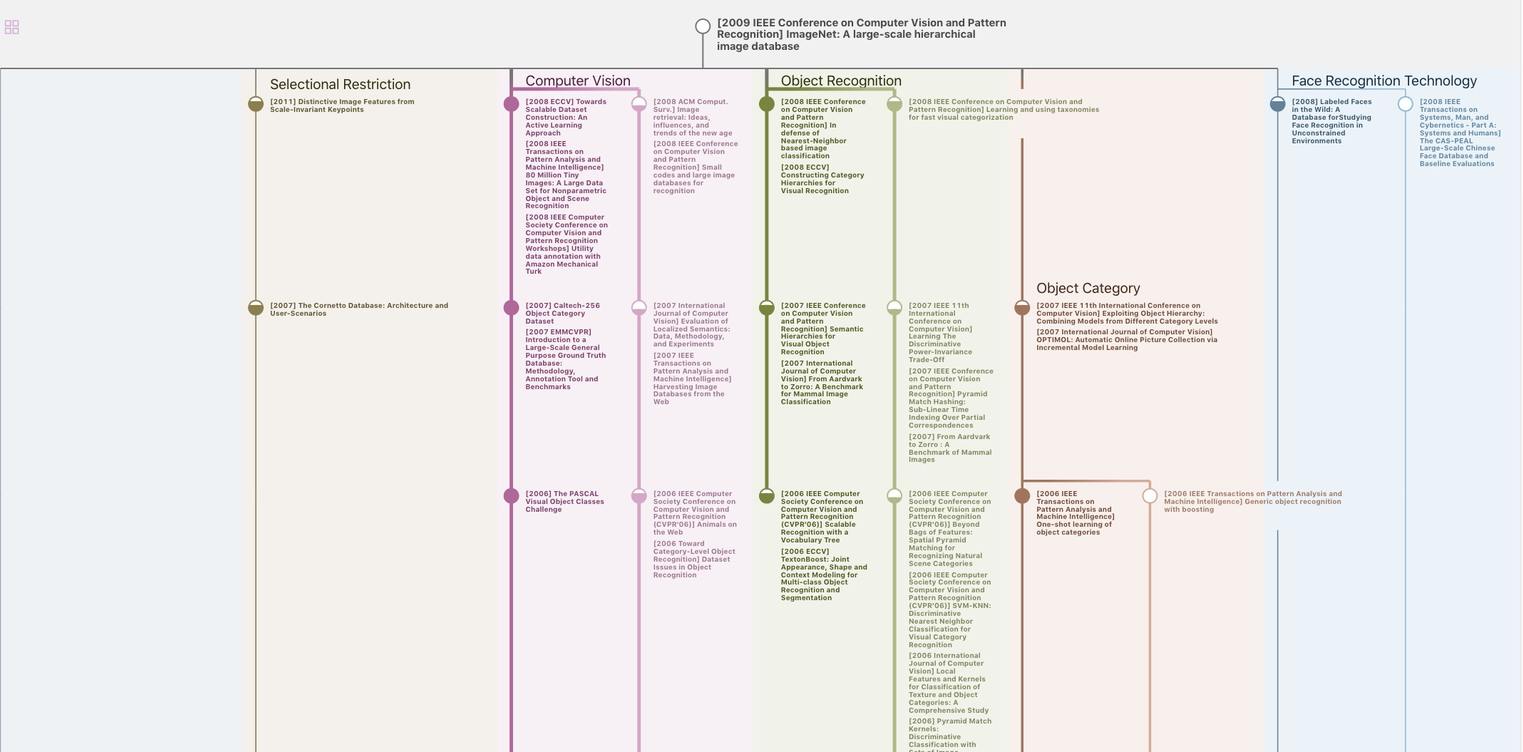
生成溯源树,研究论文发展脉络
Chat Paper
正在生成论文摘要