Predicting physiologic response to changes in positive end-expiratory pressure in mechanically ventilated children: a computable phenotype and machine learning approach
semanticscholar(2020)
摘要
Background Positive end-expiratory pressure (PEEP) is often increased to improve ventilation efficiency and gas exchange during pediatric mechanical ventilation. Although it is clinically important to optimize PEEP in this population, there is a paucity of literature to guide the clinician at the bedside. Increasingly, time-series physiologic data are available for mechanically ventilated subjects in the intensive care unit. However, these data have not been adequately explored in the literature. Therefore, we sought to apply time-series computable phenotyping on time-series physiologic data and develop a model to predict PEEP response in mechanically ventilated children. Methods We conducted a retrospective analysis of continuous data in a academic hospital multidisciplinary intensive care unit. Patients were elgible for inclusion in the study if they received mechanically ventilation for > 25 hours and were < 18 years of age. Time-series data from the patient monitor and mechanical ventilator were abstracted 1-hour preceding and 1-hour following a PEEP change. PEEP increase (PEEPincrease), a responderwas defined as anyone who exhibited an improved dead-space fraction (Vd/Vt); non-respondersdemonstrated a worsening Vd/Vtin the hour following the PEEP change. Features from continuous mechanical ventilation variables were extracted and used to train a support vector machine model in order to predict Vd/Vt response to changes in PEEP. The performance of the model was assessed by calculating the area under the receiver operator characteristic curve (AUROC) and computing measures of diagnostic accuracy. Results In all, 393 PEEP change cases were included in the analysis in 83 subjects. A total of 27 computable phenotypes were identified and incorporated into the model. The AUROC was 0.82 and 0.90 for classifying response to PEEP increases and decreases respectively. The overall diagnostic accuracy was 0.75 for PEEP increases and 0.84 for PEEP decreases. Conclusions The model classified responders to increases and decreases in PEEP with reasonable accuracy. The model performed better for those cases when PEEP was decreases. In the future, these methods may play an important role in optimizing care of the mechanically ventilated pediatric patients, especially if they can be tailored to individual institutions.
更多查看译文
关键词
physiologic response,computable phenotype,machine learning,end-expiratory
AI 理解论文
溯源树
样例
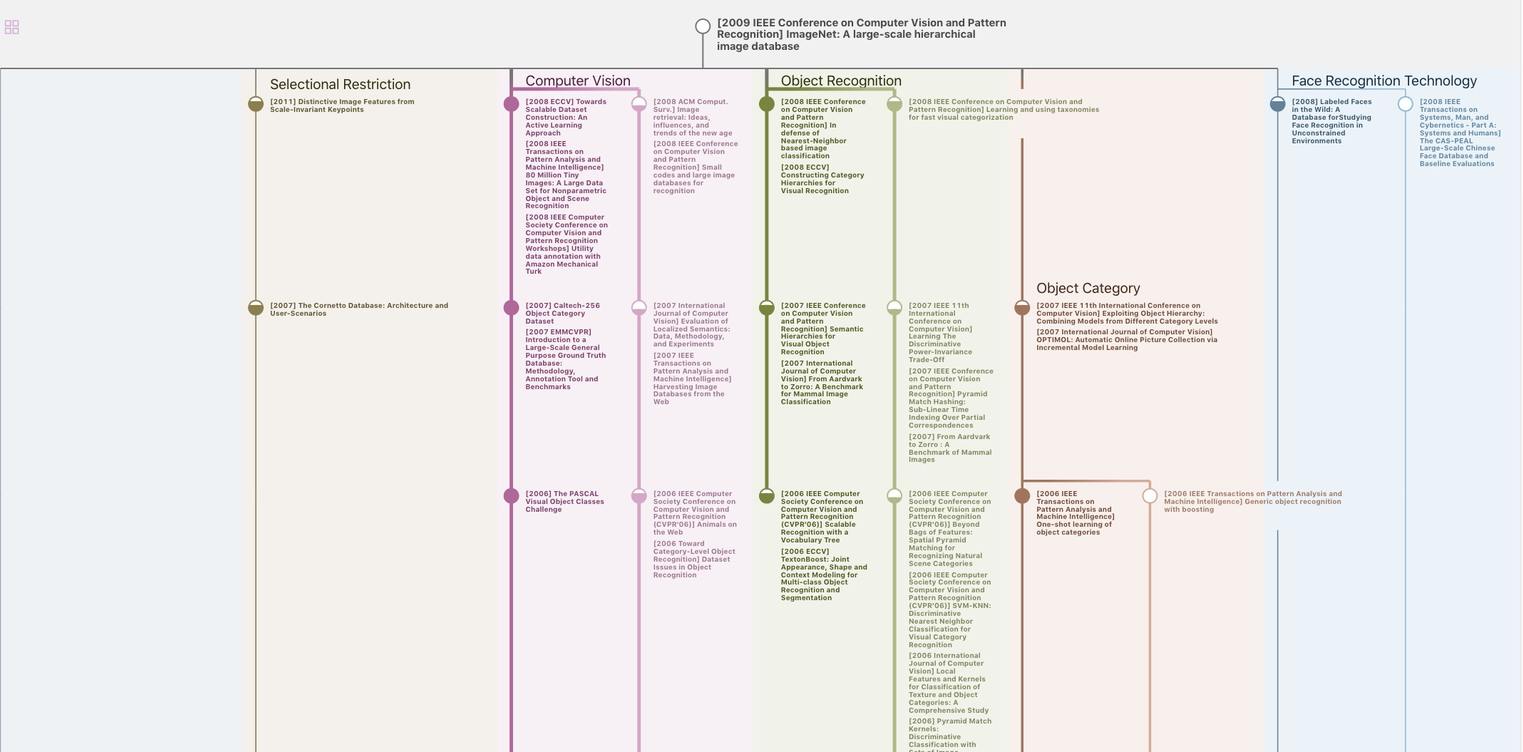
生成溯源树,研究论文发展脉络
Chat Paper
正在生成论文摘要