Applying Deep Learning to NASA MODIS
semanticscholar(2020)
摘要
Marine Low Cloud Mesoscale Morphology 3 Tianle Yuan1,2, Hua Song3, Robert Wood4, Johannes Mohrmann4, Kerry Meyer1, Lazaros 4 Oreopoulos1, Steven Platnick1 5 1Earth Science Directorate, NASA Goddard Space Flight Center 6 2Joint Center for Earth Systems Technology, University of Maryland, Baltimore County 7 3Science Systems and Applications, Inc. 8 4Department of Atmospheric Sciences, University of Washington 9 10 Correspondence: tianle.yuan@nasa.gov 11 12 Abstract: 13 14 Marine low clouds display rich mesoscale morphological types, distinct spatial patterns of cloud 15 fields. Being able to differentiate low cloud morphology offers a tool for the research 16 community to go one step beyond bulk cloud statistics such as cloud fraction and advance the 17 understanding of low clouds. Here we report the progress of our project that aims to create an 18 observational record of low cloud mesoscale morphology at a near-global (60S-60N) scale. First, 19 a training set is created by our team members manually labeling thousands of mesoscale 20 (128x128) MODIS scenes into six different categories: stratus, closed cellular convection, 21 disorganized convection, open cellular convection, clustered cumulus convection, and 22 suppressed cumulus convection. Then we train a deep convolutional neural network model 23 using this training set to classify individual MODIS scenes at 128x128 resolution, and test it on a 24 test set. The trained model achieves a cross-type average precision of about 93%. We apply the 25 trained model to 16 years of data over the Southeast Pacific. The resulting climatological 26 distribution of low cloud morphology types shows both expected and unexpected features and 27 suggests promising potential for low cloud studies as a data product. 28 29
更多查看译文
AI 理解论文
溯源树
样例
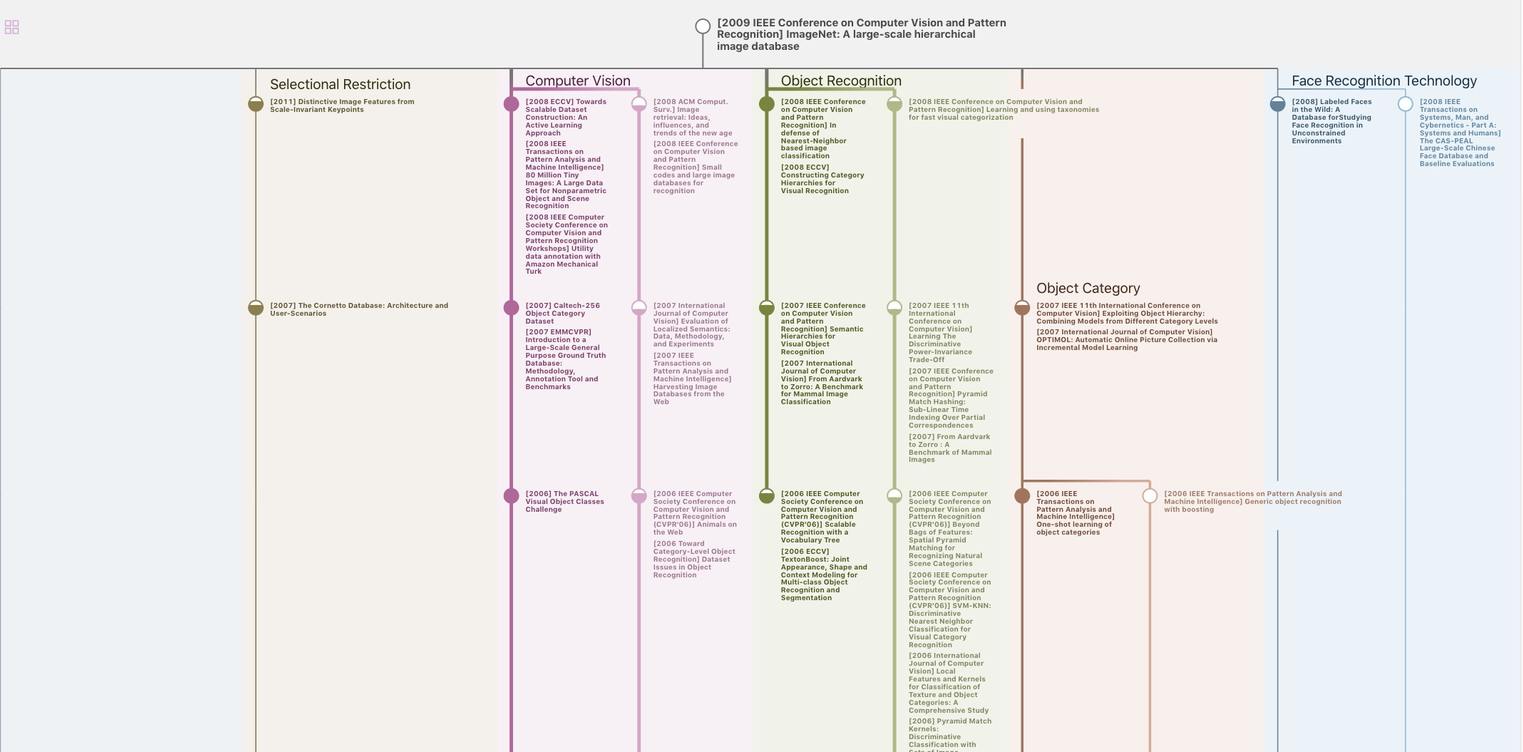
生成溯源树,研究论文发展脉络
Chat Paper
正在生成论文摘要