A General Framework for the Disintegration of PAC-Bayesian Bounds
semanticscholar(2021)
摘要
PAC-Bayesian bounds are known to be tight and informative when studying the generalization ability of randomized classifiers. However, when applied to some family of deterministic models such as neural networks, they require a loose and costly derandomization step. As an alternative to this step, we introduce new PAC-Bayesian generalization bounds that have the originality to provide disintegrated bounds, i.e., they give guarantees over one single hypothesis instead of the usual averaged analysis. Our bounds are easily optimizable and can be used to design learning algorithms. We illustrate the interest of our result on neural networks and show a significant practical improvement over the state-of-the-art framework. Introduction PAC-Bayesian theory (Shawe-Taylor and Williamson, 1997; McAllester, 1998) provides a powerful framework for analyzing the generalization ability of machine learning models such as linear classifiers (Germain et al., 2009), SVM (Ambroladze et al., 2006) or neural networks (Dziugaite and Roy, 2017). PAC-Bayesian analyses usually take the form of bounds on the average risk of a randomized classifier with respect to a learned posterior distribution given a chosen prior distribution defined over a set of hypotheses. While such bounds are very effective for analyzing stochastic classifiers, some machine learning methods need nevertheless guarantees on deterministic models. In this case, a derandomization step of the bound is required. Different forms of derandomization have been introduced in the literature for specific settings. Among them, Langford and Shawe-Taylor (2002) propose a derandomization for Gaussian posteriors over linear classifiers: thanks to the Gaussian symmetry, a bound on the risk of the maximum a posteriori (deterministic) classifier is obtainable from the bound on the average risk of the randomized classifier. Also relying on Gaussian posteriors, Letarte et al. (2019) derived a PAC-Bayes bound for a very specific deterministic network architecture using sign functions as activations. Another line of works derandomizes neural networks (Neyshabur et al., 2018; Nagarajan and Kolter, 2019a). While being technically different, it starts from PAC-Bayesian guarantees on the randomized classifier and use an “output perturbation” bound to convert a guarantee from a random classifier to the mean classifier. These works highlight the need of a general framework for the derandomization of classic PAC-Bayesian bounds. In this paper, we focus on another kind of derandomization, sometimes referred to as disintegration of the PAC-Bayesian bound, and first proposed by Catoni (2007, Th.1.2.7) and Blanchard and Fleuret (2007): Instead of bounding the average risk of a randomized classifier with respect to the posterior distribution, the desintegrated PACBayesian bounds upper-bound the risk of a sampled (unique) classifier from the posterior distribution. Despite their interest in derandomizing PAC-Bayesian bounds, this kind of bounds have only received little study in the literature; especially we can cite the recent work of Rivasplata et al. (2020, Th.1(i)) who derived a general disintegrated PAC-Bayesian theorem. Moreover, these bounds have never been used in practice. Driven by machine learning practical purposes, our objective is twofold: To derive new tight disintegrated PACBayesian bounds (i) that directly derandomize any type of classifiers without any other additional step and with (almost) no impact on the guarantee, (ii) that can be easily optimized to learn classifiers with strong guarantees. Our main contribution has a practical objective: Providing a new general framework based on the Rényi divergence allowing efficient learning. We also derive an information-theoretic bound giving interesting new insights on disintegration procedures. Note that for the sake of readability we deferred to the technical appendix the proofs of our theoretical results.
更多查看译文
关键词
bounds,disintegration,pac-bayesian
AI 理解论文
溯源树
样例
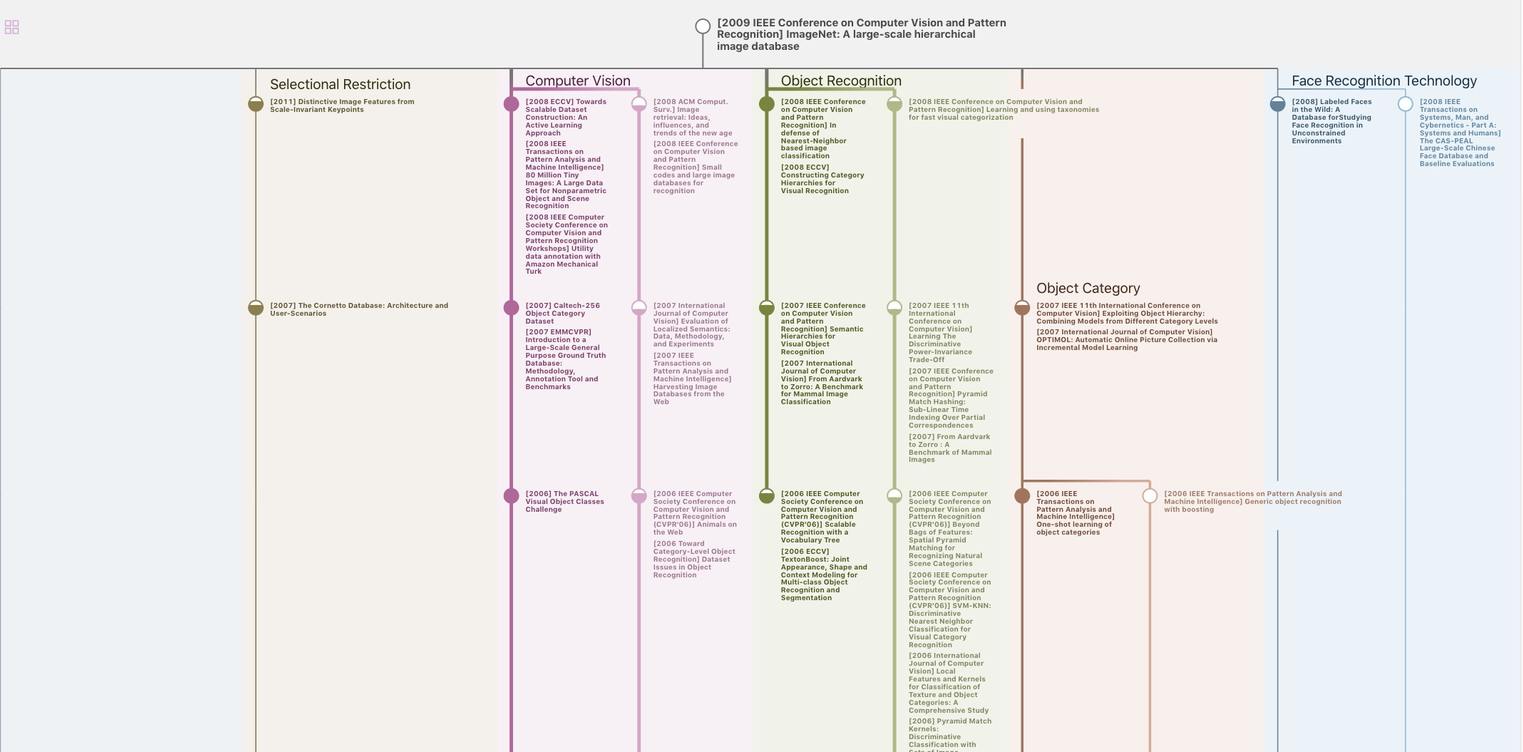
生成溯源树,研究论文发展脉络
Chat Paper
正在生成论文摘要