Bilevel optimization for hyperparameter tuning in structured sparse models
semanticscholar(2020)
摘要
Context A whole variety of sparse estimators have been developed over the last decades. In particular it is possible to finely model brain responses using refined sparse linear models. Such models take into account the spatial aspects as well as the temporal aspects of the brain responses [4]. These estimators are based on the resolution of nonsmooth optimization problems, which can now be solved in a very efficient way, e.g., using block coordinate descent techniques [5]. However, these estimators rely on hyperparameters, namely, regularization parameters in this context. Setting regularization parameters for sparse estimators is notoriously difficult, though crucial in practice: the quality of the estimator can dramatically varies for different values of these regularization parameters. This calibration challenge can prevent practitioners from using these refined models in practice. For this internship, the motivation is to develop tools based on differential programming [1] to automatically set such hyperparameters for time-frequency models [4], and provide practitioners with automatically calibrated method for brain signals analysis. If one denotes Y ∈ Rn×q the data (EEG and MEG), X ∈ Rn×p the design matrix, Φ ∈ Cq×m a time-frequency transform (Short-time Fourier Transform), B ∈ Rp×q the unknown the brain activity and its time-frequency transform Z = BΦ ∈ Cp×m the problem reads:
更多查看译文
AI 理解论文
溯源树
样例
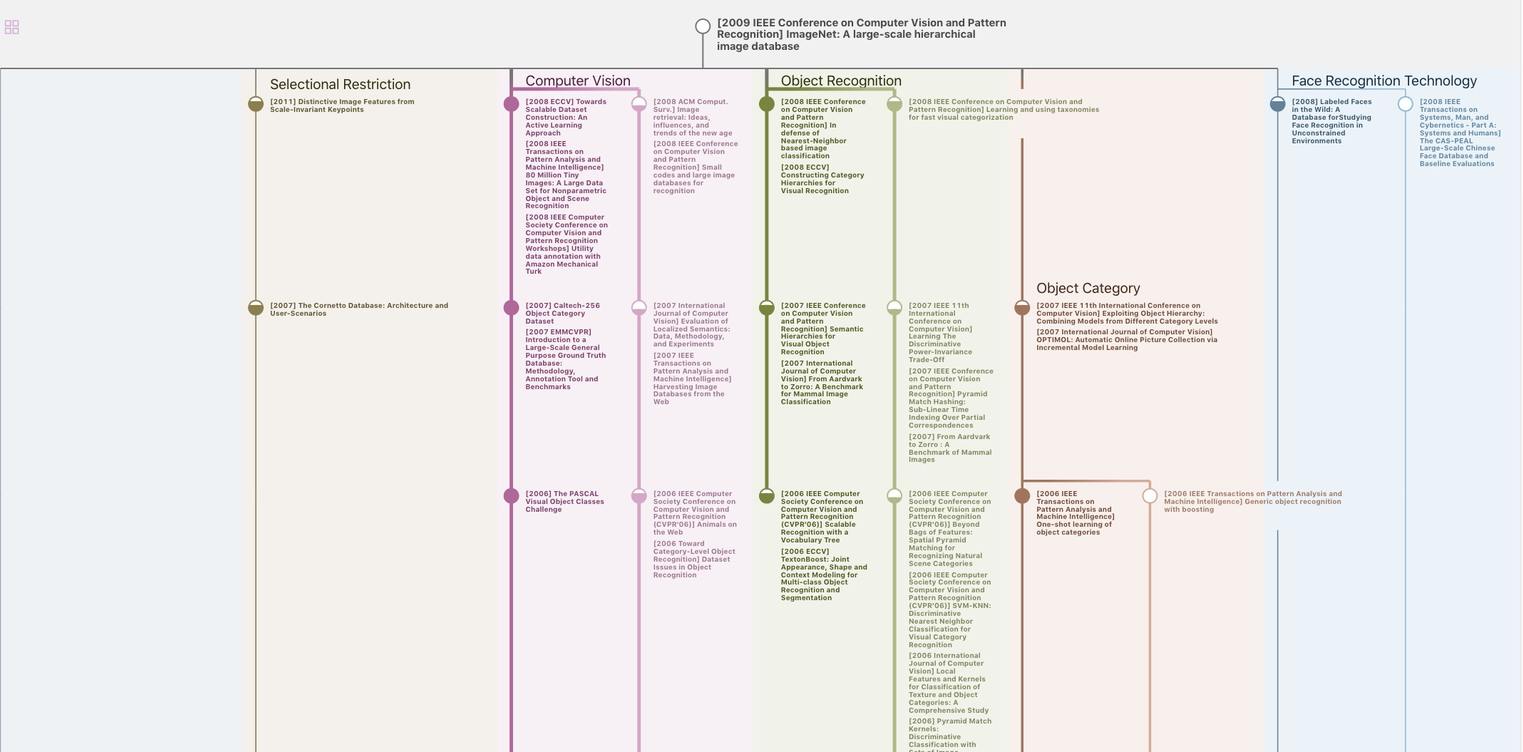
生成溯源树,研究论文发展脉络
Chat Paper
正在生成论文摘要