Improved digital image-based assessment of soil aggregate size by applying convolutional neural networks
COMPUTERS AND ELECTRONICS IN AGRICULTURE(2021)
摘要
Assessing and control of soil aggregate size during tillage operations are important to guarantee a proper seedbed preparation and in consequence a high crop yield, as well as to achieve a high resource use efficiency. In this research, a convolutional neural network (CNN) called SoilNet is proposed to classify images of nine different soil aggregate sizes taken from three different heights of 60, 80 and 100 cm (the higher the height, the lower the images' resolution). SoilNet combines a residual architecture with attention modules. Specifically, convolutional block attention modules are added after a series of convolution layers to highlight important local regions and extract more discriminative features. Moreover, features from multiple layers are combined by means of hypercolumns to increase predictive performance. In addition, fine-tuning of three pre-trained CNNs, VGG16, ResNet-50 and MobileNetV2 were applied. Based on the results, the performance of the pre-trained CNNs decreased with decreasing the image resolution increasing the photography height. The best mean overall classification accuracies (MCAs) obtained by the pre-trained CNNs were 96.97, 96.15 and 93.81%, for the photography heights of 60, 80 and 100 cm, respectively. However, the SoilNet showed a more satisfactory performance for classifying the images captured at greater heights with lower resolutions. The MCAs obtained by the network for the imaging heights of 60, 80 and 100 cm were 97.34, 97.06 and 97.13%, respectively. The SoilNet improved the overall classification accuracies obtained by applying the classical image processing on the same datasets in a previous study, up to 25.30, 29.18 and 30.46%, at imaging heights of 60, 80 and 100 cm, respectively.
更多查看译文
关键词
Precision agriculture,Site-specific cultivation,Soil tilth sensing,Digital imaging,Deep learning
AI 理解论文
溯源树
样例
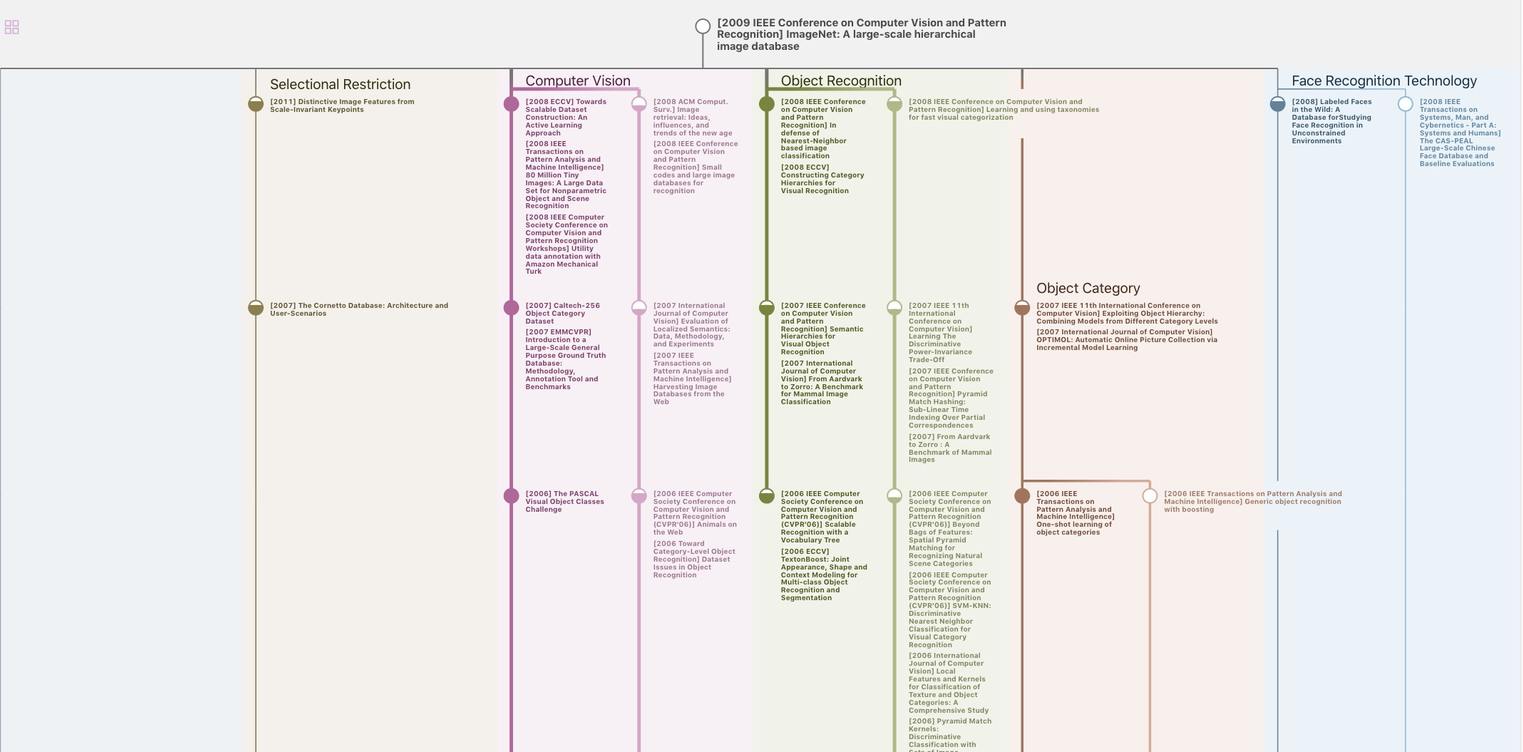
生成溯源树,研究论文发展脉络
Chat Paper
正在生成论文摘要