Effective and Inconspicuous Over-the-Air Adversarial Examples with Adaptive Filtering.
IEEE International Conference on Acoustics, Speech, and Signal Processing (ICASSP)(2022)
摘要
While deep neural networks achieve state-of-the-art performance on many audio classification tasks, they are known to be vulnerable to adversarial examples - artificially-generated perturbations of natural instances that cause a network to make incorrect predictions. In this work we demonstrate a novel audio-domain adversarial attack that modifies benign audio using an interpretable and differentiable parametric transformation - adaptive filtering. Unlike existing state-of-the-art attacks, our proposed method does not require a complex optimization procedure or generative model, relying only on a simple variant of gradient descent to tune filter parameters. We demonstrate the effectiveness of our method by performing over-the-air attacks against a state-of-the-art speaker verification model and show that our attack is less conspicuous than an existing state-of-the-art attack while matching its effectiveness. Our results demonstrate the potential of transformations beyond direct waveform addition for concealing high-magnitude adversarial perturbations. allowing adversaries to attack more effectively in challenging, real-world settings.
更多查看译文
关键词
Adversarial examples,speaker verification
AI 理解论文
溯源树
样例
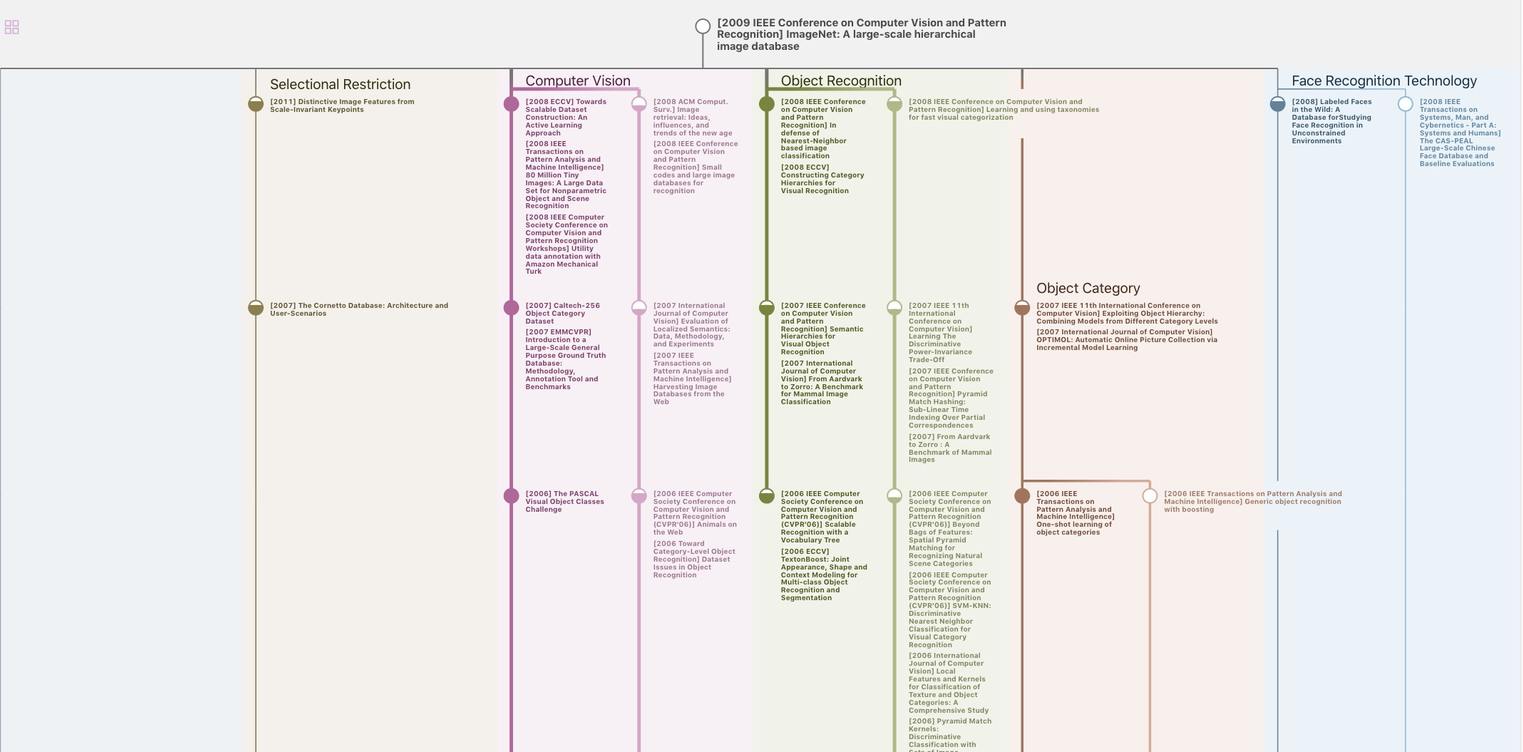
生成溯源树,研究论文发展脉络
Chat Paper
正在生成论文摘要