Increasing Driver Flexibility through Personalized Menus and Incentives in Ridesharing and Crowdsourced Delivery Platforms
semanticscholar(2021)
摘要
Allowing drivers to choose which requests to fulfill provides drivers with much-needed autonomy in ridesharing and crowdsourced delivery platforms. While stochastic, a driver’s acceptance of requests in their menu is influenced by the platform’s offered compensation. Therefore, in this work, we create and solve an optimization model to determine personalized menus and incentives to offer drivers. We exploit variable properties to circumvent nonlinear variable relationships, formulating the model as a linear integer program. Stochastic driver responses are modeled as a sample of variable and fixed scenarios. An imposed premium counterbalances solution overfitting. Solution methods decompose and iterate, improving performance of computational experiments that use request/driver trip information from the Chicago Regional Transportation Network. Our approach outperforms alternative methods and a fixed incentives menu-only model by strategically using personalized incentives to prioritize promising matches and increase drivers’ willingness to accept requests. This benefits both customers and drivers: the average driver income is increased by 4.1% compared to the menu-only model, and 96.6% of requests are matched (4.1% higher than the menu-only method). Higher incentives are offered when drivers are more likely to accept, while fewer incentives and menu slots are reserved for driver-request pairs less likely to be accepted.
更多查看译文
AI 理解论文
溯源树
样例
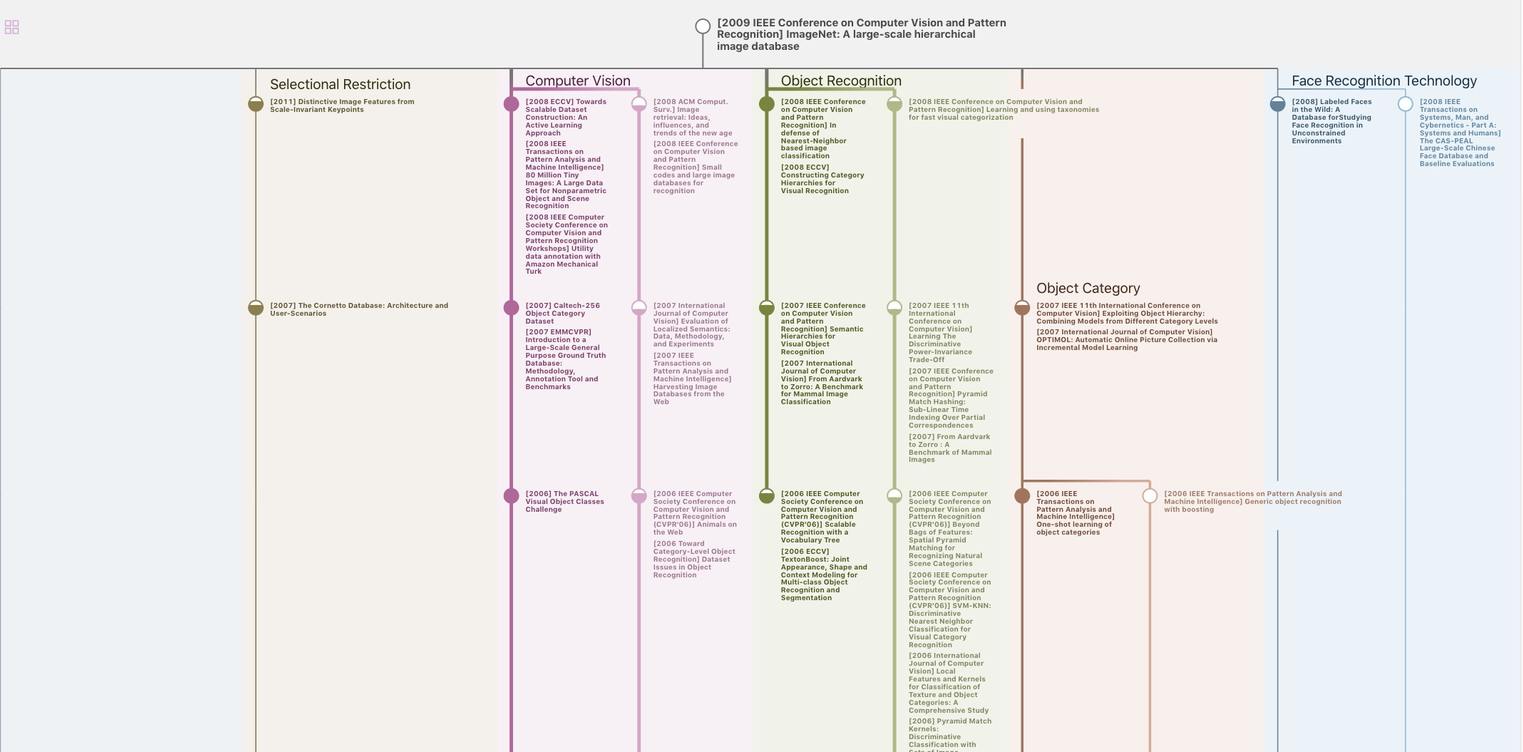
生成溯源树,研究论文发展脉络
Chat Paper
正在生成论文摘要