TRIGGER: TempoRal Interaction Graph GenEratoR
semanticscholar(2021)
摘要
Efforts on temporal graph generation have focused on generating instances from the same steady state (e.g., keeping a fixed-size window over a sequence of edges generated from the same model). Unfortunately, such generators cannot capture the underlying information richness of the temporal aspects of activity graphs. Based on the underlying phenomena being represented by the graph, temporal properties of interest will vary. In addition to topological features, such as neighbor information, we are interested in frequencies of communication. Subsequently, our work can be split into two natural steps: building models that can represent the temporal characteristics of nodes of a graph and generating temporal activity graphs that display the features of our model. We present TRIGGER (TempoRal Interaction Graph GenEratoR): A Markov Model-based activity generation approach that classifies the nodes into profiles and generates a series of repeating interactions in continuous time. Then, we show how to estimate an input model to represent a real world graph. We carried out extensive experiments to validate our approach using various real-world temporal datasets and metrics on the quality of generated graphs. We show that our approach can generate realistic temporal activity graphs and match temporal metrics such as burstiness, spread, and persistence and static metrics at both graph and the node scale.
更多查看译文
AI 理解论文
溯源树
样例
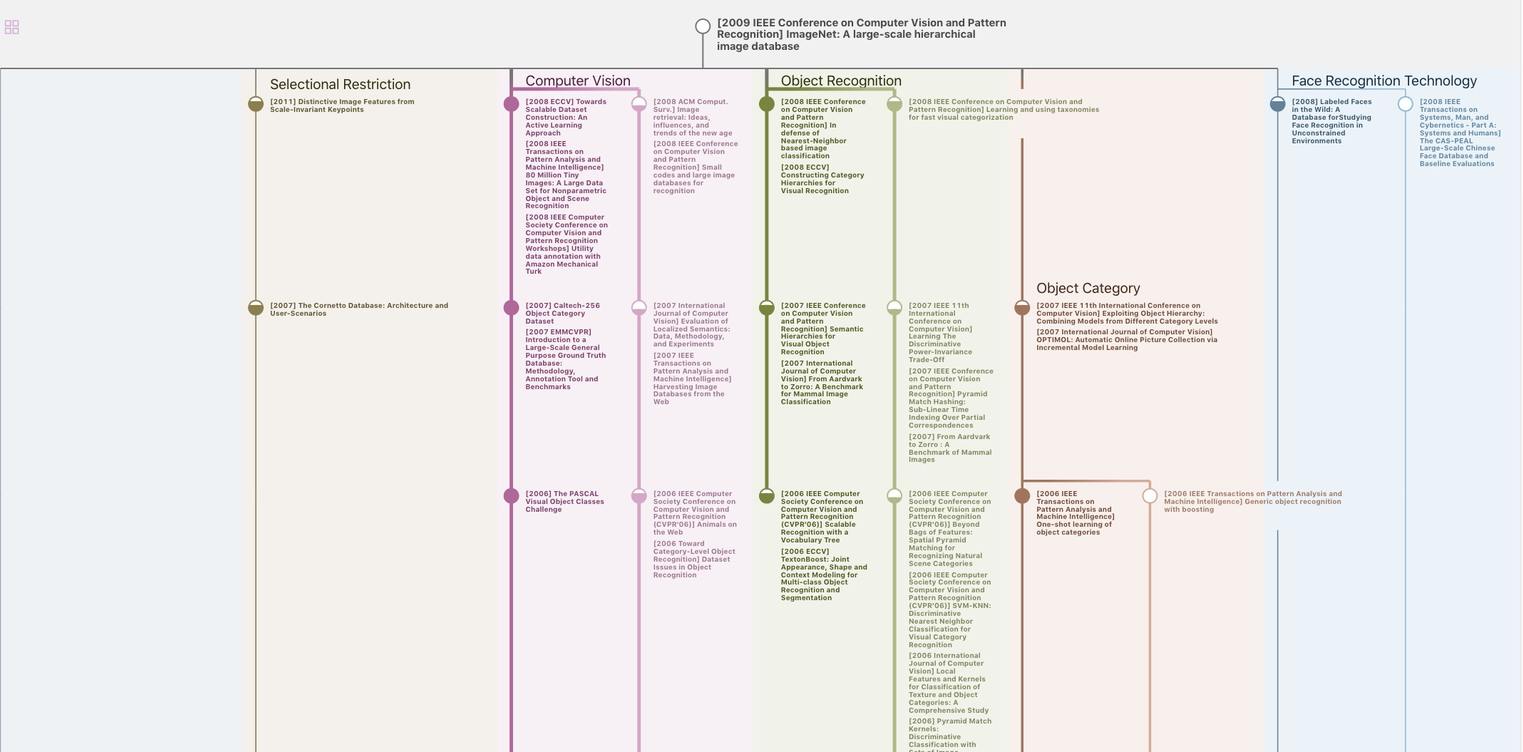
生成溯源树,研究论文发展脉络
Chat Paper
正在生成论文摘要