Smarter micro-targeting to improve global health outcomes: scaling cluster segmentation on novel types of data for precision public health
semanticscholar(2018)
摘要
In global health, optimized interventions that rely on voluntary uptake should account for decision-making heterogeneity within the target population. This is a major challenge: to efficiently use scarce resources, the right people should be targeted with the right intervention at the right time. This entails segmenting the population of interest based on the differences that underlie their decision-making, prioritizing which segments to target, and then developing segment-specific intervention strategies. Effective global health interventions at scale require large financial and human resource investments, and both are limited in resource-poor settings. Therefore, scaling segmentation approaches to optimize intervention development and deployment is a key challenge and requires collaboration at the intersection of global health and machine learning experts. Here, we demonstrate how the collection of novel types of data coupled with the application of unsupervised classification algorithms can help micro-target interventions on the ground to drive health outcomes. Highlighting a case study on Voluntary Medical Male Circumcision in Africa, we discuss lessons learned and innovations in future programs, especially how mobile approaches to data collection and analytics can be streamlined to make segmentation a scalable approach across global health.
更多查看译文
AI 理解论文
溯源树
样例
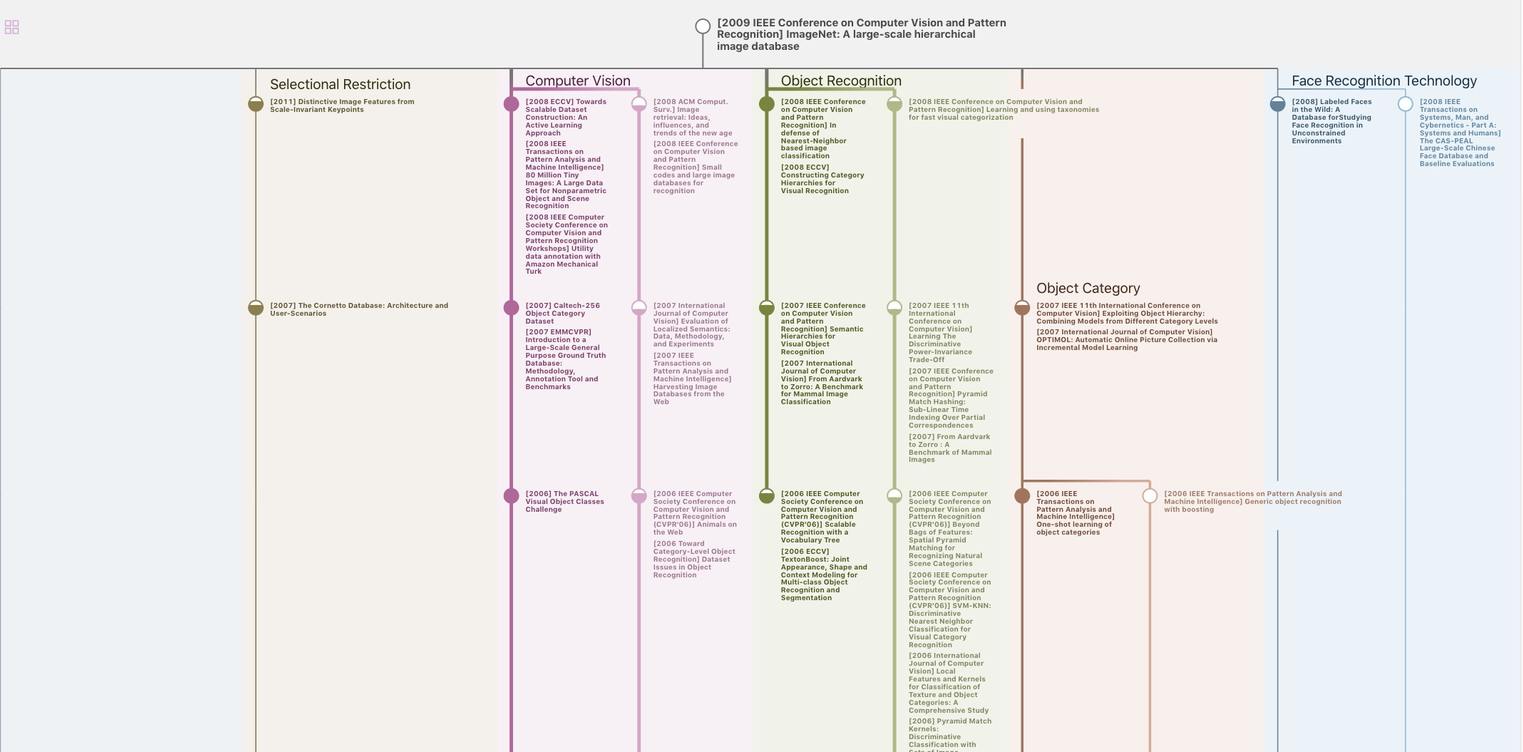
生成溯源树,研究论文发展脉络
Chat Paper
正在生成论文摘要