Title : Large field of view-spatially resolved transcriptomics at nanoscale resolution Short title : DNA nanoball stereo-sequencing
semanticscholar
摘要
High-throughput profiling of in situ gene expression represents a major advance towards the systematic understanding of tissue complexity. Applied with enough 3 capture area and high sample throughput it will help to define the spatio-temporal dynamics of gene expression in tissues and organisms. Yet, current technologies have considerable bottlenecks that limit widespread application. Here, we have combined DNA nanoball (DNB) patterned array chips and in situ RNA capture to develop Stereo-seq (Spatio-Temporal Enhanced REsolution Omics-sequencing). This approach allows high sample throughput transcriptomic profiling of histological sections at unprecedented (nanoscale) resolution with areas expandable to centimeter scale, high sensitivity and homogenous capture rate. As proof of principle, we applied Stereo-seq to the adult mouse brain and sagittal sections of E11.5 and E16.5 mouse embryos. Thanks to its unique features and amenability to additional modifications, Stereo-seq can pave the way for the systematic spatially resolved-omics characterization of tissues and organisms. MAIN TEXT Single-cell RNA-sequencing (scRNA-seq) technologies have unveiled an unexpectedly large degree of heterogeneity in cell types and cell states among tissues (1-5). Because multicellular organization and cell-cell interactions determine individual cell function, spatially resolved transcriptomic information is necessary to fully understand tissue complexity in normal physiology or under perturbation. This is best illustrated in embryonic development, where the spatio-temporal coordination of cells and signaling cues determine the dynamics of self-organized patterning, but in fact applies to any tissue and biological process. For example, spatially resolved transcriptomic information of the complex interactions between cancer cells, immune cells and stromal cells in the tumor niche (6, 7) could be useful to improve anticancer therapies. Similarly, revealing the dynamic changes of gene expression responsible for the spreading pattern of certain neurodegenerative diseases (8, 9) could help prevent this process. A number of remarkable methodologies have been recently developed that enable the high-throughput transcriptomic profiling of in situ gene expression. These techniques can be grouped into three main categories depending on the RNA 4 capture approach: a) physical segmentation using laser microdissection, b) pre-designed targeted probes (for either single-molecule hybridization or amplification and in situ decoding) or c) DNA-barcoded probes. Examples of the former approach include LCM-seq (laser capture microscopy coupled with full-length mRNA-sequencing) (10) and GEO-seq (geographical position sequencing) (11), which are highly operable but have low capture throughput, limited resolution (center-to-center distance of each array component) and the accuracy of spatial information is subjected to experimental variability. Examples of techniques based on pre-designed probes are MERFISH (multiplexed error-robust FISH) (12) and seqFISH (sequential fluorescence in situ hybridization) (13) for hybridization-based methods, and FISSEQ (fluorescent in situ RNA sequencing) (14) and STARmap (spatially-resolved transcript amplicon readout mapping) (15) for in situ decoding. General caveats of these techniques are that they rely on a finite number of sequences, can only be practically applied to a small field of view and require complex instrumentation. As for the latter group, Slide-seq (16), spatial transcriptomics (ST, Visium) (17) and their optimized versions Slide-seqV2 and HDST (high-definition spatial transcriptome) (18, 19), respectively, use barcoded arrays and have attracted significant attention. Similarly, DBiT-seq (deterministic barcoding in tissue for spatial omics sequencing) uses microfluidic channels for delivering barcoded probes directly into tissues and can simultaneously capture transcripts and selected protein targets (20). Although unbiased in the nature of captured transcripts, barcoded probe-based techniques suffer from an inadequate balance between resolution, average numbers of gene/transcript per bin and capture rate across multiple bins. Besides, as for hybridization-based methods, the measured areas are small (e.g., Visium 42.5 mm2, Slide-seq/Slide-seqV2 7 mm2, HDST 13.68 mm2 and DBiT-seq 25 mm2) (17-20) and face a major bottleneck of scalability. Increasing the resolution is important because it can help define transcriptomic gradients within tissues more accurately and allow detection of the subcellular localization of transcripts. This could also reduce problems associated with RNA diffusion, capture rate or disturbed patterns caused by unmeasured inter-bin space. Likewise, enlarging the capture area could allow the 5 measurement of whole mammalian embryos, human organs, or model organisms or the simultaneous assessment of multiple smaller samples (e.g., biopsies) on the same chip, avoiding the batch effect problem caused by separate runs and facilitating automation. Our aim was to develop a spatially resolved transcriptomic technology that combines billions of tightly packed barcodes in known positions with large-captured areas, high capture rate and high reproducibility. We deposited DNA nanoball (DNB) containing random barcoded sequences onto a modified silicon surface (chip) photolithographically etched with a grid-patterned array of spots where the DNB are efficiently docked in (21), each spot being approximately 220 nm in diameter and with a center-to-center distance of 500 or 715 nm (Fig. 1, step 1). The use of random barcode-labeled DNB generated by rolling circle amplification allows a much larger spatial barcode pool size (425) compared with bead-based approaches and at the same time maintains sequence fidelity. The array was then microphotographed, incubated with primers and sequenced to obtain the data matrix containing the coordinate identity (CID) of every etched DNB (Fig. 1, step 2). Then, molecular identifiers (MID) and polyT sequence-containing oligonucleotides were ligated on each spot through hybridization with the CID (Fig. 1, step 3). This DNB-based strategy enables the generation of large chips containing barcoded probes at a much higher density than any other previous method. The next step consisted in the capture of tissue polyA-tailed RNA, which was achieved in situ by loading fresh-nitrogen frozen tissue sections onto the chip surface, followed by fixation, permeabilization and finally reverse transcription and amplification (Fig. 1, step 4). Amplified cDNAs were collected, used as template for library preparation and sequenced together with the CID (Fig. 1, step 5). Computational analysis of the sequencing data allows spatially resolved transcriptomics with a resolution of 500 or 715 nm and minimal inter-bin space (Fig. 1, step 6), resulting in a significantly higher number of spots per 100 μm2 (average size of a mammalian single cell) than any other available method (Fig. 2a). Currently, we have used Stereo-seq chips of 50, 100 and 200 mm2 in tissues like the mouse olfactory bulb (~11.7 mm2), half mouse brain (~26.7 mm2) or a whole E11.5 6 (~10.5 mm2) and E16.5 (~76.9 mm2) mouse embryos (fig. S1a). Notably, we have also developed DNB patterned array chips of up to 42.25 cm2 for potential application to large tissues such as whole human brain tissue sections (fig. S1a, b). We termed our approach Stereo-seq (Spatio-Temporal Enhanced REsolution Omics-sequencing), We first profiled the mouse olfactory bulb with Stereo-seq because it is a widely used model tissue for spatially resolved transcriptomic approaches (18, 19). We used patterned Stereo-seq chips (715 nm resolution) in combination with DAPI staining, which confirmed that the spatial transcriptomic information reflects the cellular structure of the tissue (Fig. 2b). Stereo-seq captured numbers ranging on average from 9,342 genes and 34,435 transcripts per 143 m bin (bin 200, 200 × 200 DNB) to 275 genes and 346 transcripts per 14 m bin (bin 20, 20 × 20 DNB, equivalent to ~1 cell) (Fig. 2c). Our reanalysis of previously reported datasets showed that Visium captures an average of 5,735 genes and 25,598 transcripts at 100 m resolution (mouse brain), Slide-seq 37 genes and 45 transcripts at 10 m resolution (hippocampus) (16), Slide-seqV2 426 genes and 494 transcripts at 10 m resolution (hippocampus) (18), and DBiT-seq 3,301 genes and 7,603 transcripts at 100 m resolution (E10 mouse embryo) and 1,801 genes and 3,572 transcripts at 20 m resolution (E10 mouse embryo eye/brain) (Fig. 2c). Gene and transcript capture of Stereo-seq per 2 m-diameter bin (bin 3, 3 × 3 DNB, subcellular resolution) was substantially higher than HDST (mouse olfactory bulb; 2 genes and 2 transcripts at 2 m resolution) (19) (Fig. 2d), which is so far the only sequencing-based spatially resolved transcriptomic technology with subcellular resolution. We then performed unsupervised clustering to computationally reconstruct the spatial identity of the olfactory bulb profiled with Stereo-seq. We observed clustering consistent with known anatomical structures including the outer plexiform layer (OPL), granular cell zone deep (GCL-D), granular cell layer internal (GCL-I), internal plexiform layer (IPL), subependymal zone (SEZ), olfactory nerve layer (ONL), glomerular layer (GL) and granular cell layer externa (GCL-E) (Fig. 2e). This also identified two distinct sublayers within the GL and five in the ONL, suggesting potential sub-regional differences. The consistency between biological replicates using two adjacent sections 7 of the mouse olfactory bulb profiled with Stereo-seq was high (fig. S1c-f). In addition, there was also high correlation between similar anatomical areas in each of the olfactory bulb halves from a single section (fig. S2a). Analysis of reported mouse olfactory bulb datasets obtained with HDST or Slide-seqV2 did not reflect tissue organi
更多查看译文
AI 理解论文
溯源树
样例
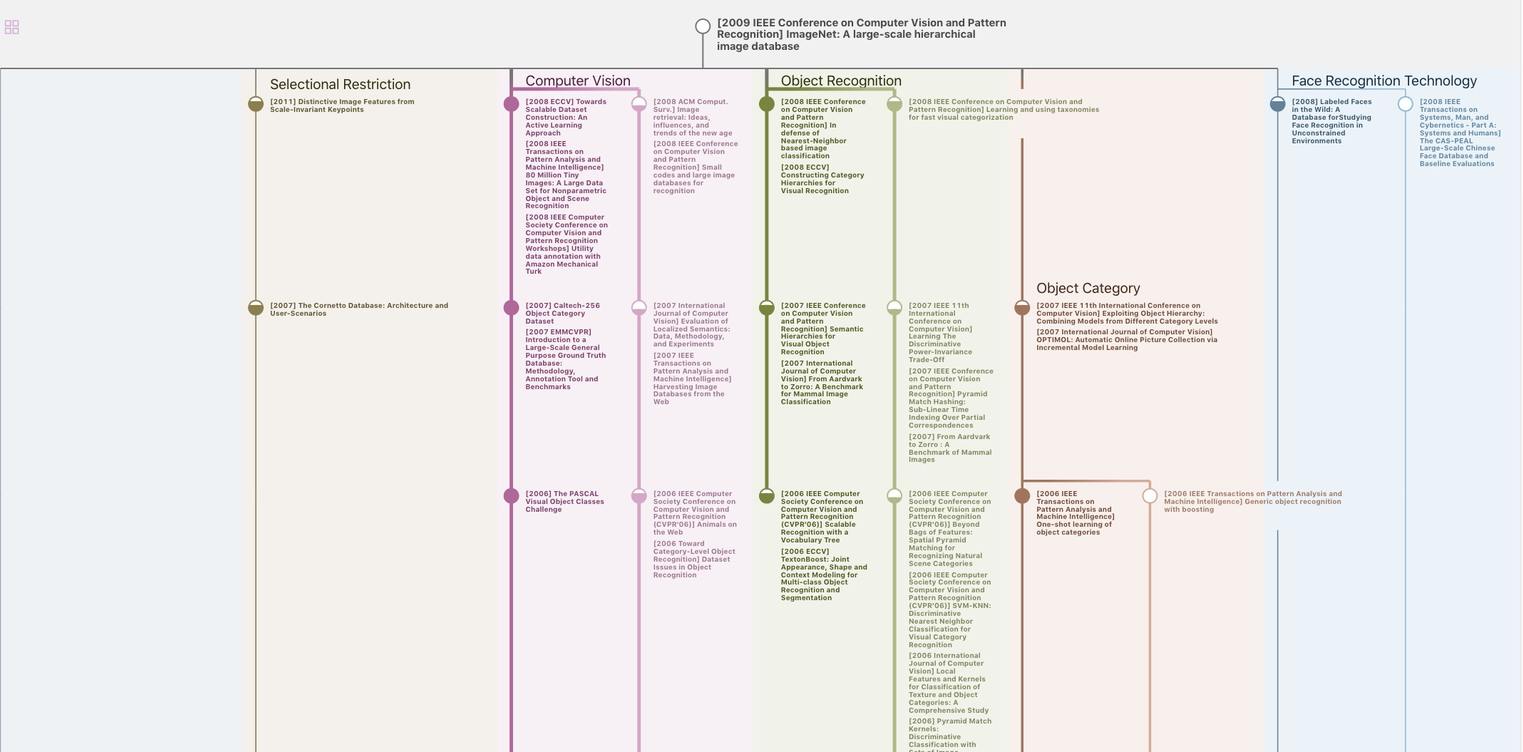
生成溯源树,研究论文发展脉络
Chat Paper
正在生成论文摘要