Estimation and inference of signals via the stochastic geometry of spectrogram level sets
ArXiv(2021)
摘要
Spectrograms are fundamental tools in the detection, estimation and analysis of signals in the time-frequency analysis paradigm. The spectrogram of a signal (usually corrupted with noise) is the squared magnitude of its short time Fourier transform (STFT), which in turn is a generalised version of the classical Fourier transform, augmented with a window in the time domain. Signal analysis via spectrograms have traditionally explored their peaks, i.e. their maxima, complemented by a recent interest in their zeros or minima. In particular, recent investigations have demonstrated connections between Gabor spectrograms of Gaussian white noise and Gaussian analytic functions (abbrv. GAFs) in different geometries. However, the zero sets (or the maxima or minima) of GAFs have a complicated stochastic structure, which makes a direct theoretical analysis of usual spectrogram based techniques via GAFs a difficult proposition. These techniques, in turn, largely rely on statistical observables from the analysis of spatial data, whose distributional properties for spectrogram extrema are mostly understood empirically. In this work, we investigate spectrogram analysis via an examination of the stochastic, geometric and analytical properties of their level sets. This includes a comparative analysis of relevant spectrogram structures, with vs without the presence of signals coupled with Gaussian white noise. We obtain theorems demonstrating the efficacy of a spectrogram level sets based approach to the detection and estimation of signals, framed in a concrete inferential set-up. Exploiting ∗Dept. of Mathematics, National University of Singapore, subhrowork@gmail.com †Dept. of Mathematics, National University of Singapore, lin meixia@u.nus.edu ‡Dept. of Mathematics, National University of Singapore, sun dongfang@u.nus.edu 1 ar X iv :2 10 5. 02 47 1v 1 [ ee ss .S P] 6 M ay 2 02 1 these ideas as theoretical underpinnings, we propose a level sets based algorithm for signal analysis that is intrinsic to given spectrogram data. We substantiate the effectiveness of the algorithm by extensive empirical studies, and provide additional theoretical analysis to elucidate some of its key features. Our results also have theoretical implications for spectrogram zero based approaches to signal analysis.
更多查看译文
关键词
spectrogram level sets,stochastic geometry,estimation,signals
AI 理解论文
溯源树
样例
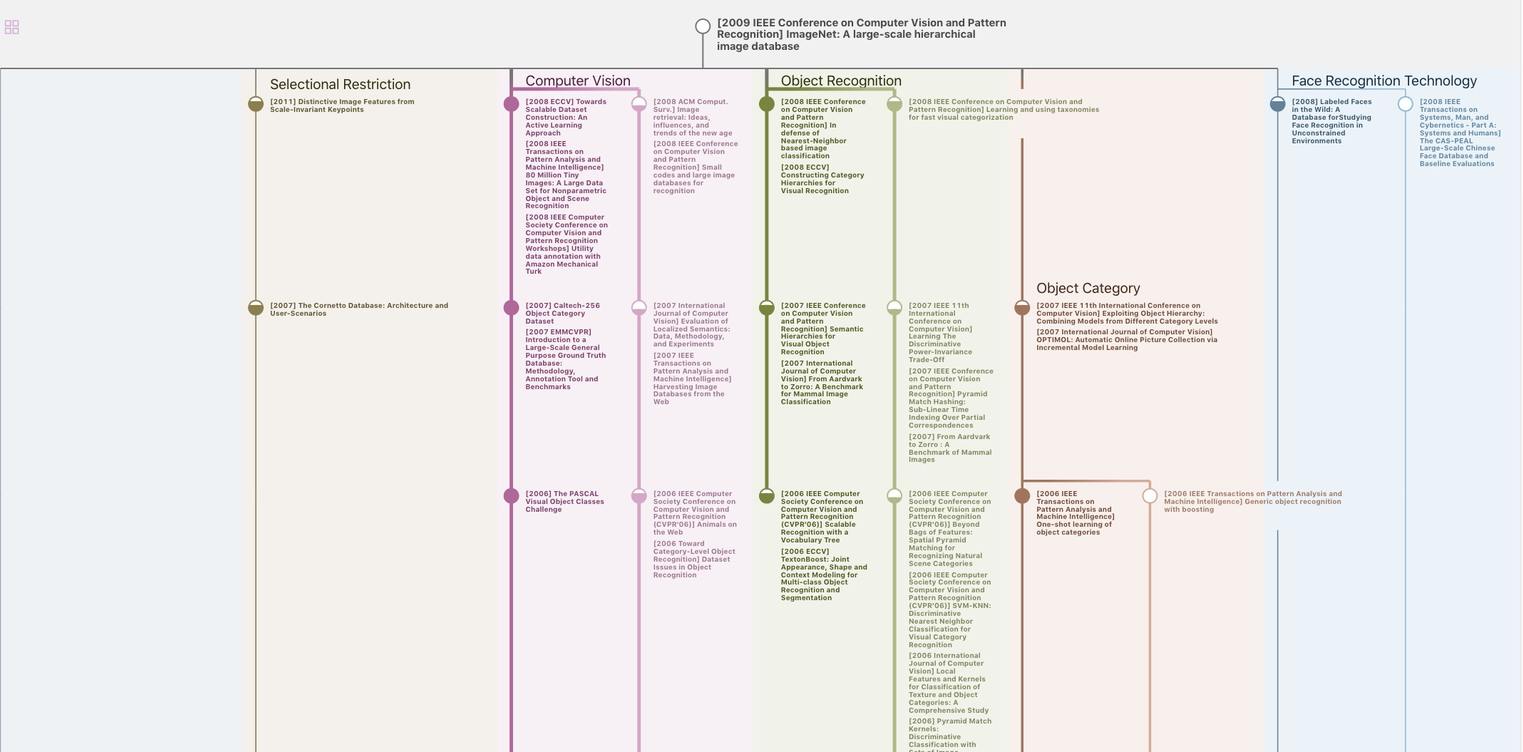
生成溯源树,研究论文发展脉络
Chat Paper
正在生成论文摘要