Regionalized LCI modeling: The Case of Regionalized Cotton Datasets
semanticscholar(2017)
摘要
Companies in the fashion industry are increasingly looking for reliable data to make informed decisions and to prioritize their sustainability efforts. Life Cycle Assessment (LCA) provide a comprehensive and holistic way to assess environmental impacts over the full life cycle; yet, credible LCA data on the cultivation and processing of textiles is still limited. The World Apparel and Footwear Life Cycle Assessment Database (WALDB) was founded to solve this data challenge and to deliver robust data for environmental impact assessment and footprinting. One key limitation concerns LCA data on cotton cultivation. To date, the entire cultivation of cotton is represented in the form of two data points, i.e., country-generic LCA datasets which uniformly describe cotton cultivation in the context of the USA and in China. In other words, there is a lack in the coverage and geographical representativeness of LCA data representing global cotton cultivation. We believe that the integration of spatial data into LCA calculations can deliver more representative data for the assessment of cotton cultivation, when combined with a computerized method for regionalized LCI modeling. Regionalized LCI modeling is the procedure that generates and links process datasets to the location where they occur (Mutel et al. 2012). Spatial explicit data on various context conditions (precipitation, soil properties, etc.) and production parameters (crop-specific fertilizer input, yield, etc.) is now available, in decent resolution and on a global scale (Hengl et al. 2014; Monfreda et al. 2008; Mueller et al. 2012). However, the consideration of such data in the generation of LCA datasets is too labor-intensive with the classical means of data processing. Agricultural LCA datasets are mainly generated manually, according to specific guidelines and emissions models and involving a wide array of raw data sources, ranging from public available databases (FAOSTAT, EUROSTAT, etc.), company data, surveys, case studies, publications, measurements, etc. (Nemecek et al. 2015). They typically are sitegeneric meaning that one datasets represent an entire country. Reinhard et al. (2017) have developed a regionalization framework that is capable of processing the spatial explicit data on various context conditions and production parameters into comprehensive LCA datasets. This work-in-progress article examines the extensions of the framework for the generation of robust and geographically representative cotton datasets.
更多查看译文
AI 理解论文
溯源树
样例
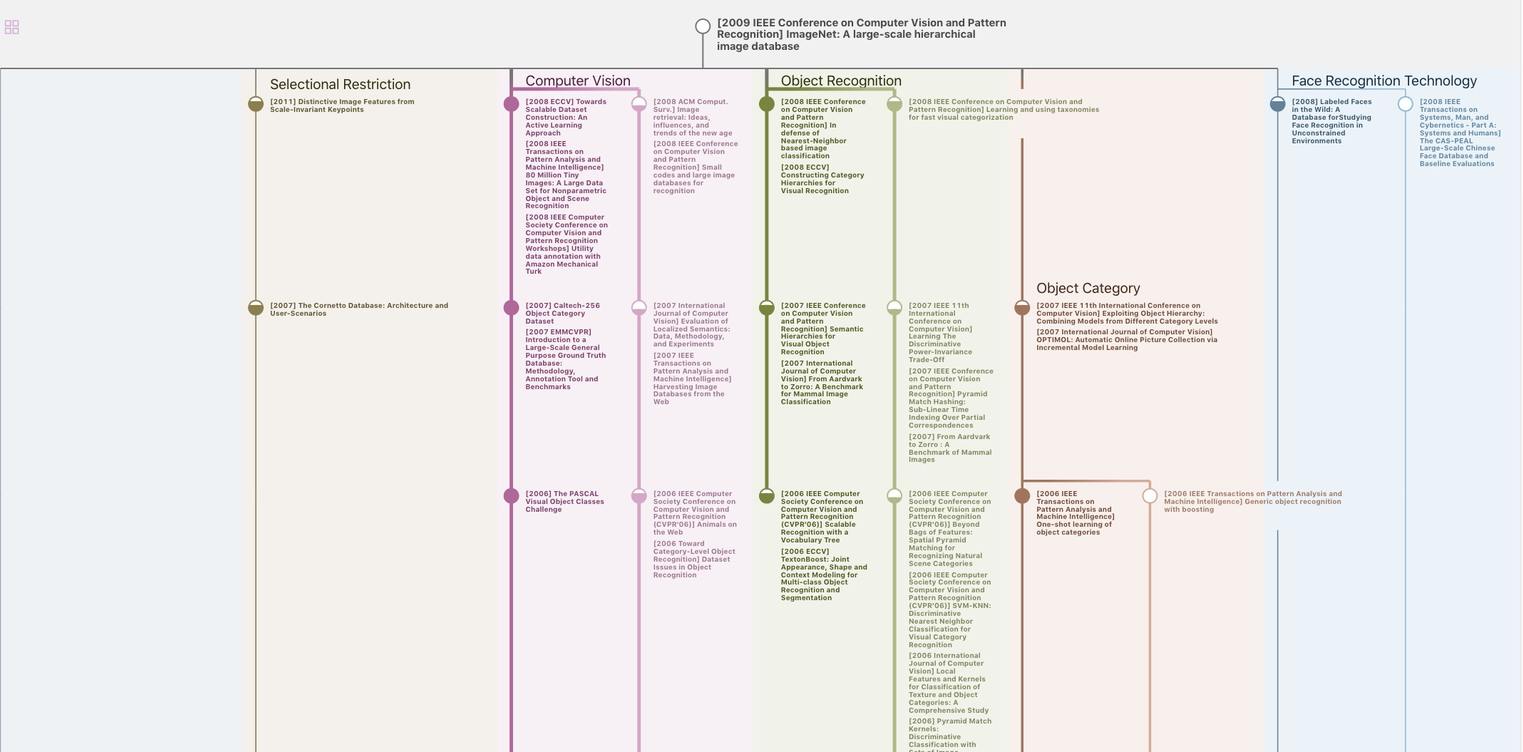
生成溯源树,研究论文发展脉络
Chat Paper
正在生成论文摘要