Feature-based data assimilation in geophysics – response to reviewers
semanticscholar(2018)
摘要
Many applications in science require that computational models and data be combined. In a Bayesian framework, this is usually done by defining likelihoods based on the mismatch of model outputs and data. However, matching model outputs and data in this way can be unnecessary or impossible. For example, using large amounts of steady state data is unnecessary because these data are redundant, it : . :: It is numerically difficult to assimilate data in chaotic systems, and it . :: It is often impossible to assimilate data of a complex system into a low-dimensional model. These ::: As : a ::::::: specific ::::::: example, :::::::: consider 5 : a :::::::::::::: low-dimensional :::::::: stochastic :::::: model ::: for ::: the :::::: dipole :: of ::: the :::::: Earth’s :::::::: magnetic ::::: field, ::::: while :::: other ::::: field :::::::::: components ::: are ::::::: ignored :: in :: the :::::: model. :::: The ::::: above : issues can be addressed by selecting features of the data, and defining likelihoods based on the features, rather than by the usual mismatch of model output and data. Our goal is to contribute to a fundamental understanding of such a feature-based approach that allows us to assimilate selected aspects of data into models. Specifically, we ::: We ::: also : explain how the feature-based approach can be interpreted as a method for reducing an effective dimension , and derive new noise models, 10 based on perturbed observations, that lead to computationally efficient solutions. Numerical implementations of our ideas are illustrated in four examples.
更多查看译文
AI 理解论文
溯源树
样例
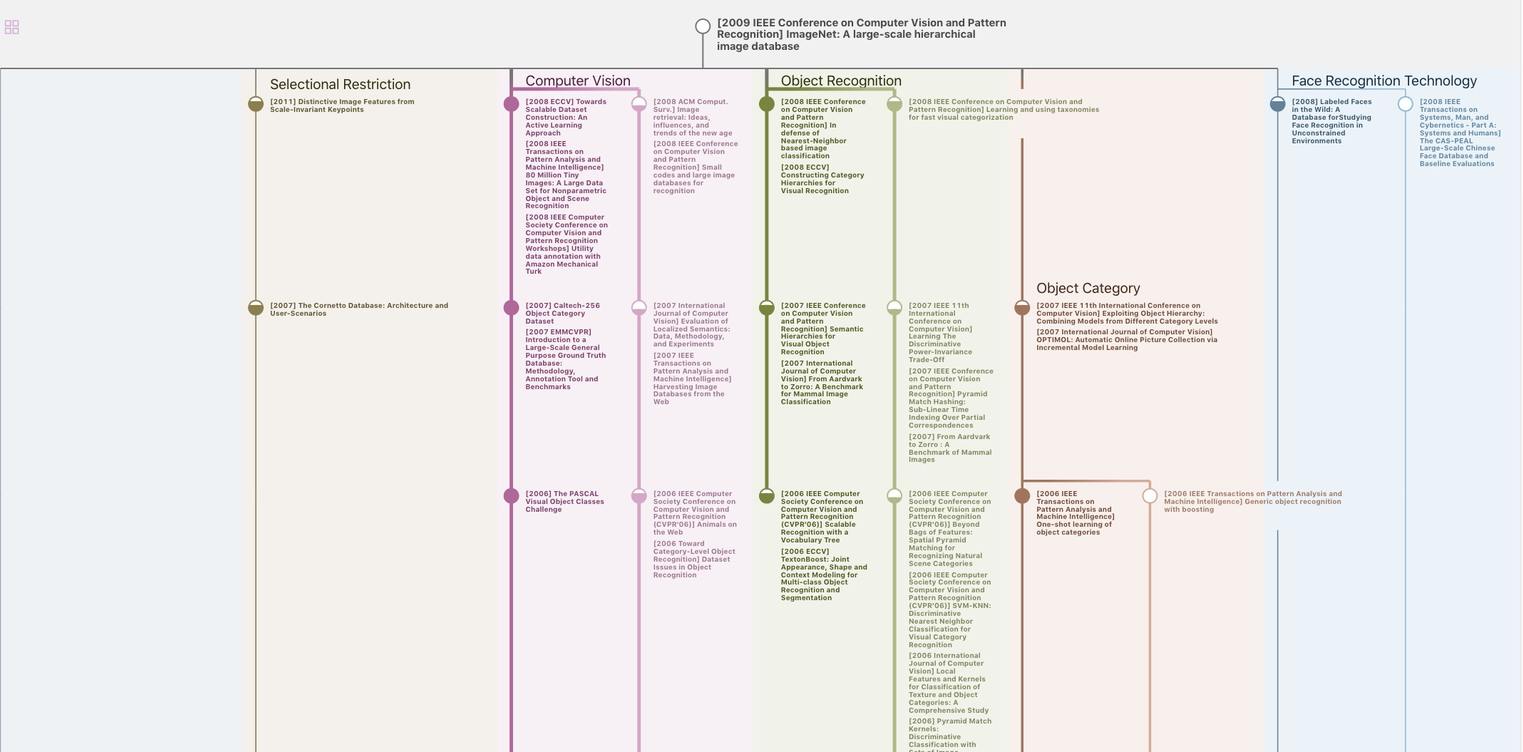
生成溯源树,研究论文发展脉络
Chat Paper
正在生成论文摘要