Tunable Quantum Wavelet Transform and Improved PSO Based Novel Evolutionary Extreme Learning Machine for Epilepsy Detection
semanticscholar(2018)
摘要
In our present work, EEG signals of various classes are broken down in Tunable Quantum Wavelet Transform (TQWT) system. The TQWT decays the EEG signals into sub-groups and arranged them into different descending order of frequencies. The nonlinearity of the EEG signals is evaluated by processing from the acquired features, which is additionally utilized as an element for ordering the typical classes of EEG signals. In this work, EEG signals are arranged in a solitary Random forest (RF) classification issues. In the first place classification is done in seizure classes, and the other one is the typical, seizure free classes. Features got from the EEG signal of these classes are feeded to the contribution of arbitrary Random Forest (RF), classifier prepared with improved PSO (IPSO). For seizure free and seizure classes, we accomplished 99.5581% precision, 98.5261% of Sensitivity and 99.6169% of Specificity and 99.0674% of Gmean esteem with RF classifier and TQWT feature extractor. Keywords— EEG Signal, Epilepsy, TQWT, IPSO, Random Forest classifier.
更多查看译文
AI 理解论文
溯源树
样例
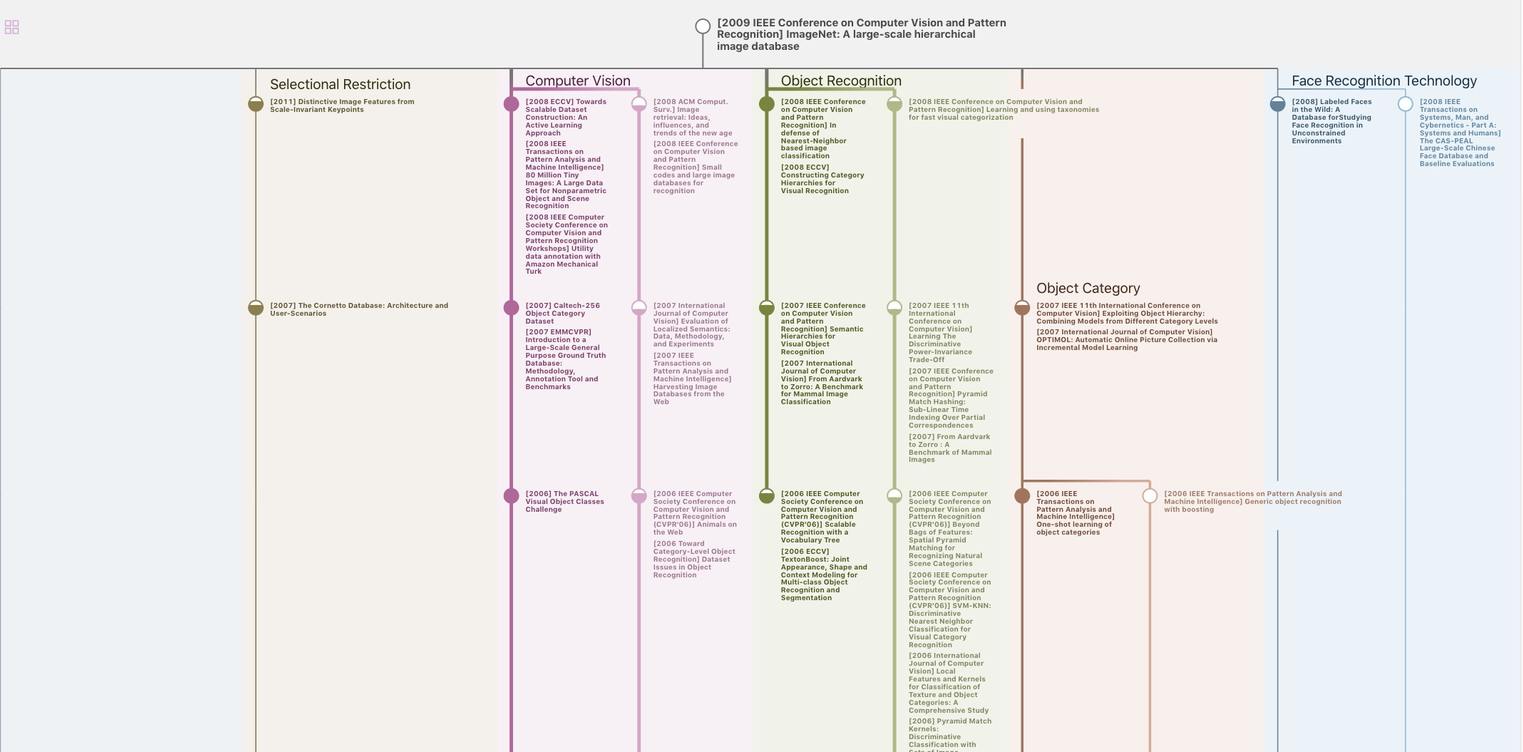
生成溯源树,研究论文发展脉络
Chat Paper
正在生成论文摘要